Pose-Dependent Weights and Domain Randomization for Fully Automatic X-Ray to CT Registration
IEEE Transactions on Medical Imaging(2021)
摘要
Fully automatic X-ray to CT registration requires a solid initialization to provide an initial alignment within the capture range of existing intensity-based registrations. This work addresses that need by providing a novel automatic initialization, which enables end to end registration. First, a neural network is trained once to detect a set of anatomical landmarks on simulated X-rays. A domain randomization scheme is proposed to enable the network to overcome the challenge of being trained purely on simulated data and run inference on real X-rays. Then, for each patient CT, a fully-automatic patient-specific landmark extraction scheme is used. It is based on backprojecting and clustering the previously trained network's predictions on a set of simulated X-rays. Next, the network is retrained to detect the new landmarks. Finally the combination of network and 3D landmark locations is used to compute the initialization using a perspective-n-point algorithm. During the computation of the pose, a weighting scheme is introduced to incorporate the confidence of the network in detecting the landmarks. The algorithm is evaluated on the pelvis using both real and simulated x-rays. The mean (± standard deviation) target registration error in millimetres is 4.1 ± 4.3 for simulated X-rays with a success rate of 92% and 4.2 ± 3.9 for real X-rays with a success rate of 86.8%, where a success is defined as a translation error of less than 30
mm
.
更多查看译文
关键词
Algorithms,Humans,Imaging, Three-Dimensional,Radiography,Random Allocation,Tomography, X-Ray Computed,X-Rays
AI 理解论文
溯源树
样例
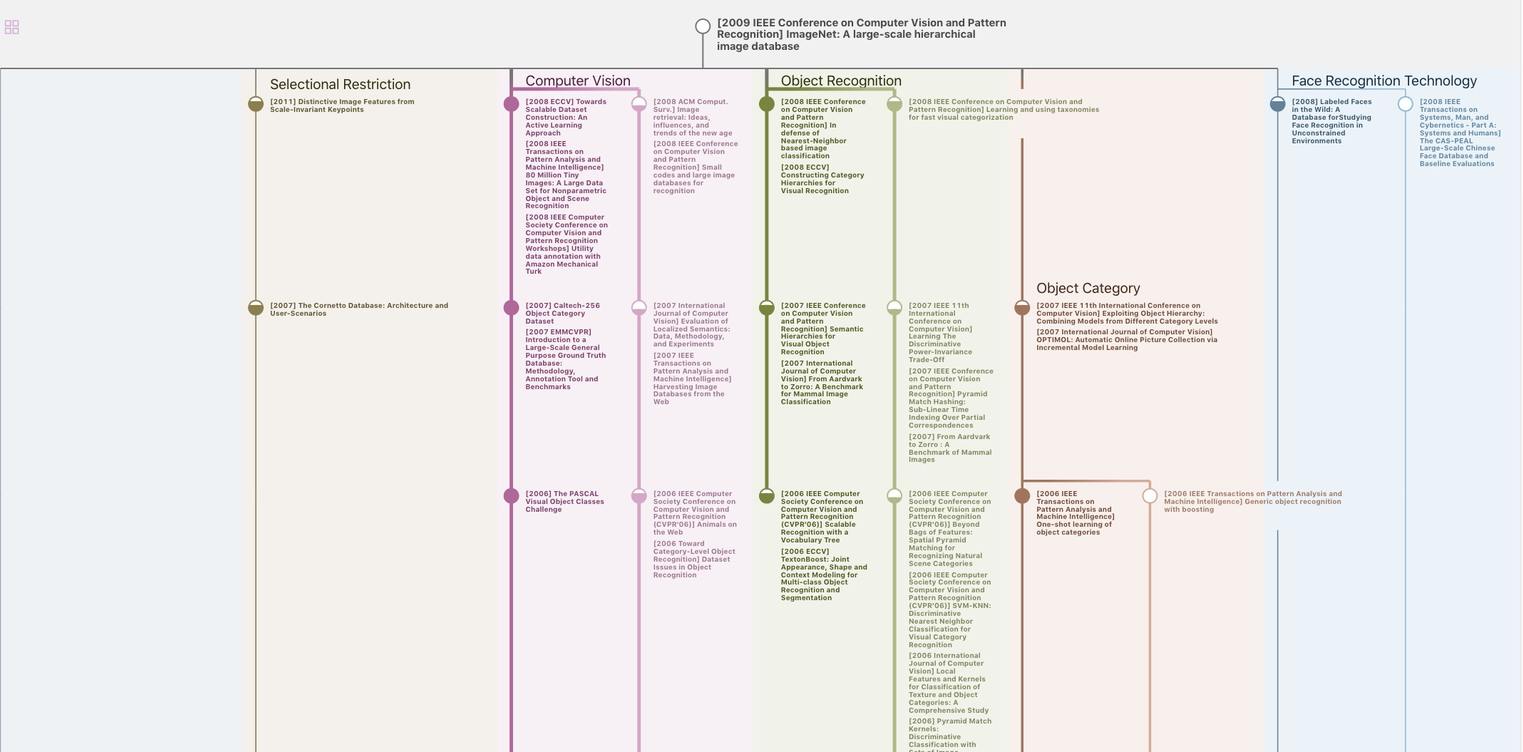
生成溯源树,研究论文发展脉络
Chat Paper
正在生成论文摘要