Learning Features From Georeferenced Seafloor Imagery With Location Guided Autoencoders
JOURNAL OF FIELD ROBOTICS(2021)
摘要
Although modern machine learning has the potential to greatly speed up the interpretation of imagery, the varied nature of the seabed and limited availability of expert annotations form barriers to its widespread use in seafloor mapping applications. This motivates research into unsupervised methods that function without large databases of human annotations. This paper develops an unsupervised feature learning method for georeferenced seafloor visual imagery that considers patterns both within the footprint of a single image frame and broader scale spatial characteristics. Features within images are learnt using an autoencoder developed based on the AlexNet deep convolutional neural network. Features larger than each image frame are learnt using a novel loss function that regularises autoencoder training using the Kullback-Leibler divergence function to loosely assume that images captured within a close distance of each other look more similar than those that are far away. The method is used to semantically interpret images taken by an autonomous underwater vehicle at the Southern Hydrates Ridge, an active gas hydrate field and site of a seafloor cabled observatory at a depth of 780 m. The method's performance when applied to clustering and content-based image retrieval is assessed against a ground truth consisting of more than 18,000 human annotations. The study shows that the location based loss function increases the rate of information retrieval by a factor of two for seafloor mapping applications. The effects of physics-based colour correction and image rescaling are also investigated, showing that the improved consistency of spatial information achieved by rescaling is beneficial for recognising artificial objects such as cables and infrastructures, but is less effective for natural objects that have greater dimensional variability.
更多查看译文
关键词
autoencoder, computer vision, mapping, underwater robotics, unsupervised learning
AI 理解论文
溯源树
样例
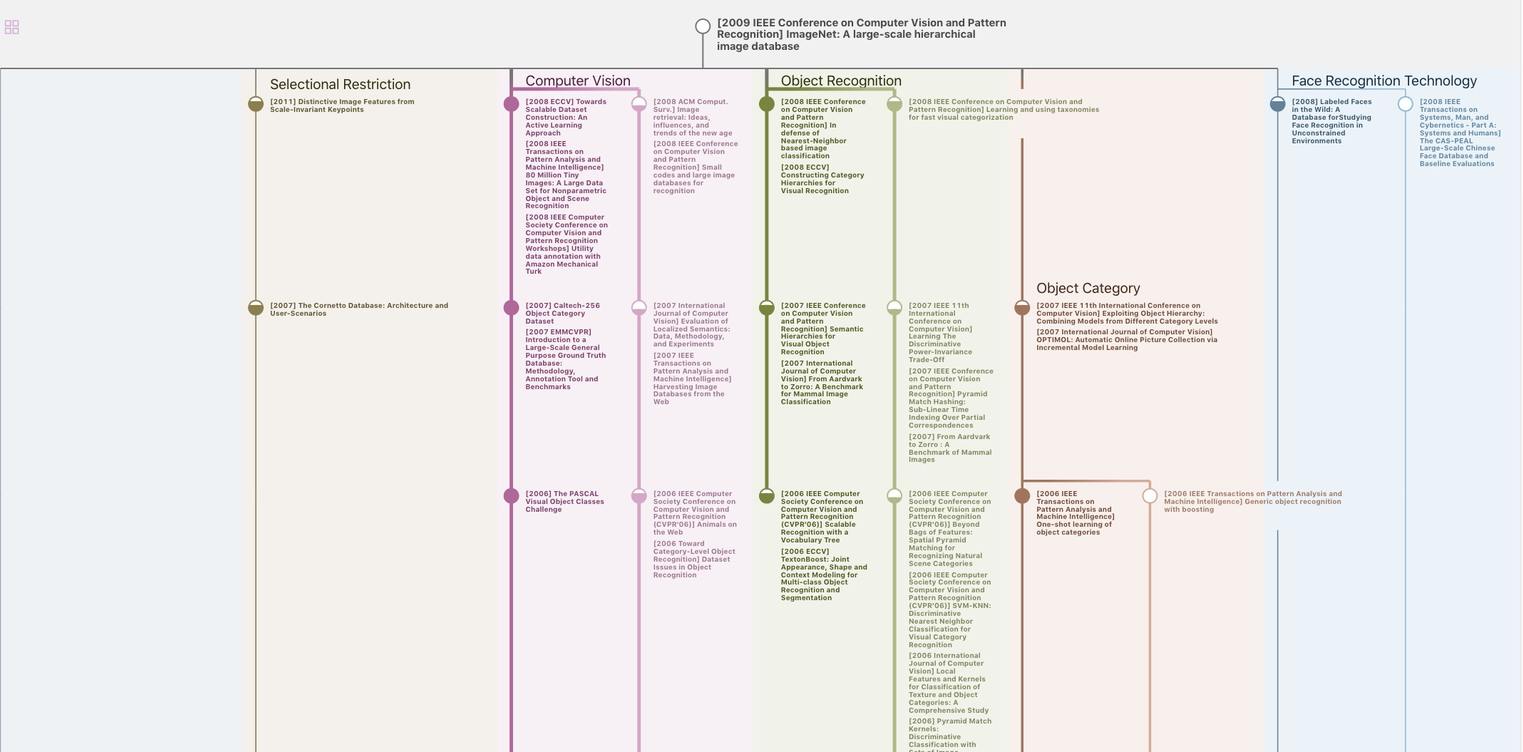
生成溯源树,研究论文发展脉络
Chat Paper
正在生成论文摘要