Near infrared spectrometric technique for testing fruit quality: Optimisation of regression models using genetic algorithms
Proceedings of SPIE(2017)
摘要
Near infrared (NIR) spectroscopy has gained extensive use in quality evaluation. It is arguably one of the most advanced spectroscopic tools in non-destructive quality testing of food stuff, from measurement to data analysis and interpretation. NIR spectral data are interpreted through means often involving multivariate statistical analysis, sometimes associated with optimisation techniques for model improvement. The objective of this research was to explore the extent to which genetic algorithms (GA) can be used to enhance model development, for predicting fruit quality. Apple fruits were used, and NIR spectra in the range from 12000 to 4000 cm(-1) were acquired on both bruised and healthy tissues, with different degrees of mechanical damage. GAs were used in combination with partial least squares regression methods to develop bruise severity prediction models, and compared to PLS models developed using the full NIR spectrum. A classification model was developed, which clearly separated bruised from unbruised apple tissue. GAs helped improve prediction models by over 10%, in comparison with full spectrum-based models, as evaluated in terms of error of prediction (Root Mean Square Error of Cross-validation). PLS models to predict internal quality, such as sugar content and acidity were developed and compared to the versions optimized by genetic algorithm. Overall, the results highlighted the potential use of GA method to improve speed and accuracy of fruit quality prediction.
更多查看译文
关键词
genetic algorithm,optimization,variable selection,apple bruise,FT-NIR,effective wavelength,model performance
AI 理解论文
溯源树
样例
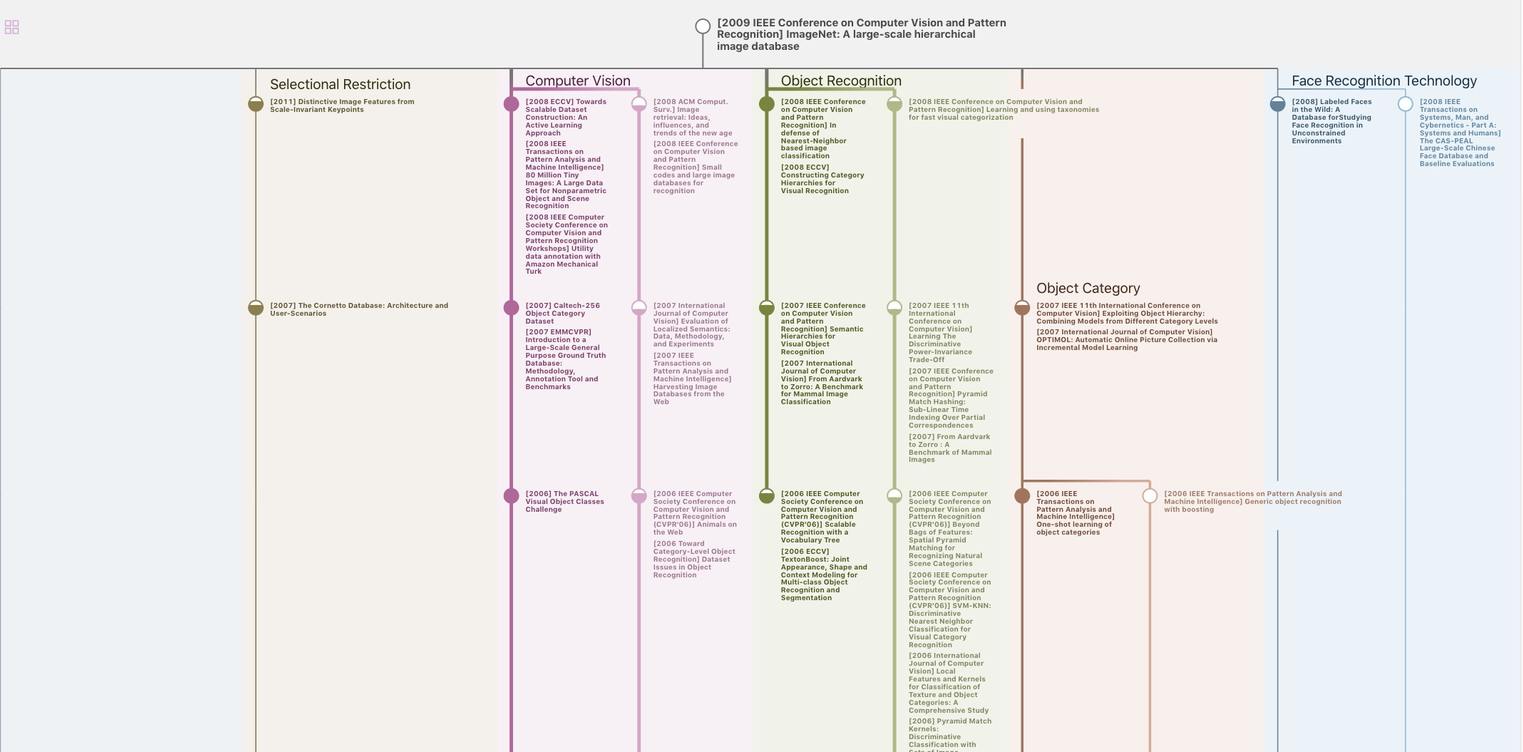
生成溯源树,研究论文发展脉络
Chat Paper
正在生成论文摘要