Interpretability and Explainability: A Machine Learning Zoo Mini-tour
arxiv(2023)
摘要
In this review, we examine the problem of designing interpretable and explainable machine learning models. Interpretability and explainability lie at the core of many machine learning and statistical applications in medicine, economics, law, and natural sciences. Although interpretability and explainability have escaped a clear universal definition, many techniques motivated by these properties have been developed over the recent 30 years with the focus currently shifting towards deep learning methods. In this review, we emphasise the divide between interpretability and explainability and illustrate these two different research directions with concrete examples of the state-of-the-art. The review is intended for a general machine learning audience with interest in exploring the problems of interpretation and explanation beyond logistic regression or random forest variable importance. This work is not an exhaustive literature survey, but rather a primer focusing selectively on certain lines of research which the authors found interesting or informative.
更多查看译文
关键词
explainability,zoo,learning,mini-tour
AI 理解论文
溯源树
样例
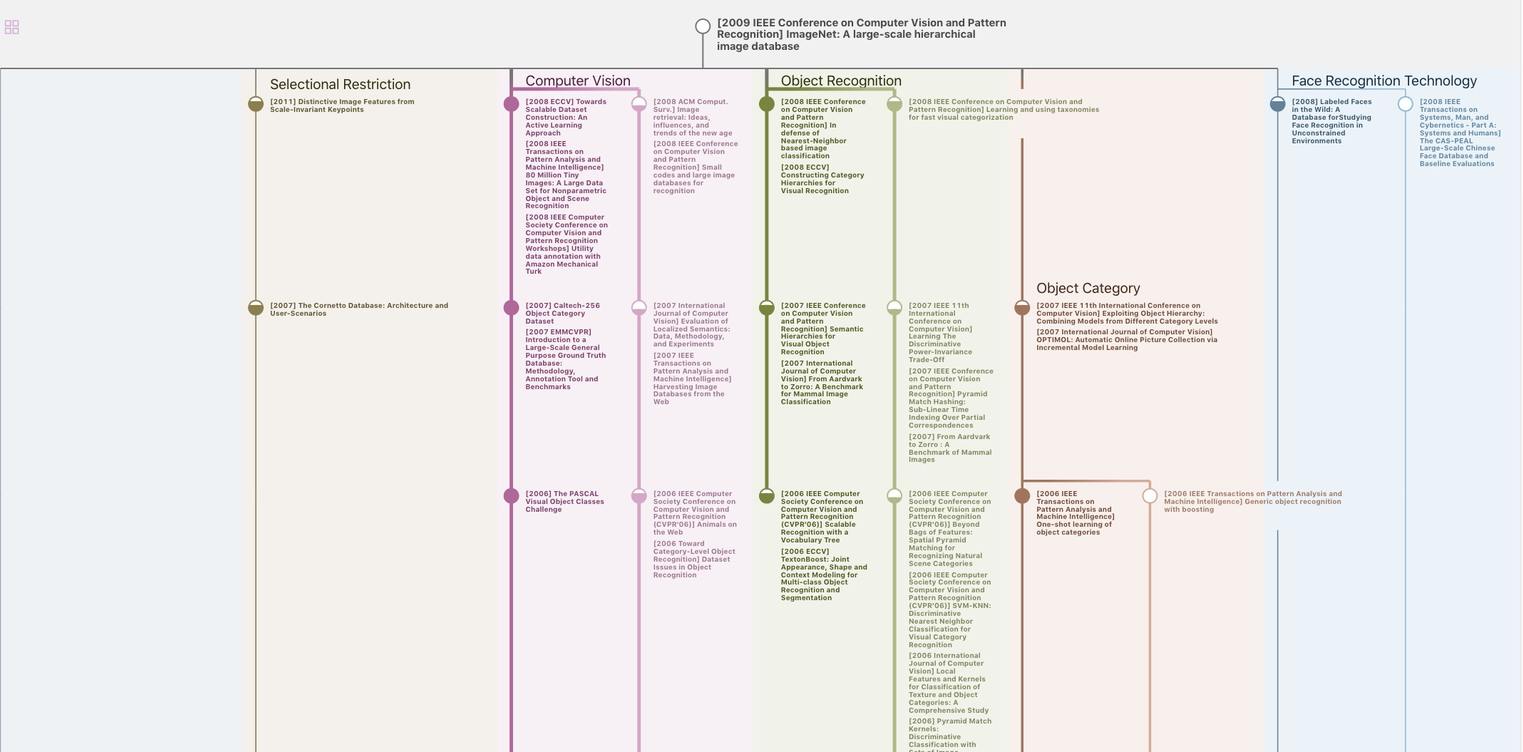
生成溯源树,研究论文发展脉络
Chat Paper
正在生成论文摘要