LUISA: Decoupling the Frequency Model From the Context Model in Prediction-Based Compression
The Computer Journal(2021)
摘要
Prediction-based compression methods, like prediction by partial matching, achieve a remarkable compression ratio, especially for texts written in natural language. However, they are not efficient in terms of speed. Part of the problem concerns the usage of dynamic entropy encoding, which is considerably slower than the static alternatives. In this paper, we propose a prediction-based compression method that decouples the context model from the frequency model. The separation allows static entropy encoding to be used without a significant overhead in the meta-data embedded in the compressed data. The result is a reasonably efficient algorithm that is particularly suited for small textual files, as the experiments show. We also show it is relatively easy to built strategies designed to handle specific cases, like the compression of files whose symbols are only locally frequent.
更多查看译文
关键词
data compression,lossless compression,prediction by partial matching,prediction tree,LUISA,compression methodology
AI 理解论文
溯源树
样例
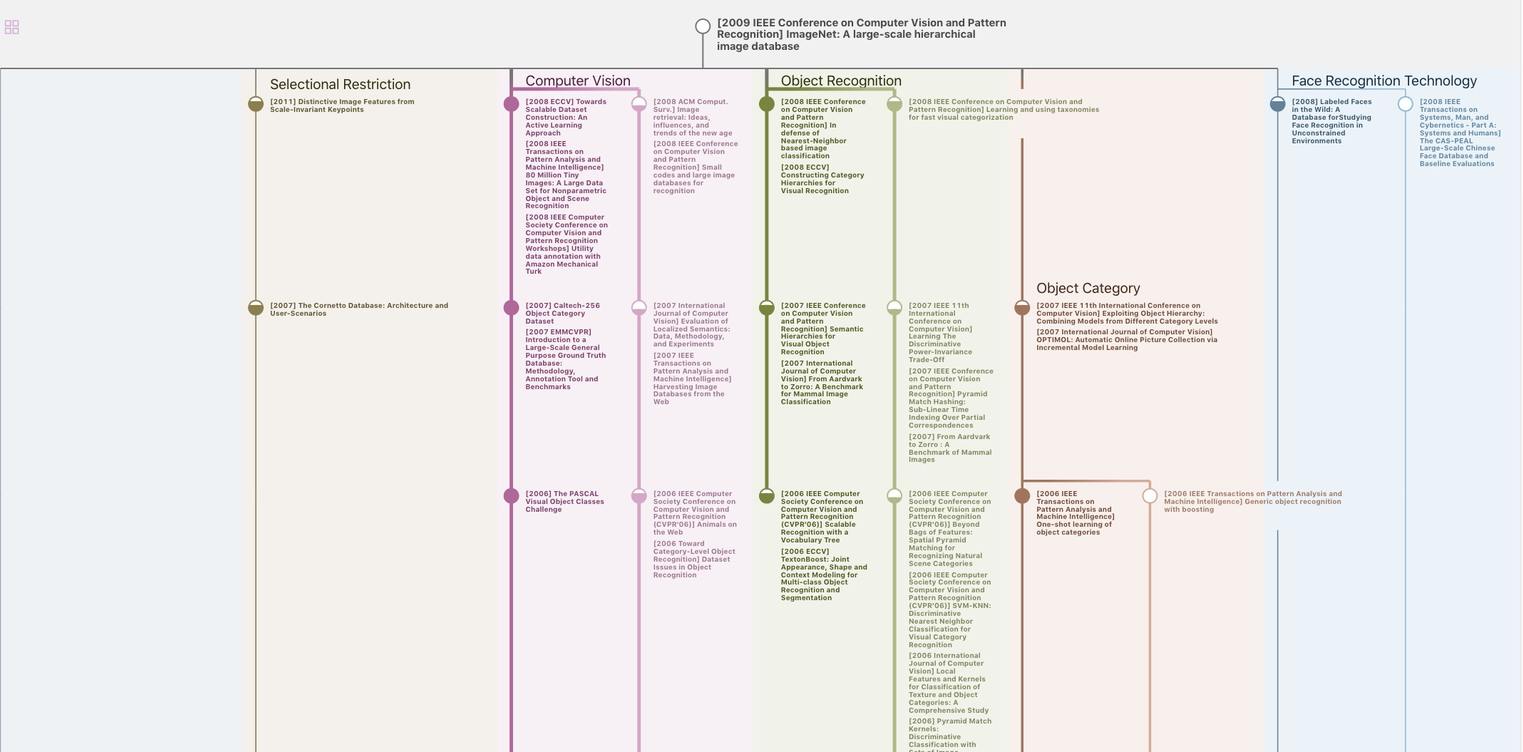
生成溯源树,研究论文发展脉络
Chat Paper
正在生成论文摘要