Limiting Privacy Breaches in Average-Distance Query
SECURITY AND COMMUNICATION NETWORKS(2020)
摘要
Querying average distances is useful for real-world applications such as business decision and medical diagnosis, as it can help a decision maker to better understand the users' data in a database. However, privacy has been an increasing concern. People are now suffering serious privacy leakage from various kinds of sources, especially service providers who provide insufficient protection on user's private data. In this paper, we discover a new type of attack in an average-distance query (AVGD query) with noisy results. The attack is general that it can be used to reveal private data of different dimensions. We theoretically analyze how different factors affect the accuracy of the attack and propose the privacy-preserving mechanism based on the analysis. We experiment on two real-life datasets to show the feasibility and severity of the attack. The results show that the severity of the attack is mainly influenced by the factors including the noise magnitude, the number of queries, and the number of users in each query. Also, we validate the correctness of our theoretical analysis by comparing with the experimental results and confirm the effectiveness of the privacy-preserving mechanism.
更多查看译文
AI 理解论文
溯源树
样例
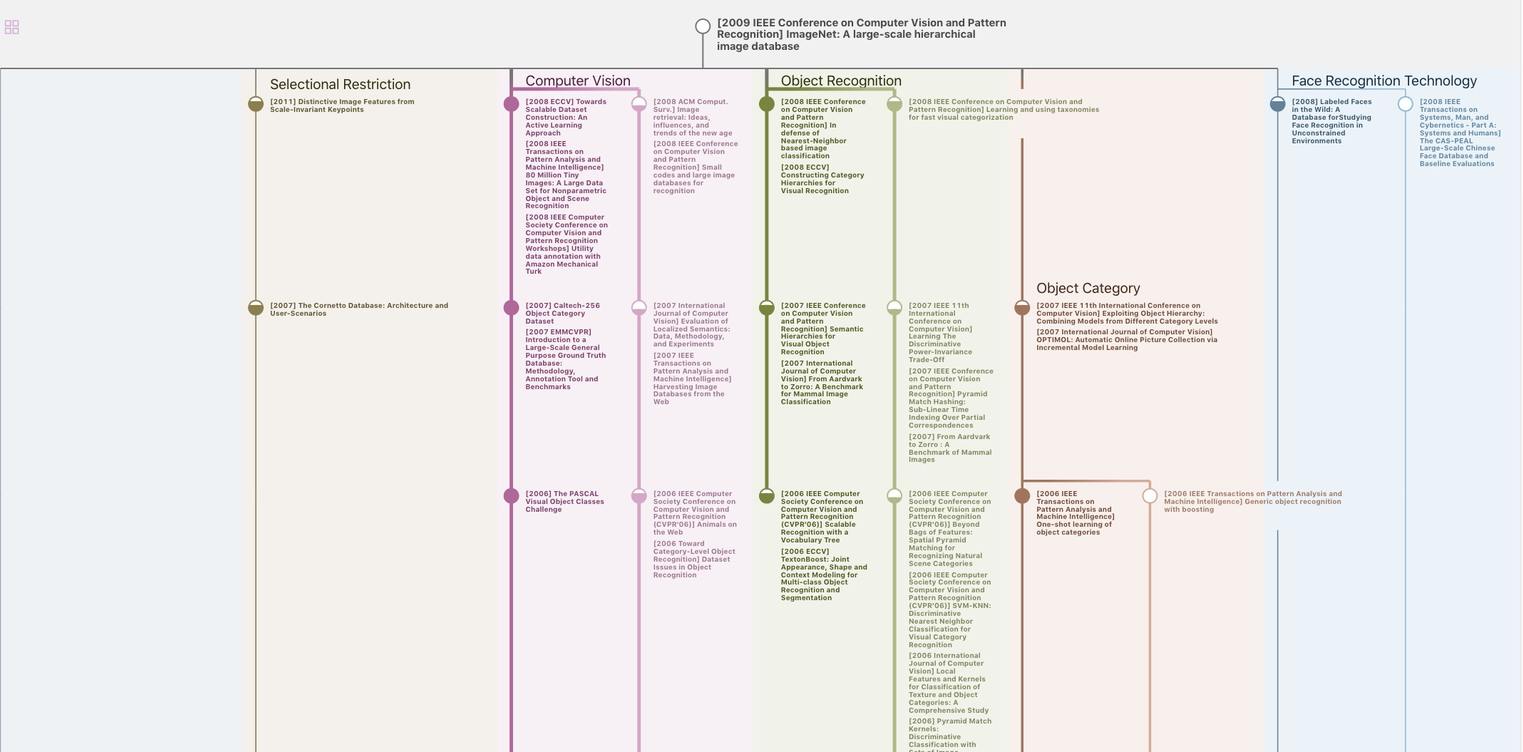
生成溯源树,研究论文发展脉络
Chat Paper
正在生成论文摘要