Diverse Temporal Aggregation and Depthwise Spatiotemporal Factorization for Efficient Video Classification
IEEE ACCESS(2021)
摘要
Video classification researches have recently attracted attention in the fields of temporal modeling and efficient 3D convolutional architectures. However, the temporal modeling methods are not efficient, and there is little interest in how to deal with temporal modeling in the 3D efficient architectures. To build an efficient 3D architecture for temporal modeling, we propose a new 3D backbone network, called VoV3D, that consists of a temporal one-shot aggregation (T-OSA) module and a depthwise factorized component, D(2 + 1)D. The T-OSA is devised to build a feature hierarchy by aggregating spatiotemporal features with different temporal receptive fields. Stacking this T-OSA enables the network itself to model short-range as well as long-range temporal relationships across frames without any external modules. We also design a depthwise spatiotemporal factorization module, D(2 + 1)D, that decomposes a 3D depthwise convolution into two spatial and temporal depthwise convolutions for efficient architecture. Through the proposed temporal modeling method (T-OSA) and the efficient factorization module (D(2 + 1)D), we construct two types of VoV3D networks: VoV3D-M and VoV3D-L. Thanks to its efficiency and effectiveness of their temporal modeling, VoV3D-L has 4 fi fewer model parameters and 14 fi less computation, surpassing the state-of-the-art TEA model on both Something-Something and Kinetics-400 datasets. We hope that VoV3D can serve as a baseline for efficient temporal modeling architecture.
更多查看译文
关键词
Action recognition, video classification, temporal modeling, efficient 3D CNN architecture, spatial-temporal feature
AI 理解论文
溯源树
样例
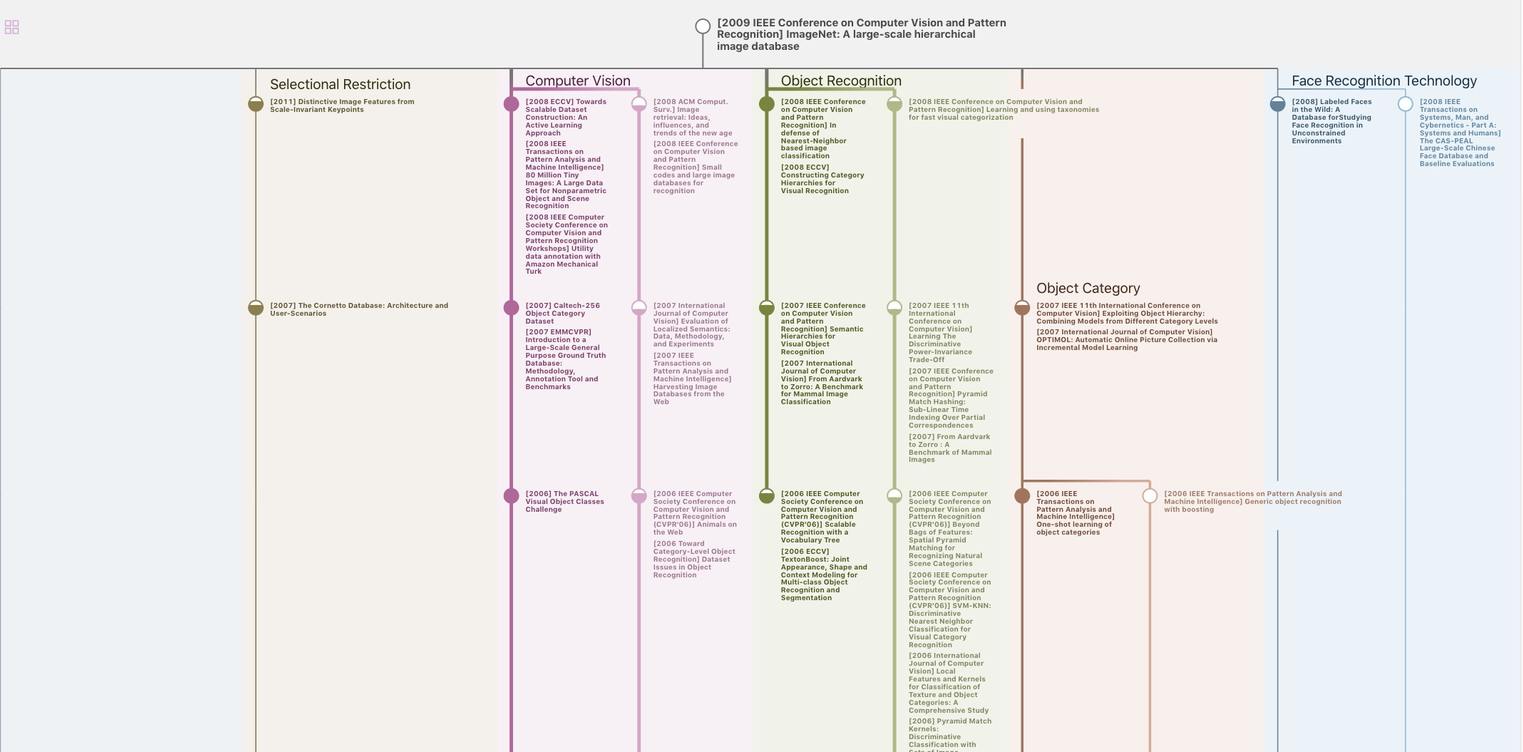
生成溯源树,研究论文发展脉络
Chat Paper
正在生成论文摘要