An Once-for-All Budgeted Pruning Framework for ConvNets Considering Input Resolution
IEEE Conference on Computer Vision and Pattern Recognition(2022)
摘要
We propose an efficient once-for-all budgeted pruning framework (OFARPruning) to find many compact network architectures close to winner tickets in the early training stage considering the effect of input resolution during the pruning process. In architecture searching stage, we mea-sure the similarity of the pruning mask to get high-quality network architecture with low energy and time consumption. After searching stage, our proposed method randomly sample the compact architectures with different pruning rates and input resolution to achieve joint optimization. Ultimately, we can obtain a cohort of compact networks adaptive to various resolution to meet dynamic FLOPs constraints on different edge devices with only once training. The experiments based on image classification and object detection show that OFARPruning has a higher accuracy than the once-for-all compression methods such as US-Net and MutualNet (1-2% better with less FLOPs), and achieve competitive performance as the conventional pruning methods (72.6% vs. 70.5% on MobileNetv2 under 170 MFLOPs) with much higher efficiency.
更多查看译文
关键词
convnets,budgeted pruning framework,input resolution,once-for-all
AI 理解论文
溯源树
样例
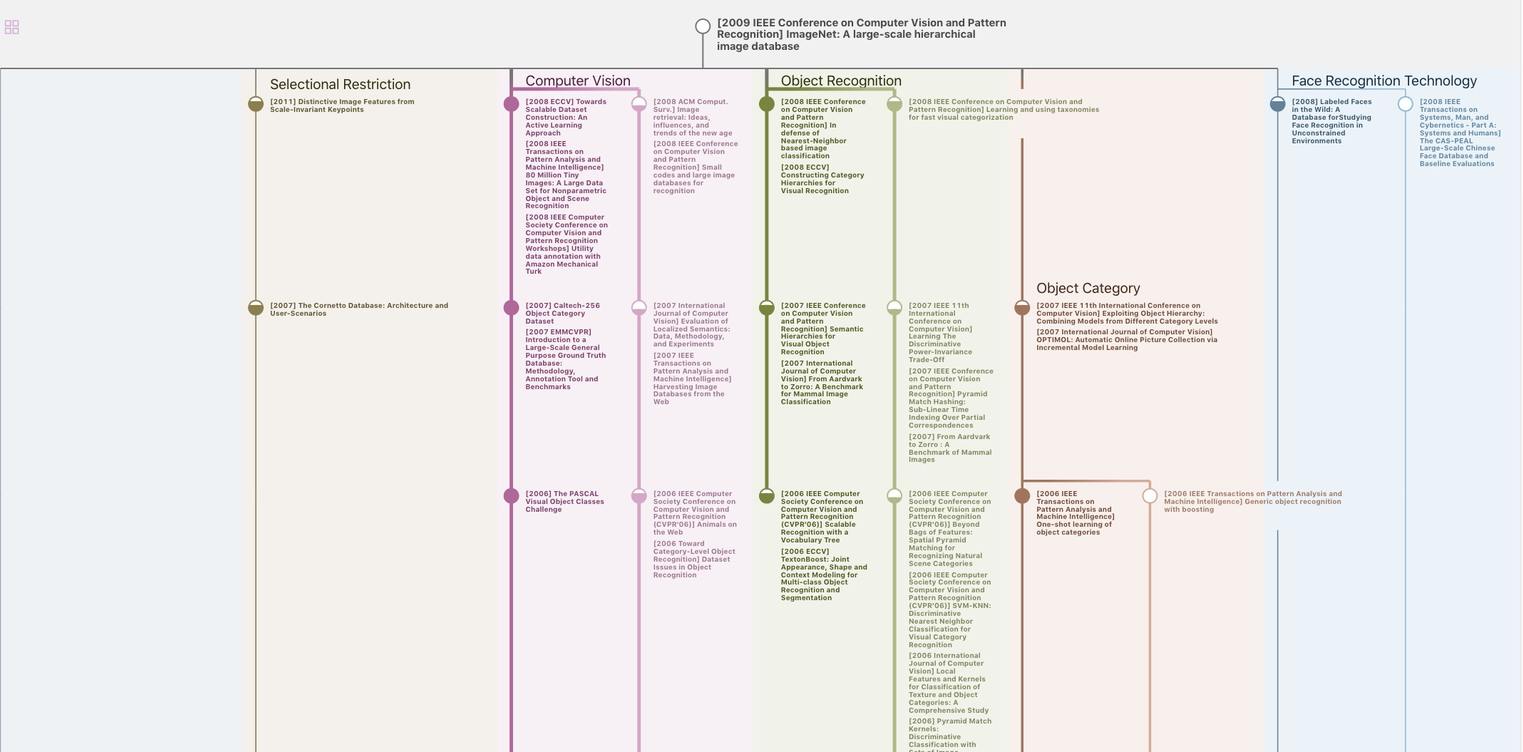
生成溯源树,研究论文发展脉络
Chat Paper
正在生成论文摘要