Sequential Deep Learning for Credit Risk Monitoring with Tabular Financial Data
arxiv(2020)
摘要
Machine learning plays an essential role in preventing financial losses in the banking industry. Perhaps the most pertinent prediction task that can result in billions of dollars in losses each year is the assessment of credit risk (i.e., the risk of default on debt). Today, much of the gains from machine learning to predict credit risk are driven by gradient boosted decision tree models. However, these gains begin to plateau without the addition of expensive new data sources or highly engineered features. In this paper, we present our attempts to create a novel approach to assessing credit risk using deep learning that does not rely on new model inputs. We propose a new credit card transaction sampling technique to use with deep recurrent and causal convolution-based neural networks that exploits long historical sequences of financial data without costly resource requirements. We show that our sequential deep learning approach using a temporal convolutional network outperformed the benchmark non-sequential tree-based model, achieving significant financial savings and earlier detection of credit risk. We also demonstrate the potential for our approach to be used in a production environment, where our sampling technique allows for sequences to be stored efficiently in memory and used for fast online learning and inference.
更多查看译文
关键词
credit risk monitoring,tabular financial data,deep learning
AI 理解论文
溯源树
样例
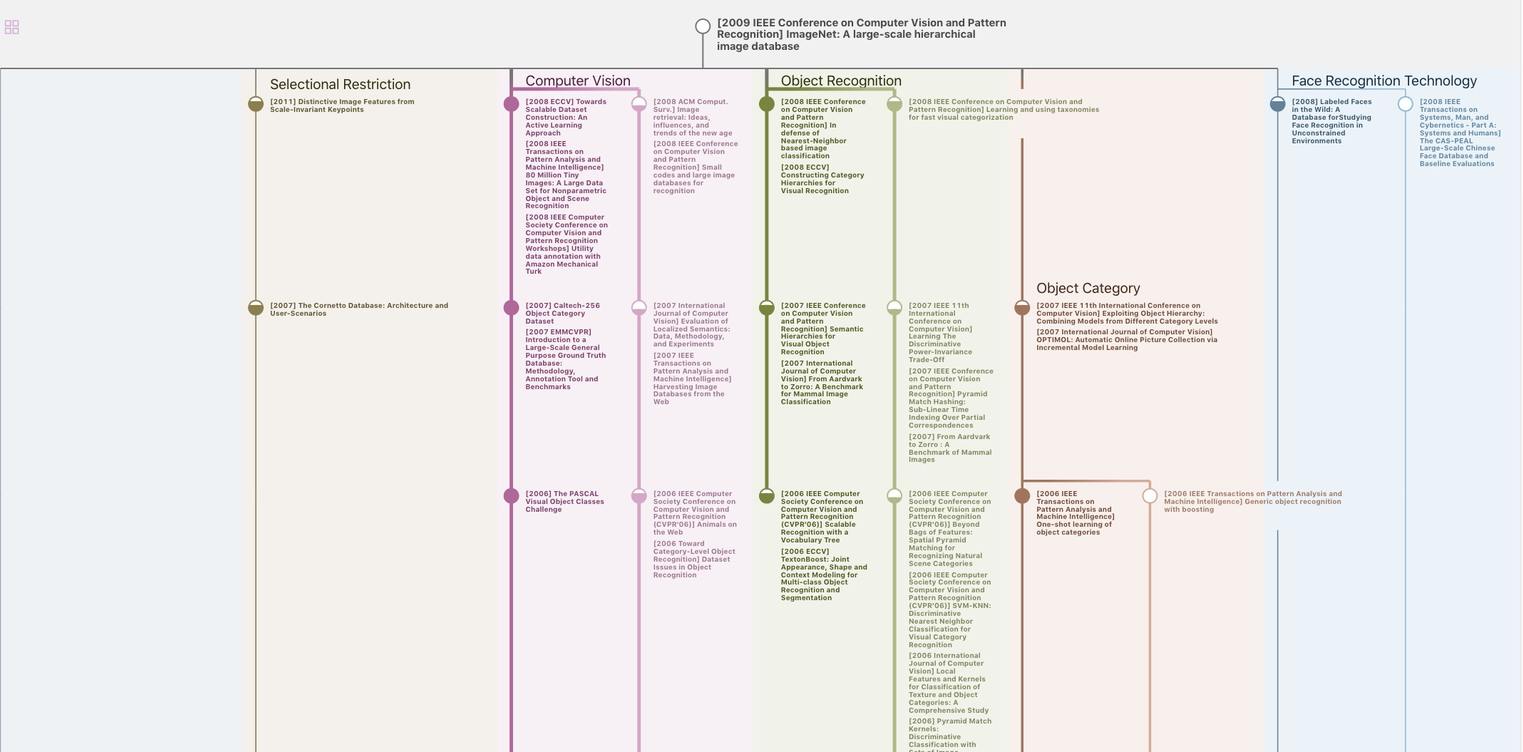
生成溯源树,研究论文发展脉络
Chat Paper
正在生成论文摘要