Uncertainty quantification for multi-scan registration
ACM Transactions on Graphics(2020)
摘要
AbstractA fundamental problem in scan-based 3D reconstruction is to align the depth scans under different camera poses into the same coordinate system. While there are abundant algorithms on aligning depth scans, few methods have focused on assessing the quality of a solution. This quality checking problem is vital, as we need to determine whether the current scans are sufficient or not and where to install additional scans to improve the reconstruction. On the other hand, this problem is fundamentally challenging because the underlying ground-truth is generally unavailable, and it is challenging to predict alignment errors such as global drifts manually. In this paper, we introduce a local uncertainty framework for geometric alignment algorithms. Our approach enjoys several appealing properties, such as it does not require re-sampling the input, no need for the underlying ground-truth, informative, and high computational efficiency. We apply this framework to two multi-scan alignment formulations, one minimizes geometric distances between pairs of scans, and another simultaneously aligns the input scans with a deforming model. The output of our approach can be seamlessly integrated with view selection, enabling uncertainty-aware view planning. Experimental results and user studies justify the effectiveness of our approach on both synthetic and real datasets.
更多查看译文
关键词
uncertainty quantification, multi-scan registration, approximation error, view planning
AI 理解论文
溯源树
样例
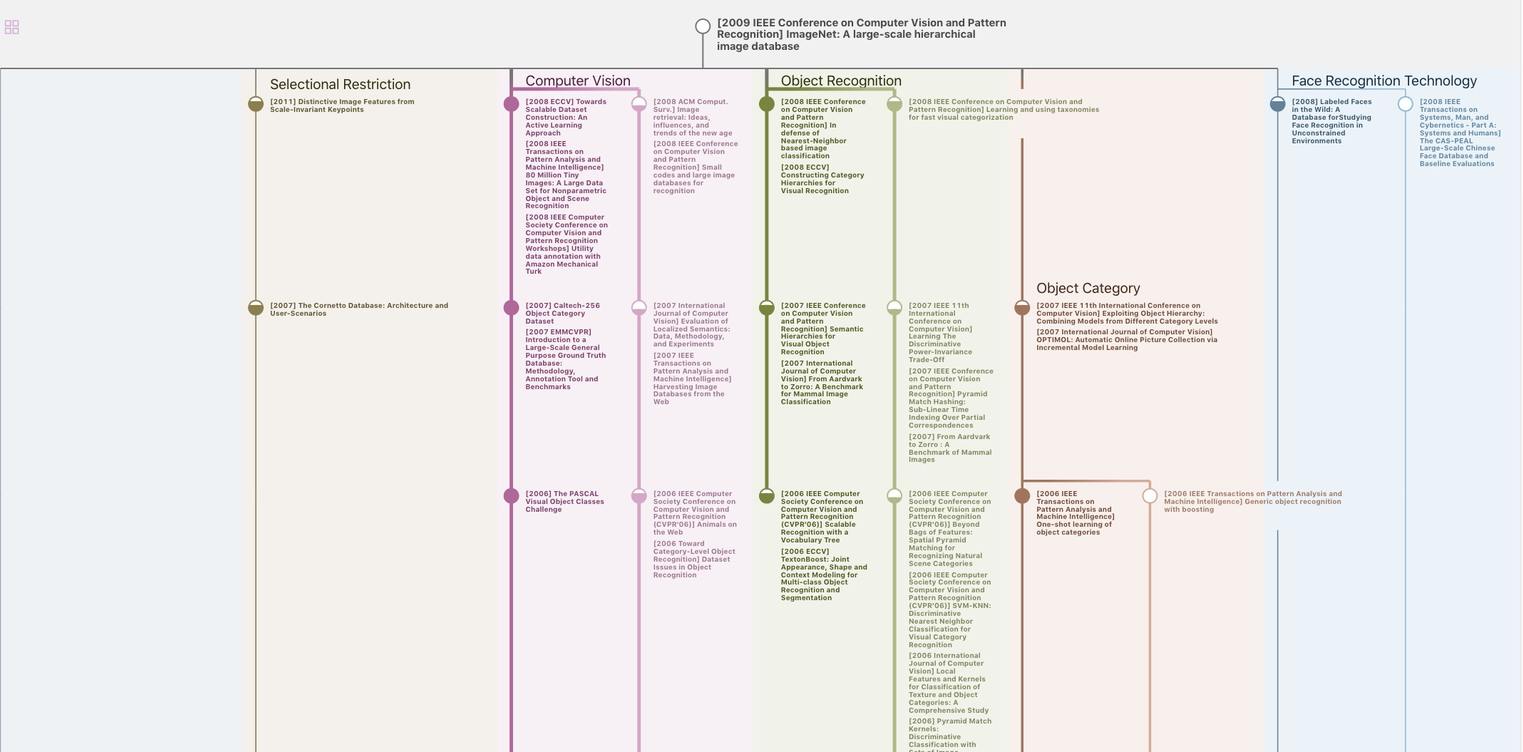
生成溯源树,研究论文发展脉络
Chat Paper
正在生成论文摘要