Broad Graph-based Non-negative Robust Continuous Clustering
IEEE ACCESS(2020)
摘要
Recently, the robust continuous clustering (RCC) has been proposed for unsupervised data classification. The RCC algorithm integrates representation learning and clustering by seeking the balance of the distance of data between intra-cluster and inter-cluster. But the inter-cluster distance in RCC highly depend on the pairwise graph of neighbors, which are constructed by the less-expressive original data. This hampers the performance of RCC on complex epically high-dimensional data. Encouraged by the hybrid feature learning and universal approximation capabilities of the broad learning system (BLS), we first propose a broad graph-based robust continuous clustering algorithm to upgrade RCC. The proposed algorithm measures the distance of the pairwise data with the feature learned from the BLS when constructing the graph. Then to further enhance the clustering performance of the RCC on high-dimensional data, we embed the non-negative matrix factorization (NMF) into the broad graph-based RCC algorithm. By resolving the original data into the basis matrix and coefficient matrix and performing the broad graph-based RCC on the coefficient matrix, the proposed approach takes full advantages of the abundant representation from the BLS and the sparsity and interpretability of NMF coefficients. We verified the proposed algorithms on the synthetic dataset, the UCI dataset and some real-world high dimension datasets. All the empirical results show the proposed algorithms outperform the baselines and improve the clustering performance of RCC effectively.
更多查看译文
关键词
Robust continuous clustering,broad learning system,non-negative matrix factorization,representation learning
AI 理解论文
溯源树
样例
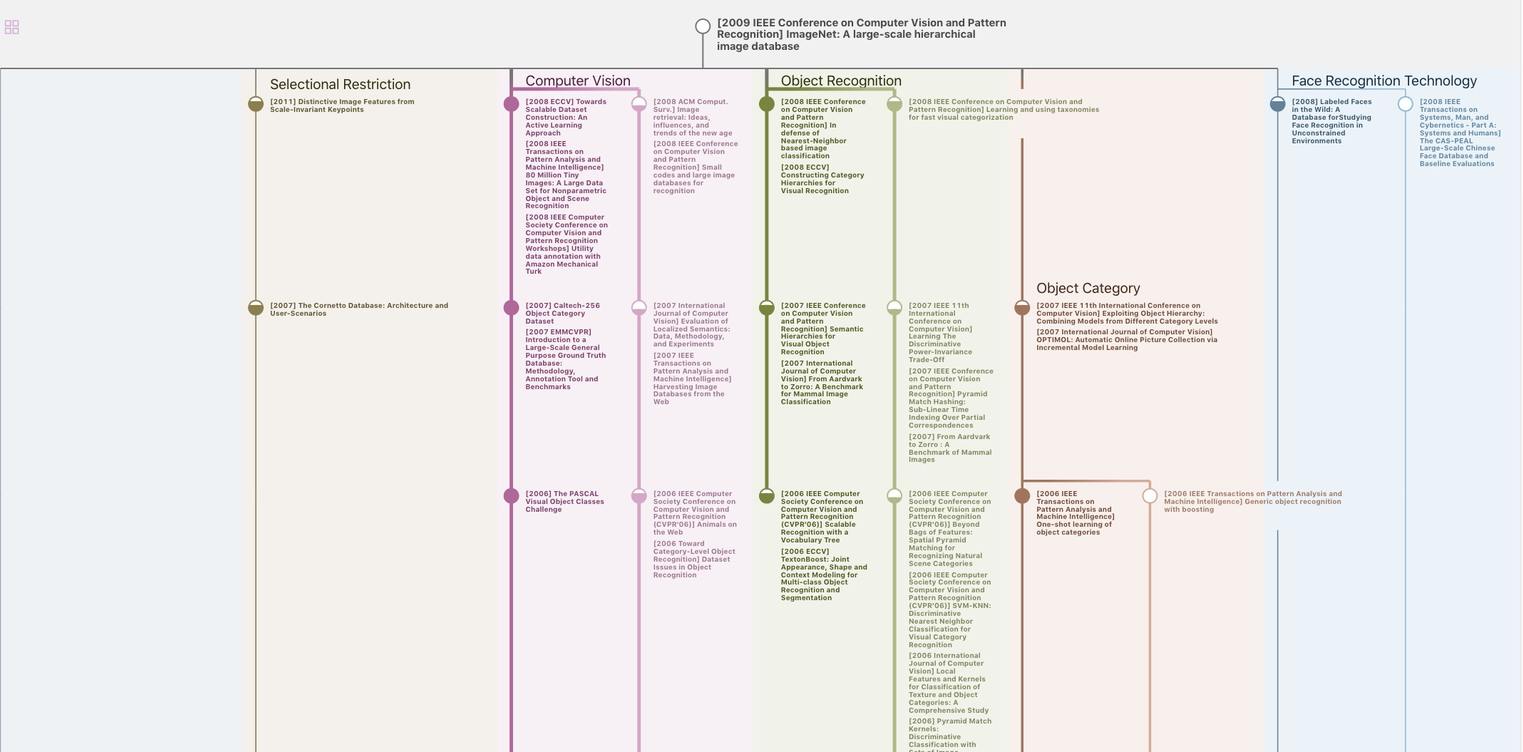
生成溯源树,研究论文发展脉络
Chat Paper
正在生成论文摘要