Laplacian score and genetic algorithm based automatic feature selection for Markov State Models in adaptive sampling based molecular dynamics
PeerJ(2020)
摘要
Adaptive sampling molecular dynamics based on Markov State Models use short parallel MD simulations to accelerate simulations, and are proven to identify hidden conformers. The accuracy of the predictions provided by it depends on the features extracted from the simulated data that is used to construct it. The identification of the most important features in the trajectories of the simulated system has a considerable effect on the results. Methods In this study, we use a combination of Laplacian scoring and genetic algorithms to obtain an optimized feature subset for the construction of the MSM. The approach is validated on simulations of three protein folding complexes, and two protein ligand binding complexes. Results Our experiments show that this approach produces better results when the number of samples is significantly lesser than the number of features extracted. We also observed that this method mitigates over fitting that occurs due to high dimensionality of large biosystems with shorter simulation times.
更多查看译文
关键词
Molecular Dynamics,Markov State Models,Laplacian scores,Computational Chemistry,Accelerated Molecular Dynamics
AI 理解论文
溯源树
样例
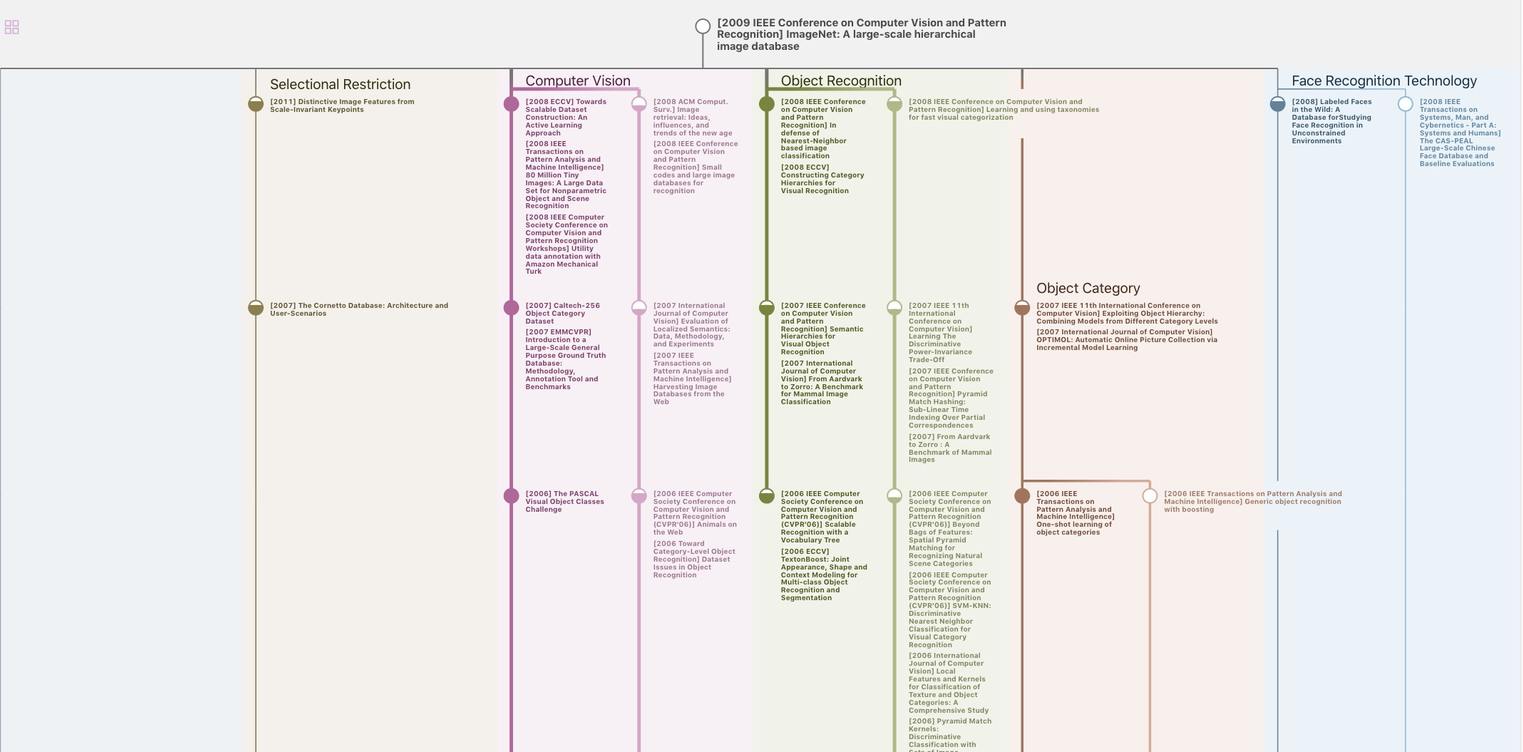
生成溯源树,研究论文发展脉络
Chat Paper
正在生成论文摘要