Discrete-Outcome Analysis Of Tornado Damage Following The 2011 Tuscaloosa, Alabama, Tornado
NATURAL HAZARDS REVIEW(2020)
摘要
Ground-truth measurements of damage caused by natural disasters are routinely used to determine which factors of the natural, built, and social environment contribute to losses from these events. Process-based models, which are based on structural design and probability theory, are often used in engineering risk analysis to predict damage and subsequent losses from these events, but empirical modeling can effectively aid in capturing the partial contribution of the factors of each of these interconnected environments leading to measured damage outcomes. An empirical assessment of tornado damage in a sample area of Tuscaloosa, Alabama, was conducted using satellite imagery collected at multiple time steps after the tornado and each building in the sample was assigned one of three damage outcomes: unaffected, damaged, or destroyed. Tornado event, building, and socioeconomic data were obtained for the sample area, and a deep learning approach based on satellite imagery was used to measure the amount of pretornado tree cover near each building in the study area. Multiple discrete-outcome modeling frameworks were tested to measure the partial effects of community characteristics leading to damage outcomes. Results show that combining publicly available imagery and deep learning approaches can produce more robust empirical models that would aid in identifying physically vulnerable locations within communities and produce more risk-informed decision-making. (c) 2020 American Society of Civil Engineers.
更多查看译文
关键词
Discrete-outcome analysis, Empirical modeling, Deep learning, Tornado vulnerability
AI 理解论文
溯源树
样例
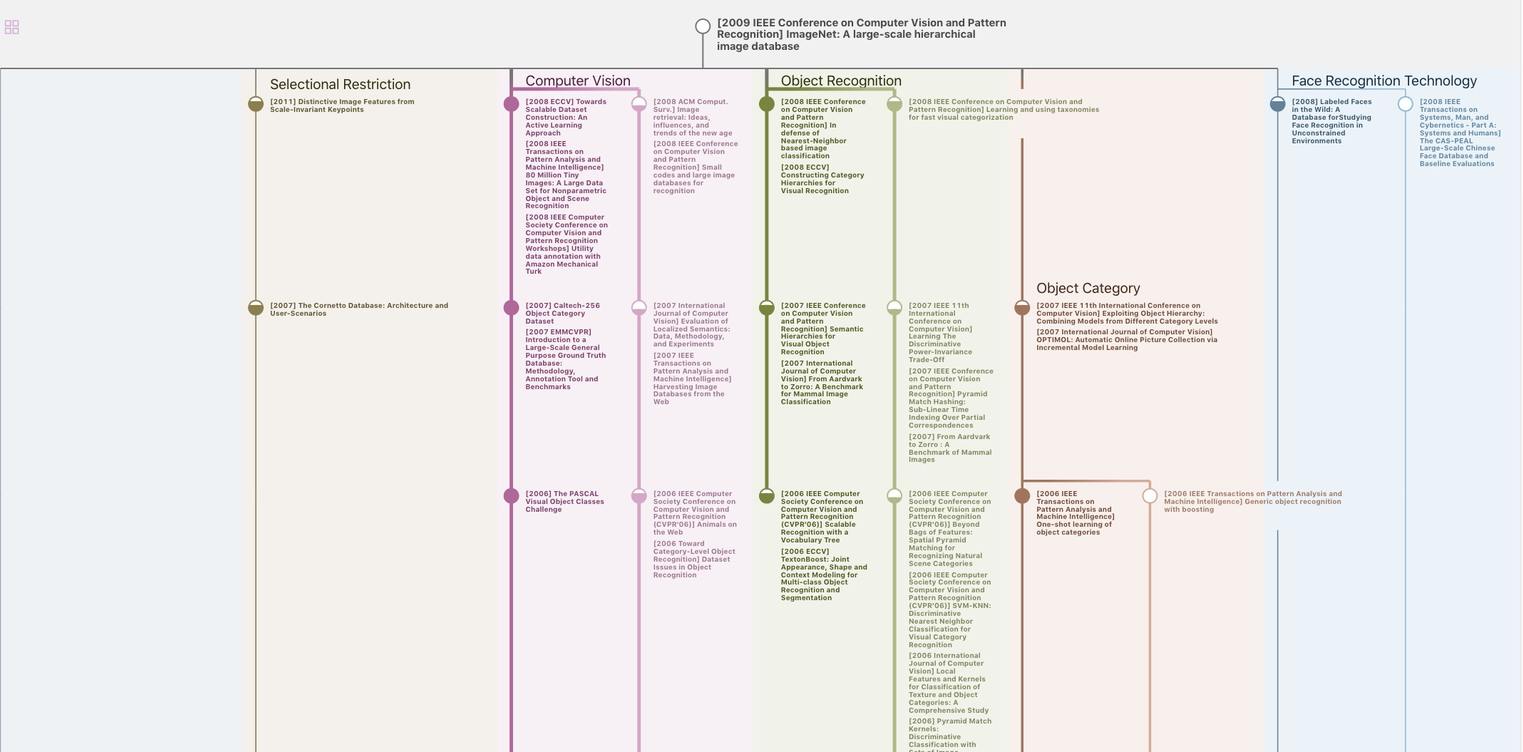
生成溯源树,研究论文发展脉络
Chat Paper
正在生成论文摘要