Interpreting Human Responses in Dialogue Systems using Fuzzy Semantic Similarity Measures
2020 IEEE International Conference on Fuzzy Systems (FUZZ-IEEE)(2020)
摘要
Dialogue systems are automated systems that interact with humans using natural language. Much work has been done on dialogue management and learning using a range of computational intelligence based approaches, however the complexity of human dialogue in different contexts still presents many challenges. The key impact of work presented in this paper is to use fuzzy semantic similarity measures embedded within a dialogue system to allow a machine to semantically comprehend human utterances in a given context and thus communicate more effectively with a human in a specific domain using natural language. To achieve this, perception based words should be understood by a machine in context of the dialogue. In this work, a simple question and answer dialogue system is implemented for a café customer satisfaction feedback survey. Both fuzzy and crisp semantic similarity measures are used within the dialogue engine to assess the accuracy and robustness of rule firing. Results from a 32 participant study, show that the fuzzy measure improves rule matching within the dialogue system by 21.88% compared with the crisp measure known as STASIS, thus providing a more natural and fluid dialogue exchange.
更多查看译文
关键词
dialogue systems,conversational agents,fuzzy semantic similarity measures,fuzzy natural language
AI 理解论文
溯源树
样例
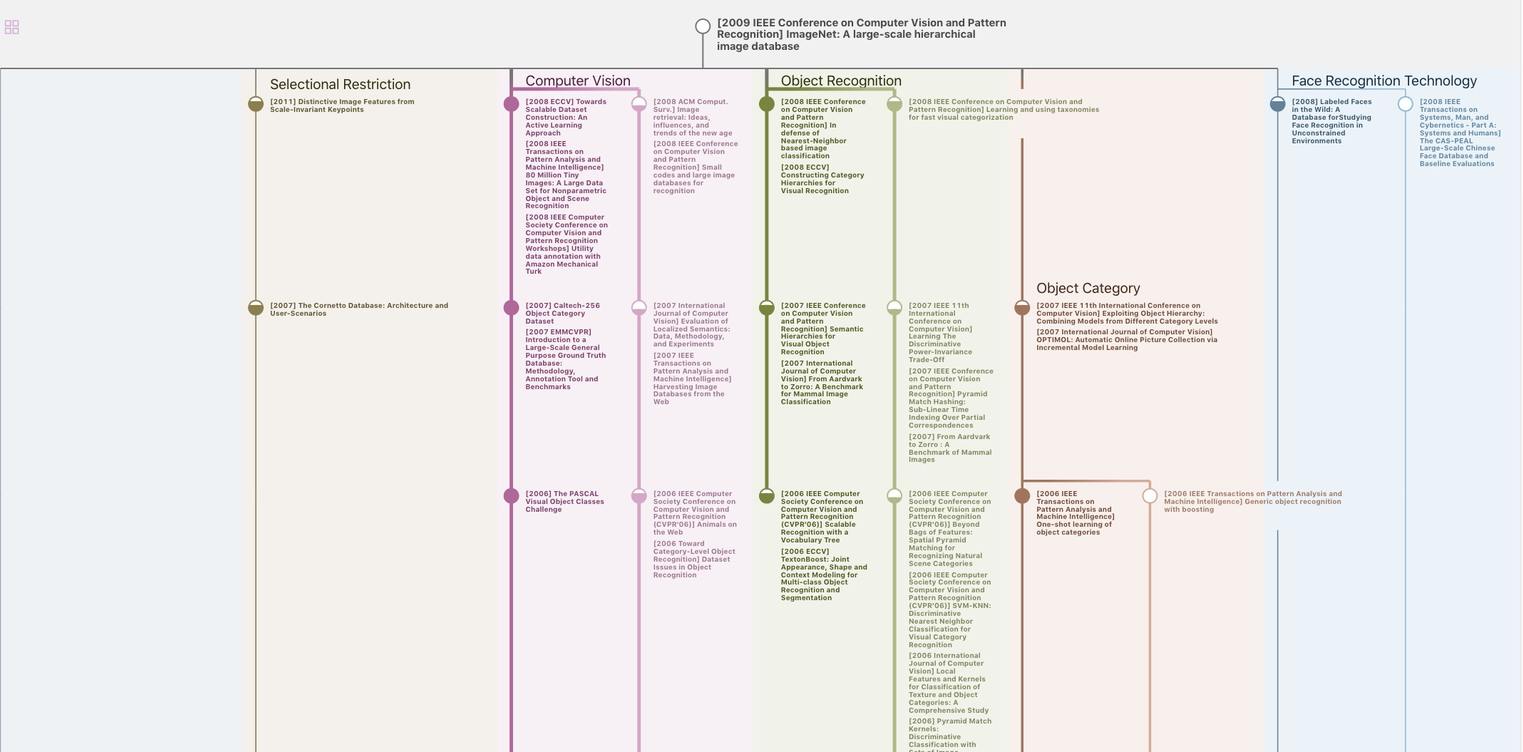
生成溯源树,研究论文发展脉络
Chat Paper
正在生成论文摘要