A structural model for contextual code changes
Proceedings of the ACM on Programming Languages(2020)
摘要
We address the problem of predicting edit completions based on a learned model that was trained on past edits. Given a code snippet that is partially edited, our goal is to predict a completion of the edit for the rest of the snippet. We refer to this task as the EditCompletion task and present a novel approach for tackling it. The main idea is to directly represent structural edits. This allows us to model the likelihood of the edit itself, rather than learning the likelihood of the edited code. We represent an edit operation as a path in the program’s Abstract Syntax Tree (AST), originating from the source of the edit to the target of the edit. Using this representation, we present a powerful and lightweight neural model for the EditCompletion task.
We conduct a thorough evaluation, comparing our approach to a variety of representation and modeling approaches that are driven by multiple strong models such as LSTMs, Transformers, and neural CRFs. Our experiments show that our model achieves a 28% relative gain over state-of-the-art sequential models and 2× higher accuracy than syntactic models that learn to generate the edited code, as opposed to modeling the edits directly.
Our code, dataset, and trained models are publicly available at https://github.com/tech-srl/c3po/ .
更多查看译文
关键词
Edit Completions,Machine Learning,Neural Models of Code
AI 理解论文
溯源树
样例
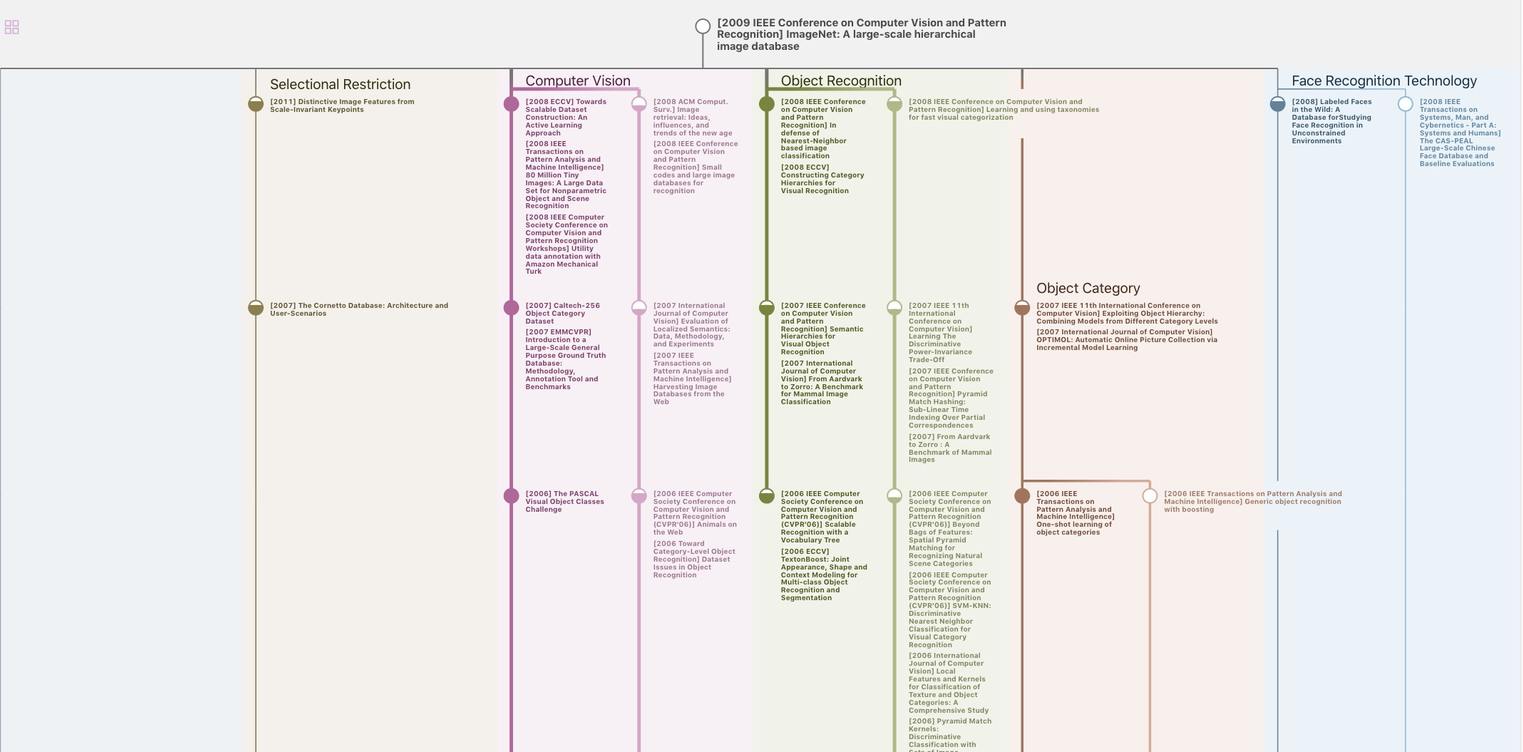
生成溯源树,研究论文发展脉络
Chat Paper
正在生成论文摘要