Pre-Trained Image Processing Transformer
2021 IEEE/CVF CONFERENCE ON COMPUTER VISION AND PATTERN RECOGNITION, CVPR 2021(2021)
摘要
As the computing power of modern hardware is increasing strongly, pre-trained deep learning models (e.g., BERT, GPT-3) learned on large-scale datasets have shown their effectiveness over conventional methods. The big progress is mainly contributed to the representation ability of transformer and its variant architectures. In this paper, we study the low-level computer vision task (e.g., denoising, super-resolution and deraining) and develop a new pre-trained model, namely, image processing transformer (IPT). To maximally excavate the capability of transformer, we present to utilize the well-known ImageNet benchmark for generating a large amount of corrupted image pairs. The IPT model is trained on these images with multi-heads and multi-tails. In addition, the contrastive learning is introduced for well adapting to different image processing tasks. The pre-trained model can therefore efficiently employed on desired task after fine-tuning. With only one pre-trained model, IPT outperforms the current state-of-the-art methods on various low-level benchmarks.
更多查看译文
关键词
low-level computer vision task,corrupted image pairs,IPT model,large-scale datasets,ImageNet benchmark,contrastive learning,pretrained image processing transformer
AI 理解论文
溯源树
样例
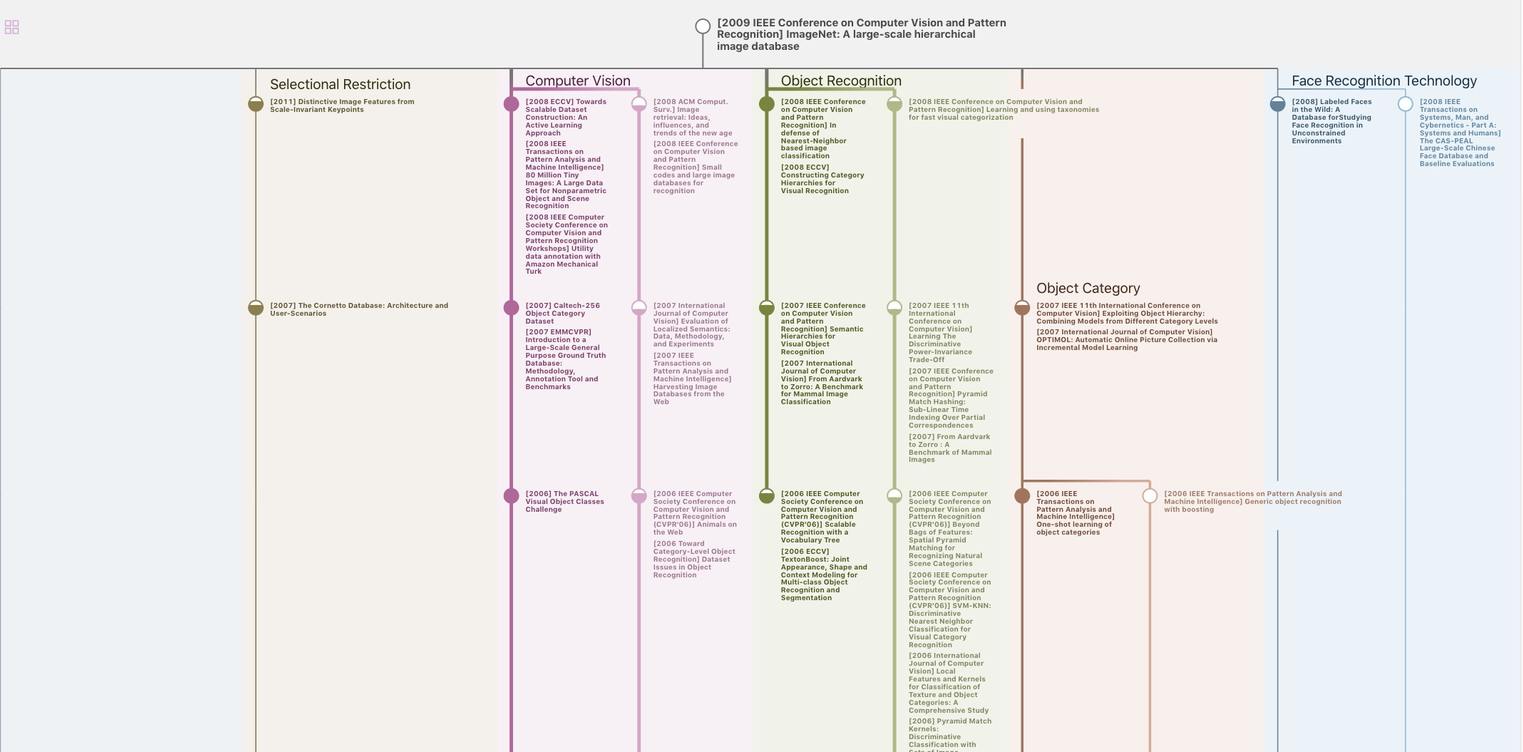
生成溯源树,研究论文发展脉络
Chat Paper
正在生成论文摘要