Towards Low Latency Multi-viewpoint 360 degrees Interactive Video: A Multimodal Deep Reinforcement Learning Approach
IEEE INFOCOM(2019)
摘要
Recently, the fusion of 360 video and multi viewpoint video, called multi-viewpoint (MVP) 360 interactive video, has emerged and created much more immersive and interactive user experience, but calls for a low latency solution to request the high-definition contents. Such viewing-related features as head movement have been recently studied, but several key issues still need to be addressed. On the viewer side, it is not clear how to effectively integrate different types of viewing-related features. At the session level, questions such as how to optimize the video quality under dynamic networking conditions and how to build an end-to-end mapping between these features and the quality selection remain to be answered. The solutions to these questions are further complicated given the many practical challenges, e.g., incomplete feature extraction and inaccurate prediction. This paper presents an architecture, called iView, to address the aforementioned issues in an MVP 360 interactive video scenario. To fully understand the viewing-related features and provide a one-step solution, we advocate multimodal learning and deep reinforcement learning in the design. iView intelligently determines video quality and reduces the latency without preprogrammed models or assumptions. We have evaluated iView with multiple real-world video and network datasets. The results showed that our solution effectively utilizes the features of video frames, networking throughput, head movements, and viewpoint selections, achieving at least 27.2%, 15.4%, and 2.8% improvements on the three video datasets, respectively, compared with several state-of-the-art methods.
更多查看译文
AI 理解论文
溯源树
样例
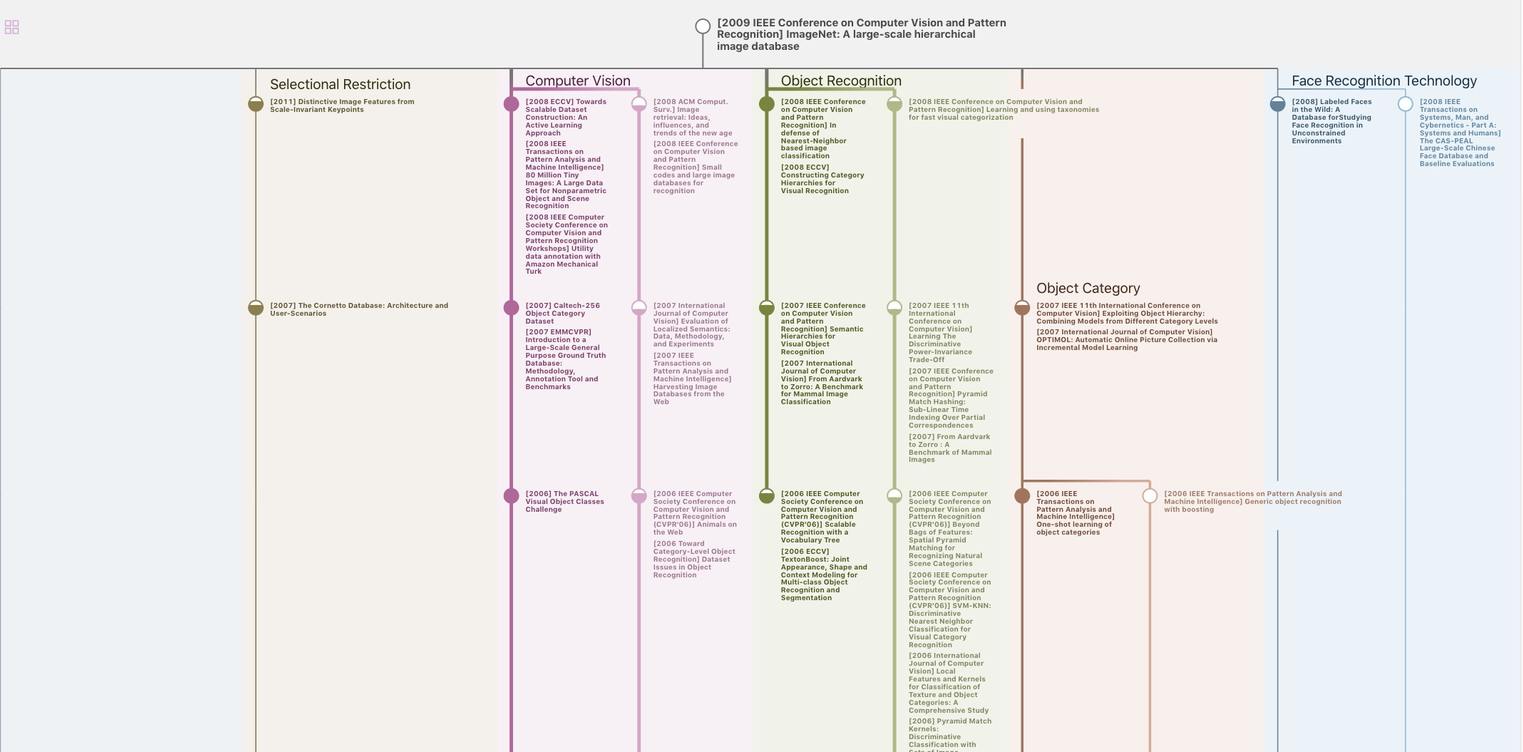
生成溯源树,研究论文发展脉络
Chat Paper
正在生成论文摘要