Identity-sensitive loss guided and instance feature boosted deep embedding for person search
Neurocomputing(2020)
摘要
Person search aims at detecting and re-identifying pedestrians from whole monitoring images, which is vital for intelligent surveillance. However, this task is still challenging due to the extremely few instances per training identity and inherent fine-grained differences among different identities. To this end, this work proposes an identity-sensitive loss guided and instance feature boosted pipeline to extract deep discriminative feature embedding for person search. First, a prior anchor pre-trained network (PAPN) is designed to obtain proper initial state for the whole deep person search training baseline. Second, a new loss function called instance enhancing loss (IEL) is proposed to learn identity-sensitive features by introducing unlabeled identity information. Specifically, the proposed IEL can selectively utilize unlabeled identities with similar appearances to labeled identities to train the person search network. Third, considering the intra-class compactness of features learned by center loss and contextual inter-class relations, two instance boosting strategies (Boosting) are used to learn more discriminative features. Extensive experiments on two benchmark datasets, namely CUHK-SYSU and PRW, demonstrate the effectiveness of our approach.
更多查看译文
关键词
Person search,Feature embedding,Loss function
AI 理解论文
溯源树
样例
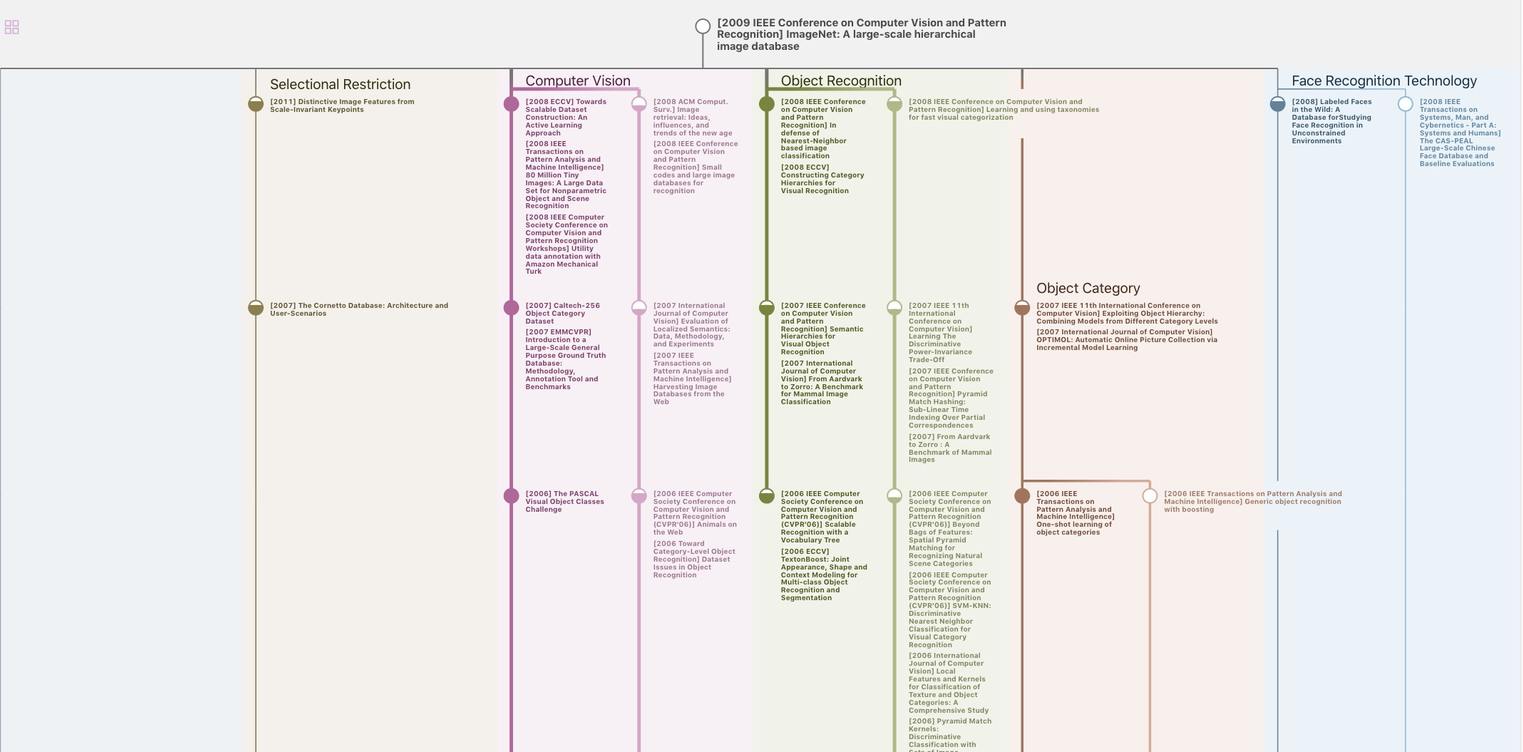
生成溯源树,研究论文发展脉络
Chat Paper
正在生成论文摘要