Investigating Self-supervised Pre-training for End-to-end Speech Translation
INTERSPEECH(2020)
摘要
Self-supervised learning from raw speech has been proven beneficial to improve automatic speech recognition (ASR). We investigate here its impact on end-to-end automatic speech translation (AST) performance. We use a contrastive predic-tive coding (CPC) model pre-trained from unlabeled speech as a feature extractor for a downstream AST task. We show that self-supervised pre-training is particularly efficient in low resource settings and that fine-tuning CPC models on the AST training data further improves performance. Even in higher resource settings, ensembling AST models trained with filter-bank and CPC representations leads to near state-of-the-art models without using any ASR pre-training. This might be particularly beneficial when one needs to develop a system that translates from speech in a language with poorly standardized orthography or even from speech in an unwritten language. Index Terms: self-supervised learning from speech, automatic speech translation, end-to-end models, low resource settings.
更多查看译文
关键词
self-supervised learning from speech, automatic speech translation, end-to-end models, low resource settings
AI 理解论文
溯源树
样例
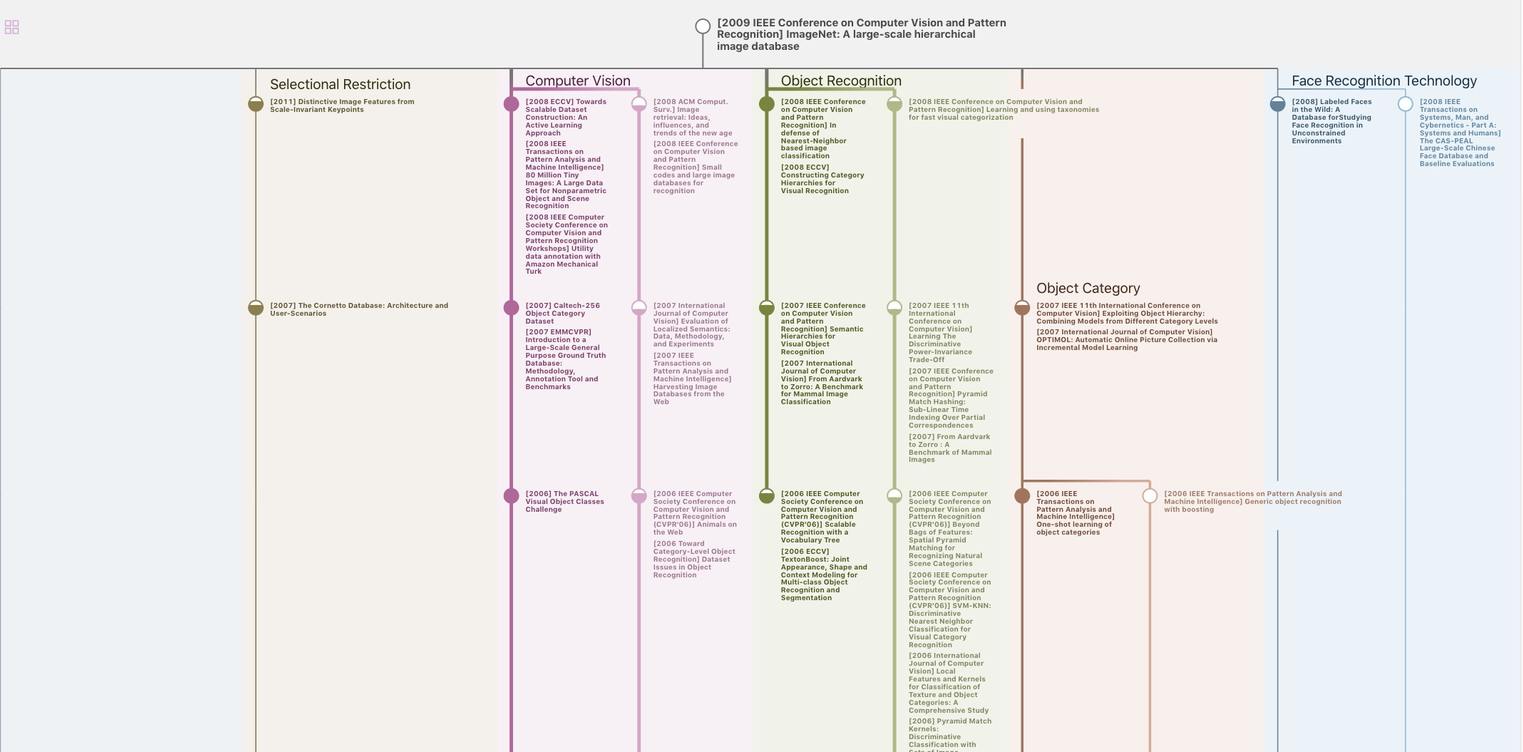
生成溯源树,研究论文发展脉络
Chat Paper
正在生成论文摘要