A New Framework for Registration of Semantic Point Clouds from Stereo and RGB-D Cameras
2021 IEEE INTERNATIONAL CONFERENCE ON ROBOTICS AND AUTOMATION (ICRA 2021)(2021)
摘要
This paper reports on a novel nonparametric rigid point cloud registration framework, Semantic Continuous Visual Odometry (CVO), that jointly integrates geometric and semantic measurements such as color or semantic labels into the alignment process and does not require explicit data association. The point clouds are represented as nonparametric functions in a reproducible kernel Hilbert space. The alignment problem is formulated as maximizing the inner product between two functions, essentially a sum of weighted kernels, each of which exploits the local geometric and semantic features. As a result of the continuous models, analytical gradients can be computed, and a local solution can be obtained by optimization over the rigid body transformation group. Besides, we present a new point cloud alignment metric that is intrinsic to the proposed framework and takes into account geometric and semantic information. The evaluations using publicly available stereo and RGB-D datasets show that the proposed method outperforms state-of-the-art outdoor and indoor frame-to-frame registration methods. An open-source GPU implementation is also provided.
更多查看译文
关键词
semantic point clouds,RGB-D cameras,nonparametric rigid point cloud registration framework,semantic labels,alignment process,data association,nonparametric functions,reproducible kernel Hilbert space,alignment problem,weighted kernels,local geometric features,semantic features,rigid body transformation group,point cloud alignment metric,semantic information,frame-to-frame registration methods,semantic continuous visual odometry,stereo cameras
AI 理解论文
溯源树
样例
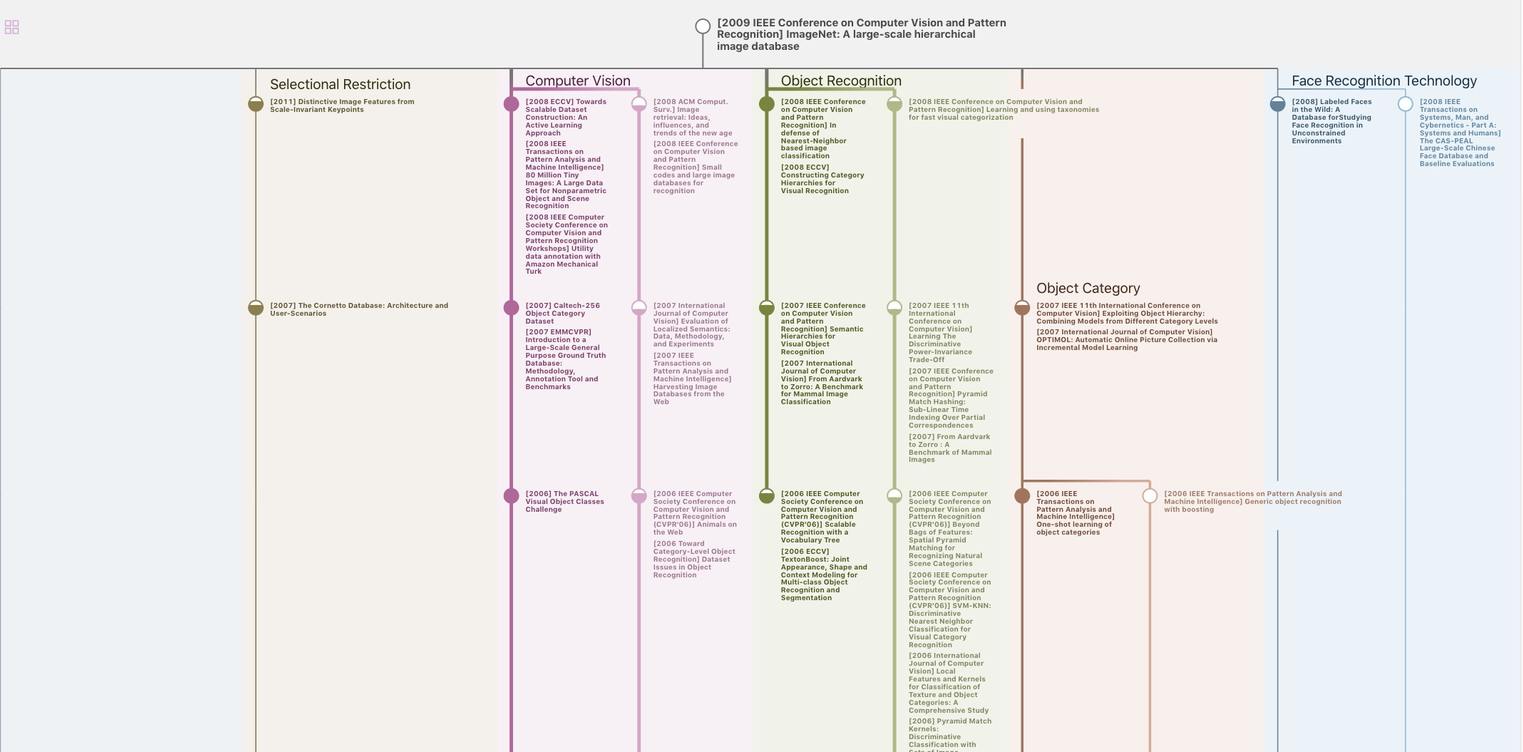
生成溯源树,研究论文发展脉络
Chat Paper
正在生成论文摘要