A Free Lunch For Unsupervised Domain Adaptive Object Detection Without Source Data
THIRTY-FIFTH AAAI CONFERENCE ON ARTIFICIAL INTELLIGENCE, THIRTY-THIRD CONFERENCE ON INNOVATIVE APPLICATIONS OF ARTIFICIAL INTELLIGENCE AND THE ELEVENTH SYMPOSIUM ON EDUCATIONAL ADVANCES IN ARTIFICIAL INTELLIGENCE(2021)
摘要
Unsupervised domain adaptation (UDA) assumes that source and target domain data are freely available and trained to-gether to reduce the domain gap. Considering the data privacy, it is impractical in real scenarios. Hence, it draws our eyes to optimize the network in the target domain without labeled source data. To explore this direction in object detection, for the first time, we propose a source data-free domain adaptive object detection (SFOD) framework via modeling it into learning with noisy labels. A straightforward method is to leverage the pre-trained network from the source domain to generate the pseudo labels for target domain. However, it is difficult to evaluate the quality of pseudo labels since no labels are available in target domain. In this paper, self-entropy descent (SED) is a metric proposed to search an appropriate confidence threshold for reliable pseudo label generation. Nonetheless, completely clean labels are still unattainable. After an experimental analysis, false negatives are found to dominate in the generated noisy labels. Undoubtedly, false negatives mining is helpful for improvement, and we ease it to false negatives simulation through data augmentation like Mosaic. Extensive experiments conducted in four adaptation tasks have demonstrated that the proposed framework can easily achieve state-of-the-art performance. From another view, it also reminds the UDA community that the labeled source data are not fully exploited in the existing methods.
更多查看译文
关键词
detection,domain
AI 理解论文
溯源树
样例
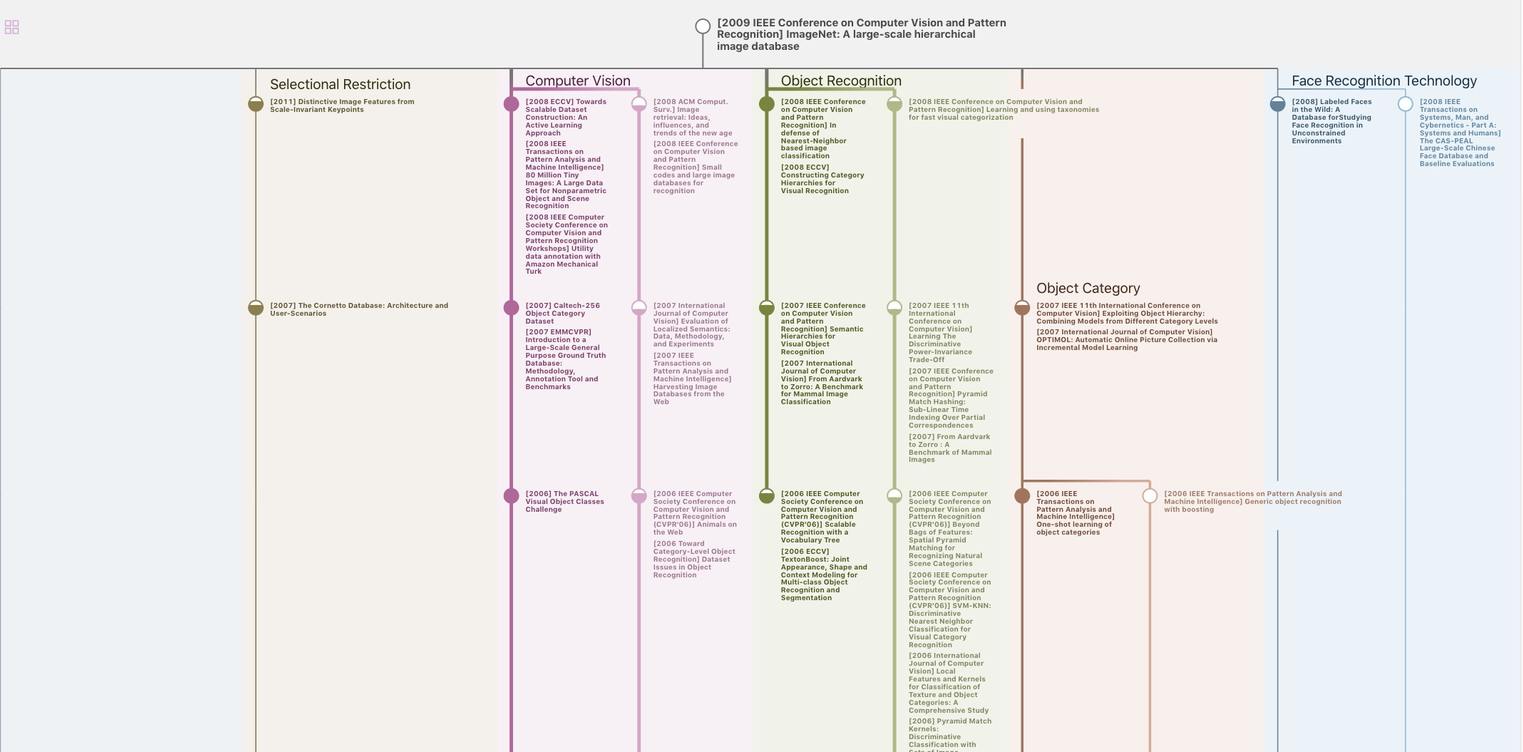
生成溯源树,研究论文发展脉络
Chat Paper
正在生成论文摘要