A Hierarchical Feature Constraint to Camouflage Medical Adversarial Attacks
MEDICAL IMAGE COMPUTING AND COMPUTER ASSISTED INTERVENTION - MICCAI 2021, PT III(2021)
摘要
Deep neural networks for medical images are extremely vulnerable to adversarial examples (AEs), which poses security concerns on clinical decision-making. Recent findings have shown that existing medical AEs are easy to detect in feature space. To better understand this phenomenon, we thoroughly investigate the characteristic of traditional medical AEs in feature space. Specifically, we first perform a stress test to reveal the vulnerability of medical images and compare them to natural images. Then, we theoretically prove that the existing adversarial attacks manipulate the prediction by continuously optimizing the vulnerable representations in a fixed direction, leading to outlier representations in feature space. Interestingly, we find this vulnerability is a double-edged sword that can be exploited to help hide AEs in the feature space. We propose a novel hierarchical feature constraint (HFC) as an add-on to existing white-box attacks, which encourages hiding the adversarial representation in the normal feature distribution. We evaluate the proposed method on two public medical image datasets, namely Fundoscopy and Chest X-Ray. Experimental results demonstrate the superiority of our HFC as it bypasses an array of state-of-the-art adversarial medical AEs detector more efficiently than competing adaptive attacks. Our code is available at https://github.com/qsyao/Hierarchical_Feature_Constraint.
更多查看译文
关键词
Adversarial attack, Adversarial defense
AI 理解论文
溯源树
样例
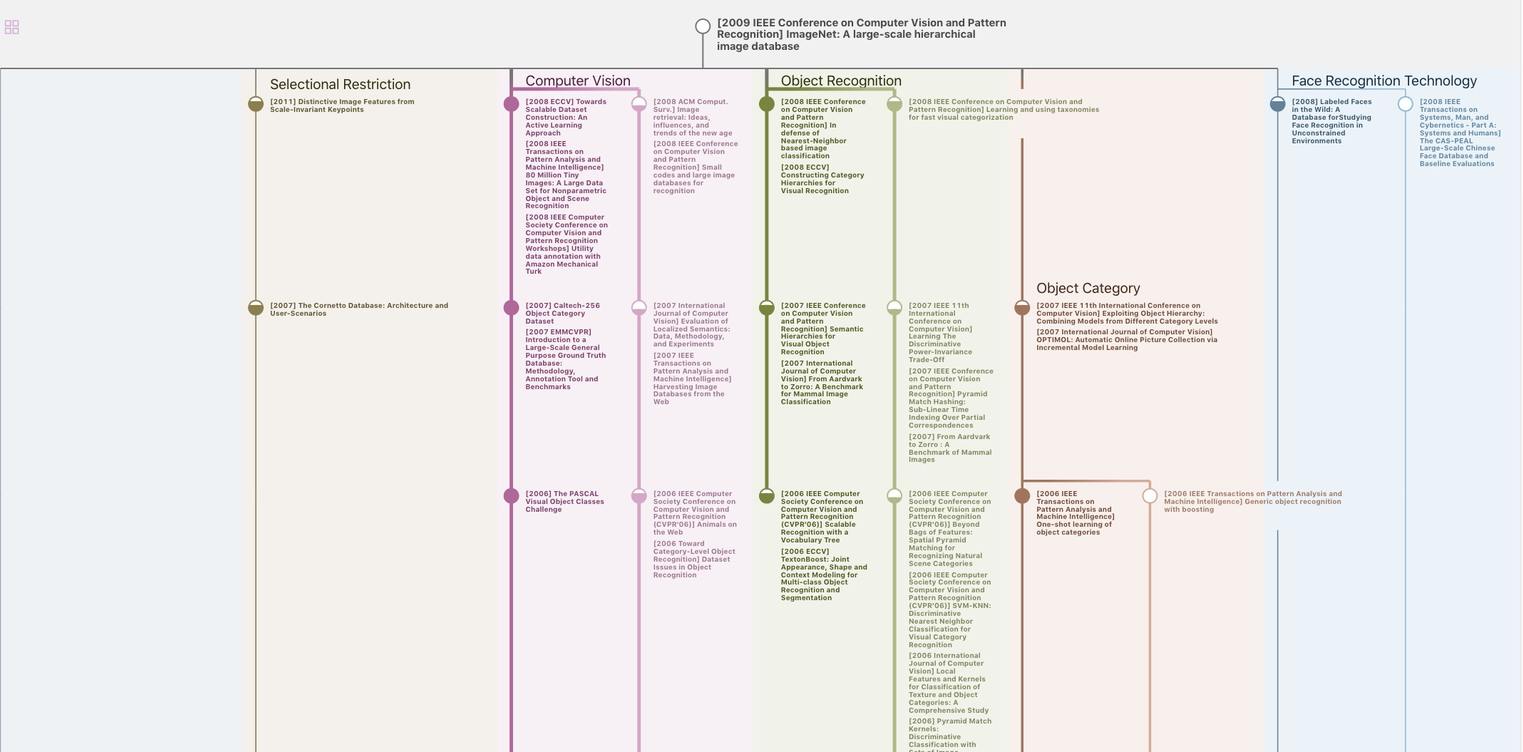
生成溯源树,研究论文发展脉络
Chat Paper
正在生成论文摘要