Semi-Supervised Subspace Learning for Pattern Classification via Robust Low Rank Constraint
MOBILE NETWORKS & APPLICATIONS(2020)
摘要
Feature subspace learning is a crucial issue in pattern analysis. However, it remains challenging when partial samples are unlabeled, which will cause weak discrimination. In this paper, we present a novel semi-supervised learning model that is capable of utilizing labeled and unlabeled training data simultaneously to learn discriminative feature subspace while preserving their locality. To achieve this goal, we joint learning feature subspace and completed labels. In the framework, low rank representation model is firstly exploited to explore the similarity relationship among all training samples, including labeled and unlabeled data. Then, the learned representation coefficients are used to generate a dynamic neighbor graph for designing the locality preservation constraints on both of label propagation and feature subspace. Finally, the prediction and true label are used to enforce the discrimination of feature subspace in the semantic space. Extensive experiment indicates that our proposed approach is more competitive than other comparison methods, while the model shows more robustness when training datasets are contaminated with noise.
更多查看译文
关键词
Subspace learning, Graph semi-supervised learning, Robust low-rank representation, Discriminative analysis
AI 理解论文
溯源树
样例
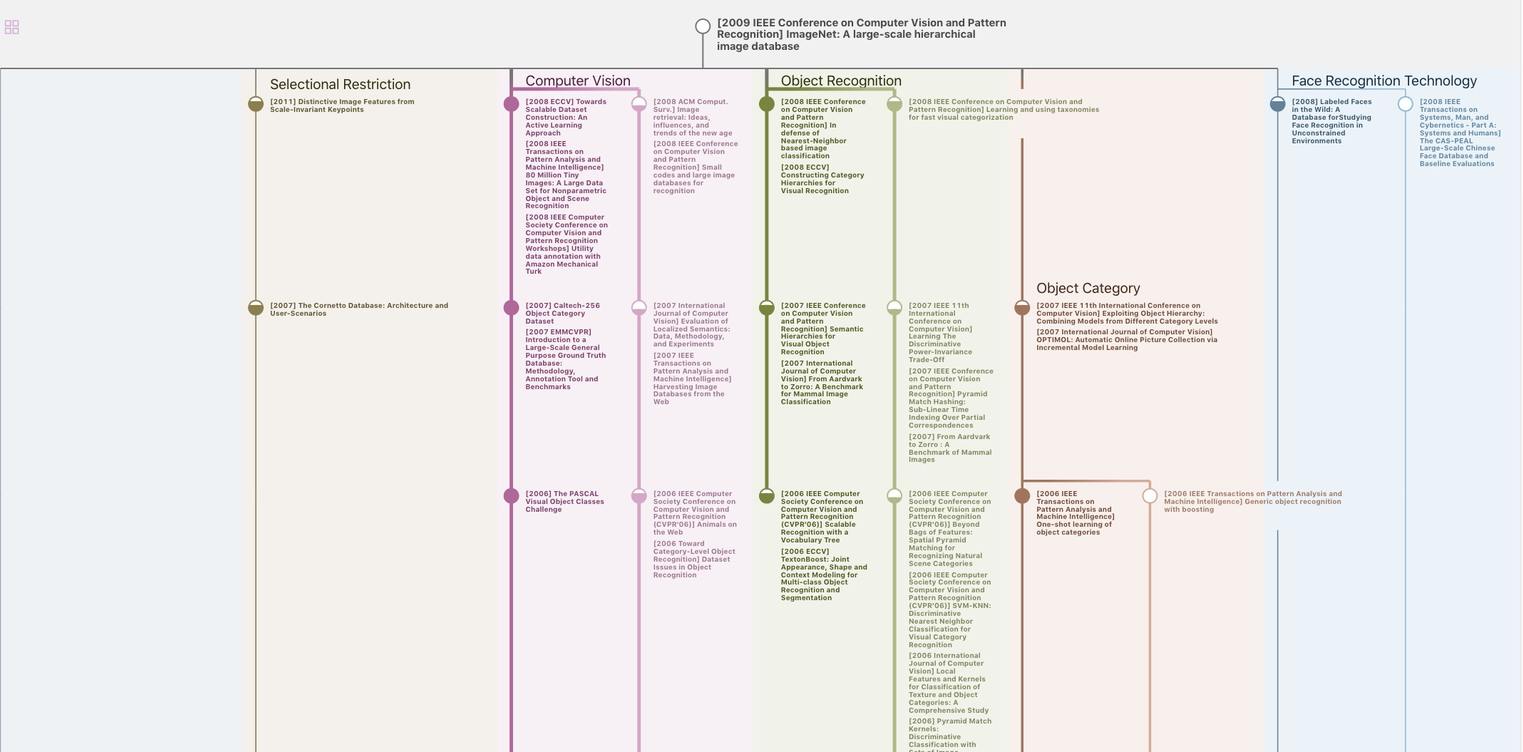
生成溯源树,研究论文发展脉络
Chat Paper
正在生成论文摘要