Variable Selection With Second-Generation P-Values
AMERICAN STATISTICIAN(2022)
摘要
Many statistical methods have been proposed for variable selection in the past century, but few balance inference and prediction tasks well. Here, we report on a novel variable selection approach called penalized regression with second-generation p-values (ProSGPV). It captures the true model at the best rate achieved by current standards, is easy to implement in practice, and often yields the smallest parameter estimation error. The idea is to use an l(0) penalization scheme with second-generation p-values (SGPV), instead of traditional ones, to determine which variables remain in a model. The approach yields tangible advantages for balancing support recovery, parameter estimation, and prediction tasks. The ProSGPV algorithm can maintain its good performance even when there is strong collinearity among features or when a high-dimensional feature space with p > n is considered. We present extensive simulations and a real-world application comparing the ProSGPV approach with smoothly clipped absolute deviation (SCAD), adaptive lasso (AL), and minimax concave penalty with penalized linear unbiased selection (MC+). While the last three algorithms are among the current standards for variable selection, ProSGPV has superior inference performance and comparable prediction performance in certain scenarios.
更多查看译文
关键词
Lasso, Penalized regression, Second-generation p-values, Variable selection
AI 理解论文
溯源树
样例
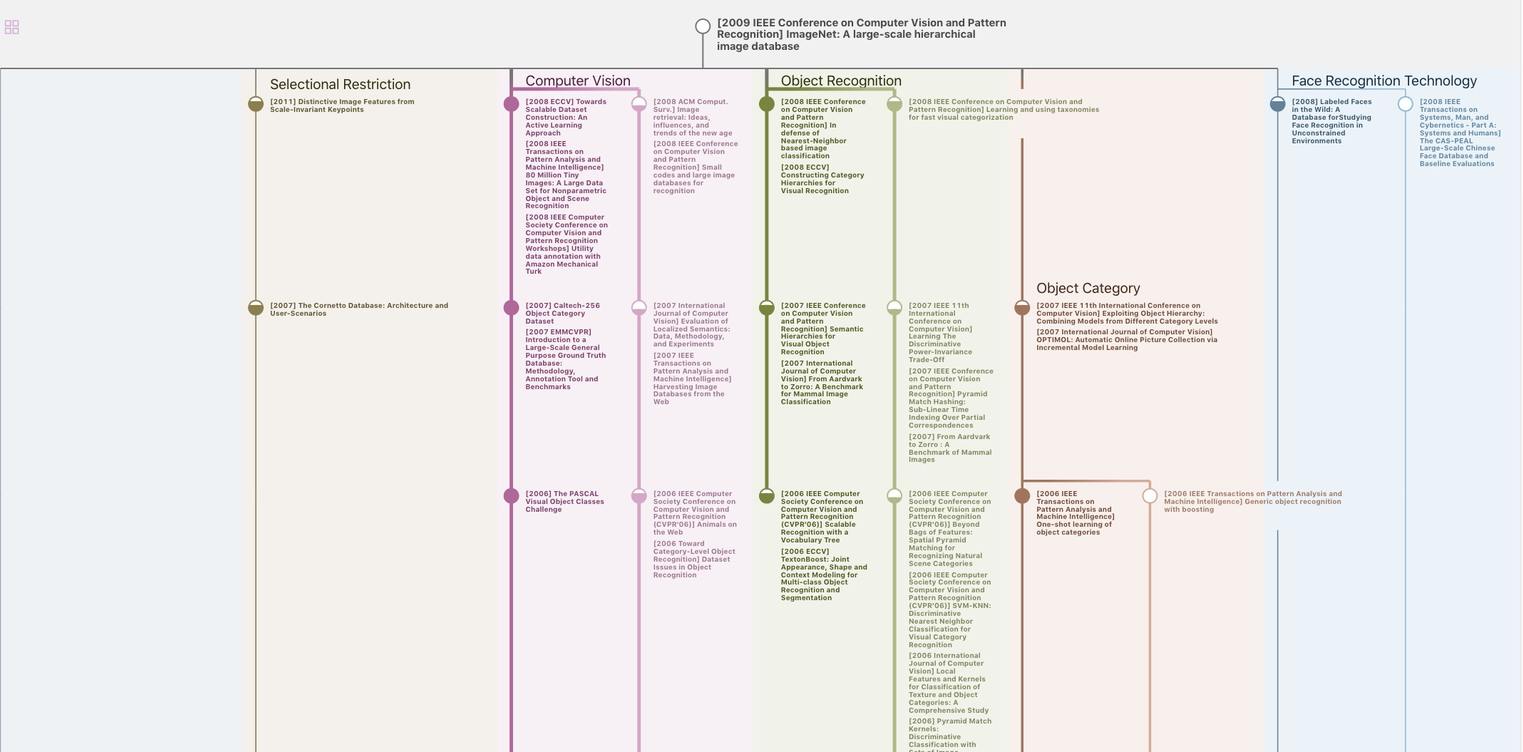
生成溯源树,研究论文发展脉络
Chat Paper
正在生成论文摘要