A novel learning algorithm based on computing the rules’ desired outputs of a TSK fuzzy neural network with non-separable fuzzy rules
Neurocomputing(2022)
摘要
In this paper, a novel learning approach to train fuzzy neural networks’ parameters based on calculating the desired outputs of their rules, is proposed. We describe the desired outputs of fuzzy rules as values that make the output error equal to the minimum. To find these desired outputs, a new constrained convex optimization problem is introduced and solved. Afterward, the parameters of fuzzy rules are trained to reduce the error between the current rules’ outputs and the estimated desired ones. Therefore, the proposed learning method avoids direct output error backpropagation, which leads to vanishing gradient and consequently getting stuck in a local optimum. Therefore, the proposed method does not need any sophisticated initialization method. This learning method is successfully utilized to train a new Takagi–Sugeno-Kang (TSK) Fuzzy Neural Network with correlated fuzzy rules. The proposed paradigm, including the proposed TSK correlation-aware architecture along with the learning method, is successfully applied to six real-world time-series predictions, regression problems, and nonlinear system identification. According to the experimental results, the performance of our proposed method outperforms other methods with a more parsimonious structure.
更多查看译文
关键词
Takagi-Sugeno-Kang (TSK) fuzzy neural networks,Non-separable fuzzy rules,Constrained convex optimization problem,Correlation-aware architecture,Gradient descent,Nonlinear function approximation,Time-series prediction
AI 理解论文
溯源树
样例
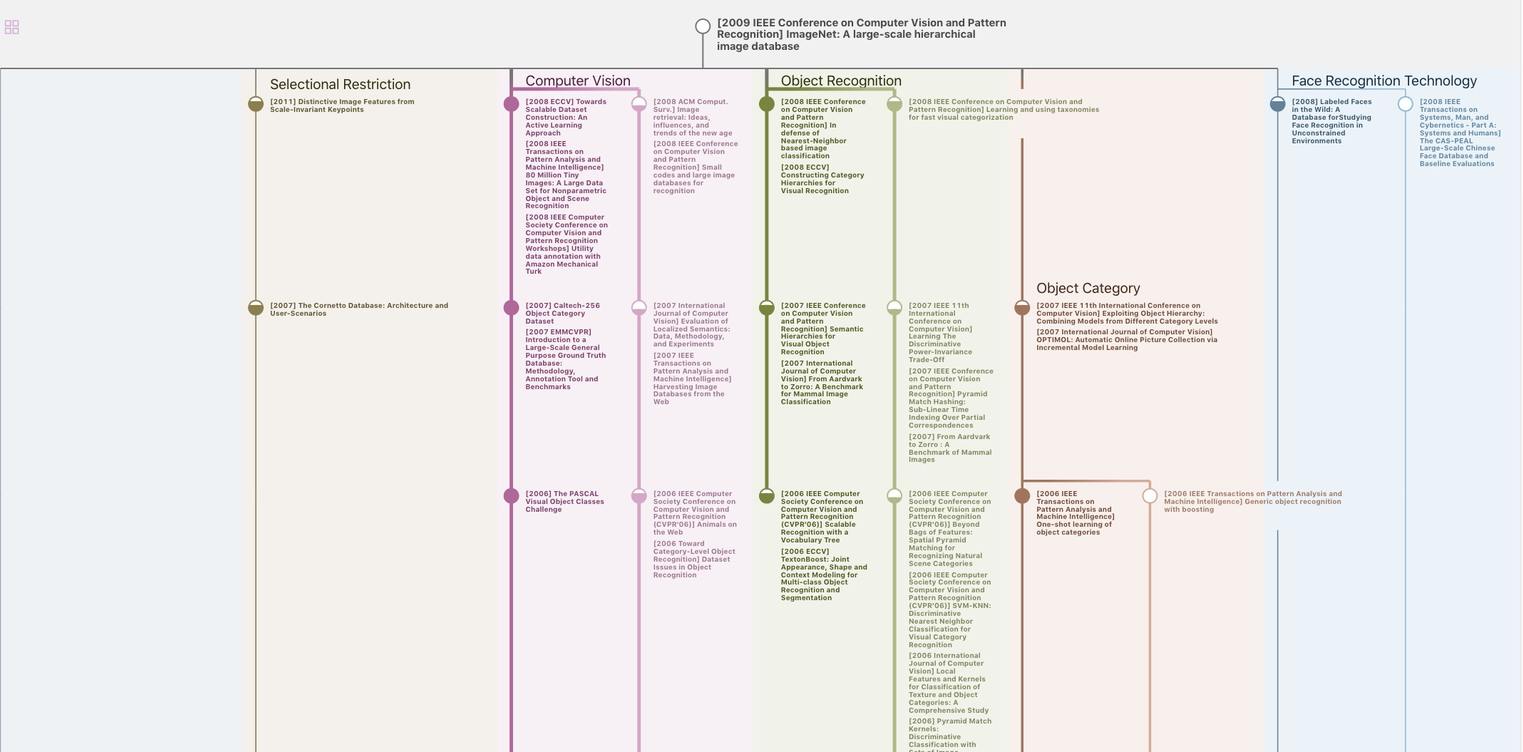
生成溯源树,研究论文发展脉络
Chat Paper
正在生成论文摘要