Open source and automatic subregional assessment of knee cartilage degradation using quantitative T2 relaxometry and deep learning
CARTILAGE(2021)
摘要
Objective. We evaluated a fully automated femoral cartilage segmentation model for measuring T2 relaxation values and longitudinal changes using multi-echo spin-echo (MESE) magnetic resonance imaging (MRI). We open sourced this model and developed a web app available at https://kl.stanford.edu into which users can drag and drop images to segment them automatically. Design. We trained a neural network to segment femoral cartilage from MESE MRIs. Cartilage was divided into 12 subregions along medial-lateral, superficial-deep, and anterior-central-posterior boundaries. Subregional T2 values and four-year changes were calculated using a radiologist's segmentations (Reader I) and the model's segmentations. These were compared using 28 held-out images. A subset of 14 images were also evaluated by a second expert (Reader 2) for comparison. Results. Model segmentations agreed with Reader I segmentations with a Dice score of 0.85 +/- 0.03. The model's estimated T2 values for individual subregions agreed with those of Reader I with an average Spearman correlation of 0.89 and average mean absolute error (MAE) of 1.34 ms. The model's estimated four-year change in T2 for individual subregions agreed with Reader 1 with an average correlation of 0.80 and average MAE of 1.72 ms. The model agreed with Reader I at least as closely as Reader 2 agreed with Reader I in terms of Dice score (0.85 vs. 0.75) and subregional T2 values. Conclusions. Assessments of cartilage health using our fully automated segmentation model agreed with those of an expert as closely as experts agreed with one another. This has the potential to accelerate osteoarthritis research.
更多查看译文
关键词
osteoarthritis, cartilage segmentation, T2 map MRI, neural network, deep learning
AI 理解论文
溯源树
样例
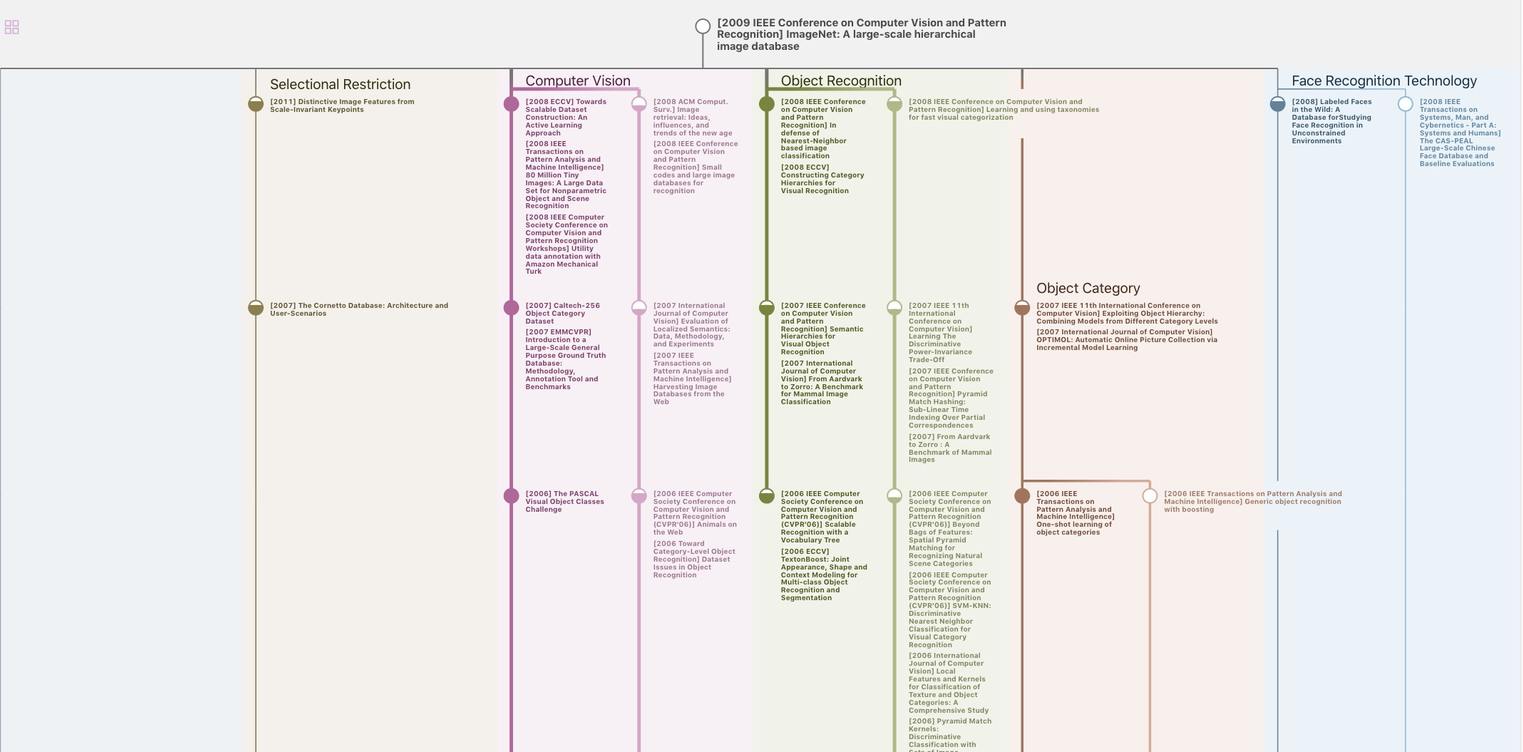
生成溯源树,研究论文发展脉络
Chat Paper
正在生成论文摘要