A novel patterning control strategy based on real-time fingerprint recognition and adaptive wafer level scanner optimization
Proceedings of SPIE(2018)
摘要
In addition to lithography process and equipment induced variations, processes like etching, annealing, film deposition and planarization exhibit variations, each having their own intrinsic characteristics and leaving an effect, a 'fingerprint', on the wafers. With ever tighter requirements for CD and overlay, controlling these process induced variations is both increasingly important and increasingly challenging in advanced integrated circuit (IC) manufacturing. For example, the on-product overlay (OPO) requirement for future nodes is approaching <3nm, requiring the allowable budget for process induced variance to become extremely small. Process variance control is seen as an bottleneck to further shrink which drives the need for more sophisticated process control strategies. In this context we developed a novel 'computational process control strategy' which provides the capability of proactive control of each individual wafer with aim to maximize the yield, without introducing a significant impact on metrology requirements, cycle time or productivity. The complexity of the wafer process is approached by characterizing the full wafer stack building a fingerprint library containing key patterning performance parameters like Overlay, Focus, etc. Historical wafer metrology is decomposed into dominant fingerprints using Principal Component Analysis. By associating observed fingerprints with their origin e.g. process steps, tools and variables, we can give an inline assessment of the strength and origin of the fingerprints on every wafer. Once the fingerprint library is established, a wafer specific fingerprint correction recipes can be determined based on its processing history. Data science techniques are used in real-time to ensure that the library is adaptive. To realize this concept, ASML TWINSCAN (TM) scanners play a vital role with their on-board full wafer detection and exposure correction capabilities. High density metrology data is created by the scanner for each wafer and on every layer during the lithography steps. This metrology data will be used to obtain the process fingerprints. Also, the per exposure and per wafer correction potential of the scanners will be utilized for improved patterning control. Additionally, the fingerprint library will provide early detection of excursions for inline root cause analysis and process optimization guidance.
更多查看译文
关键词
Patterning Fingerprints,Process Control,Overlay,Focus,Alignment,Leveling,Yield,Fingerprint Correction,Data Mining,Principal Component Analysis
AI 理解论文
溯源树
样例
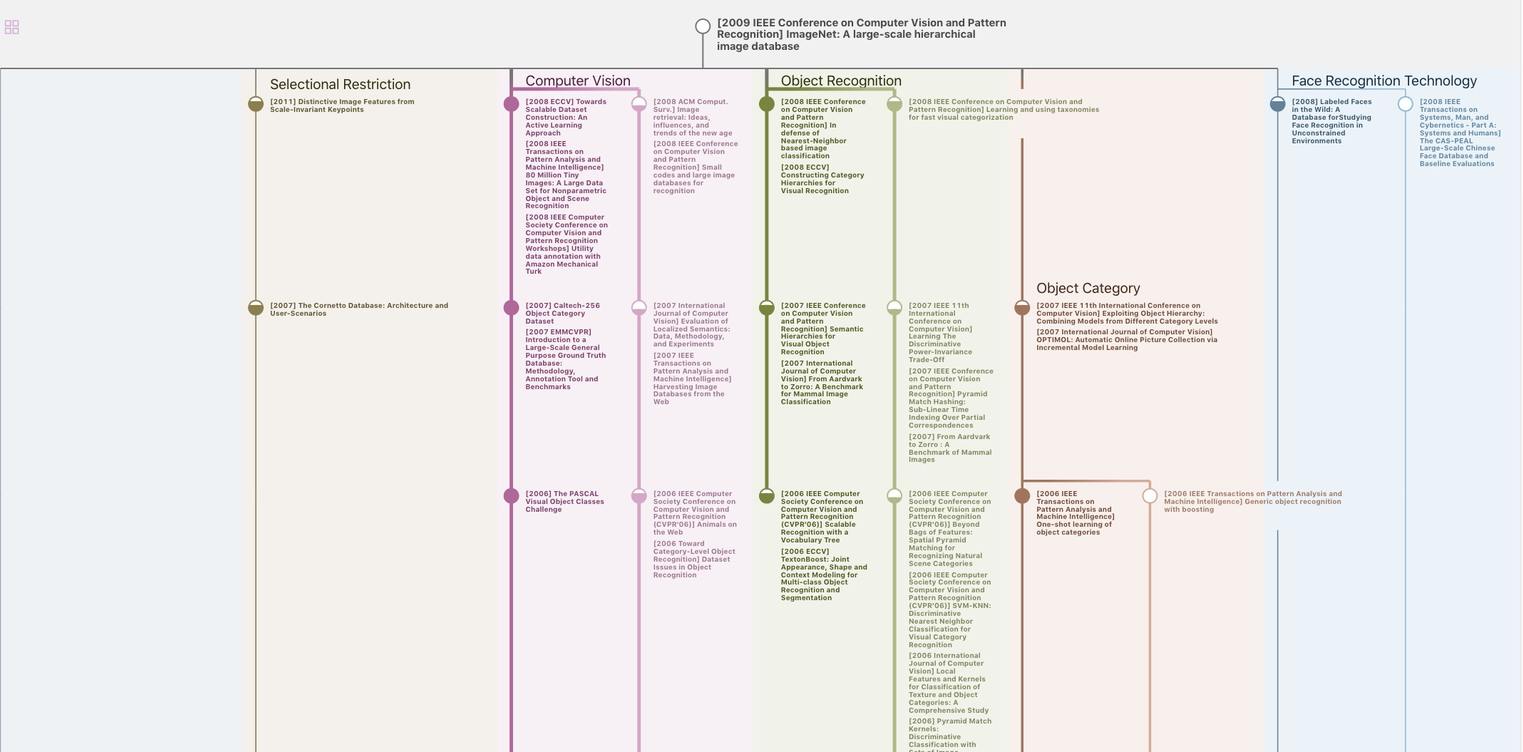
生成溯源树,研究论文发展脉络
Chat Paper
正在生成论文摘要