Clustering Of Spatial Cues By Semantic Segmentation For Anechoic Binaural Source Separation
APPLIED ACOUSTICS(2021)
摘要
The recent introduction of neural networks to speech separation has dramatically boosted the separation performance. This paper presents a novel psychoacoustic approach for speech source separation in anechoic conditions, using semantic segmentation of the interaural spectrograms of the audio mixtures. We have trained two separate U-Nets (a specialized neural network for semantic segmentation) on the interaural level difference (ILD) spectrogram, and the interaural phase difference (IPD) spectrogram of a single source. After training, these U-Nets are used to predict the class of each time frequency (TF) unit of the interaural spectrogram of the audio mixture. The ILD and IPD soft masks obtained from these U-Nets are combined by a novel scheme which utilizes the strength of the interaural cues in different frequency bands. The results show improved separation over two state of the art machine learning source separation systems utilizing the same interaural cues. There is average improvement of 7.32 dB in signal to distortion ratio (SDR) and 0.3 points improvement in short term objective intelligibility (STOI) over degenerate un-mixing estimation technique (DUET) algorithm and 2.51 dB improvement in SDR with comparable intelligibility over model-based expectation-maximization source separation and localization (MESSL) algorithm. (C) 2020 Elsevier Ltd. All rights reserved.
更多查看译文
关键词
Source separation, Anechoic, Binaural cues, Semantic segmentation, Neural networks, U-Net
AI 理解论文
溯源树
样例
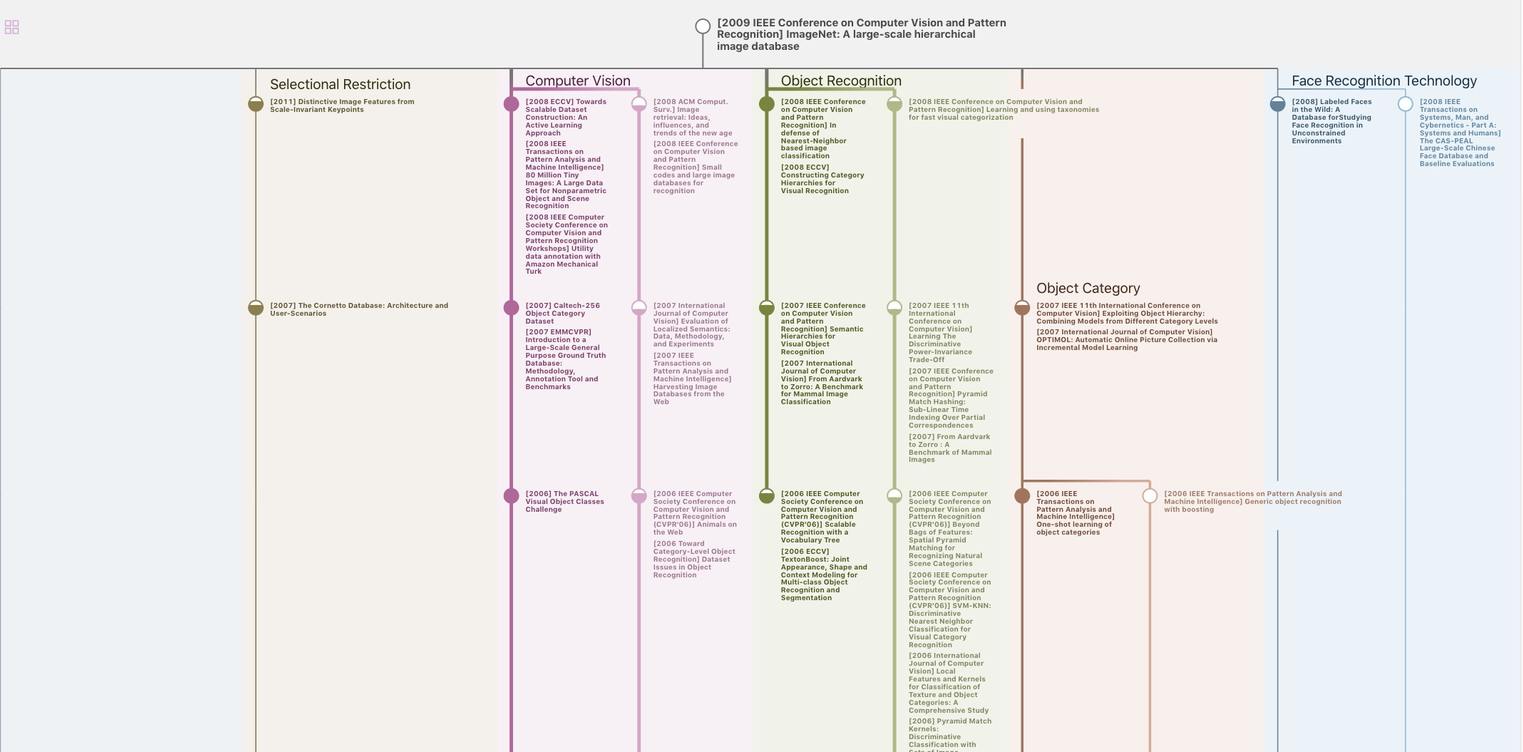
生成溯源树,研究论文发展脉络
Chat Paper
正在生成论文摘要