Untrusted Predictions Improve Trustable Query Policies
arxiv(2021)
摘要
We study how to utilize (possibly machine-learned) predictions in a model for optimization under uncertainty that allows an algorithm to query unknown data. The goal is to minimize the number of queries needed to solve the problem. Considering fundamental problems such as finding the minima of intersecting sets of elements or sorting them, as well as the minimum spanning tree problem, we discuss different measures for the prediction accuracy and design algorithms with performance guarantees that improve with the accuracy of predictions and that are robust with respect to very poor prediction quality. We also provide new structural insights for the minimum spanning tree problem that might be useful in the context of explorable uncertainty regardless of predictions. Our results prove that untrusted predictions can circumvent known lower bounds in the model of explorable uncertainty. We complement our results by experiments that empirically confirm the performance of our algorithms.
更多查看译文
关键词
trustable query policies,predictions
AI 理解论文
溯源树
样例
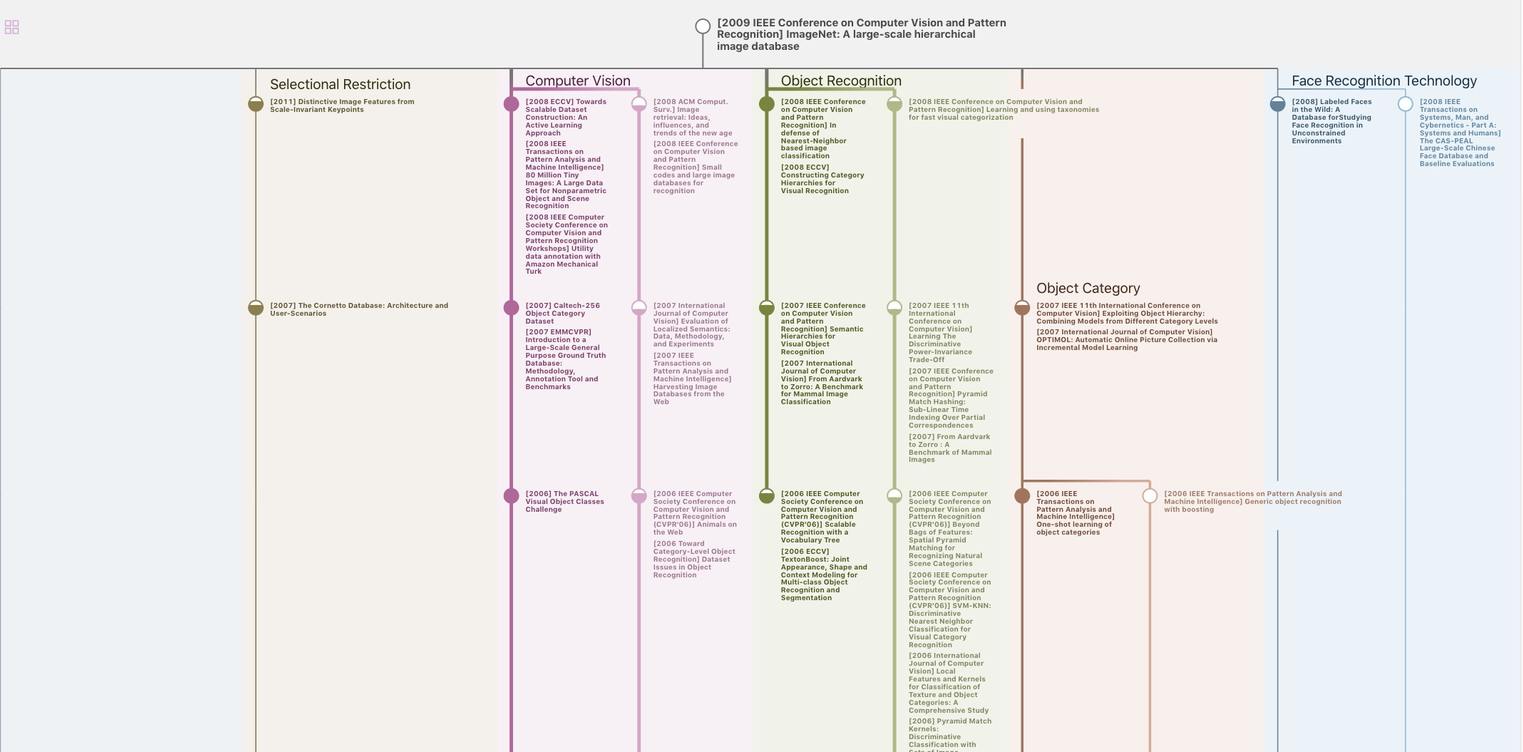
生成溯源树,研究论文发展脉络
Chat Paper
正在生成论文摘要