Predictive modeling, applied to genetically engineered mouse models of breast or lung cancer, provides insights into major oncogenic pathways
Cancer Research(2020)
摘要
The H2020 project CanPathPro is building and validating a computational predictive modelling platform applied to cancer. To this end, we develop and refine bioinformatic and experimental tools, utilized in generation and evaluation of systems biology modeling predictions. The presented work employs the following technologies and methodologies: biologic systems representing 3 levels of biologic complexity (genetically engineered mouse models—GEMMs—of breast or lung cancer, organoids and cell lines derived thereof); next-generation sequencing and SWATH-based phospho/proteomics; and two large-scale computational mechanistic models. The highly defined biologic systems are used (i) to activate selected oncogenic stimuli that modulate pathway components in a systematic manner; (ii) to characterize the signaling changes occurring during cancer development—thus generating temporally resolved datasets for model training; and (iii) to validate, in vitro and in vivo, the modeling predictions. The mechanistic models, based on ordinary differential equations, enable prediction of phenotypes and drug response in mouse or human. Model parameters are defined using project-derived experimental data, either via parameter estimation strategies or via selection of parameter distributions by a Monte Carlo approach. For simulations, the models are initialized with transcriptome data, either from GEMM-derived cell lines grown under variable conditions or from GEMM-derived neoplastic and tumor tissue, representing lesion progression. The models also integrate the relevant mutations. Results, obtained by iterative rounds of in silico predictions and in vitro validation, include in silico identification (i) of the activation status of oncogenic pathways in GEMMs, organoids, and cell lines; (ii) of the signaling changes induced by the in vitro growth conditions (e.g., growth factor modulation, drug treatments) and by the mutational profile of cell lines/organoids, or by the mutational profile and lesion stage of each GEMM; and (iii) of the drug response of cell lines and GEMMs. In conclusion, this work encompasses a highly integrative systems biology approach generating and validating new hypotheses on cancer pathways signaling and crosstalk, identifying new signal flow, and suggests new ways to interfere with tumor growth. This abstract is also being presented as Poster B49. Citation Format: Julio Banga, Lucien Frappart, Jan Hasenauer, Yann Herault, Jos Jonkers, David Koubi, Bodo Lange, Glenn Terje Lines, Aspasia Plouidou, Oliver Rinner. Predictive modeling, applied to genetically engineered mouse models of breast or lung cancer, provides insights into major oncogenic pathways [abstract]. In: Proceedings of the AACR Special Conference on the Evolving Landscape of Cancer Modeling; 2020 Mar 2-5; San Diego, CA. Philadelphia (PA): AACR; Cancer Res 2020;80(11 Suppl):Abstract nr PHB04.
更多查看译文
AI 理解论文
溯源树
样例
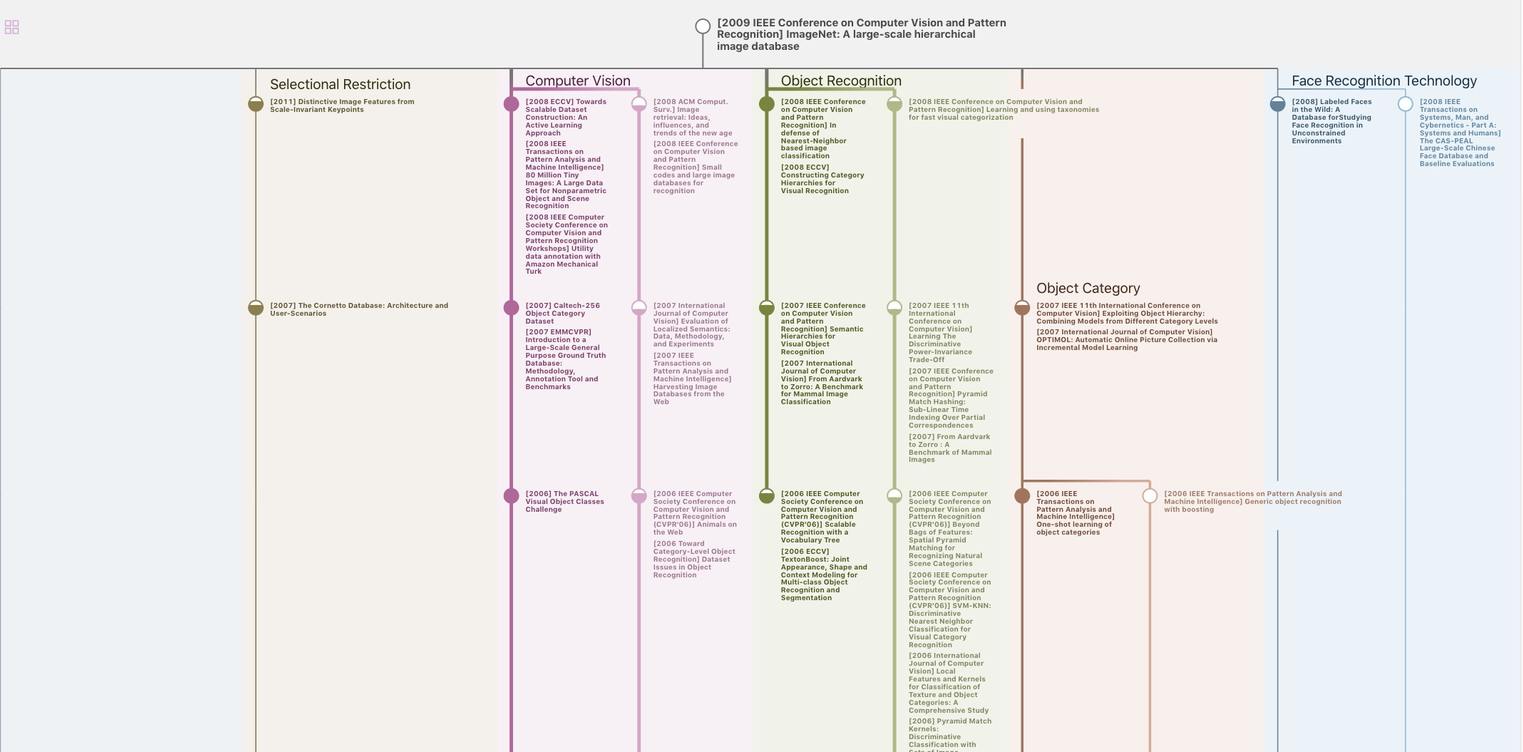
生成溯源树,研究论文发展脉络
Chat Paper
正在生成论文摘要