Neural networks for estimating physical parameters in membrane distillation
Journal of Membrane Science(2020)
摘要
Membrane permeability and heat transfer rate in membrane distillation (MD) are approximated using physically incomplete empirical methods and imperfectly collected experimental data. This work develops a method for assessing the accuracy of these empirical methods as a function of the set of included parameters and the error in those parameter measurements. We train Neural Networks (NNs) on either process parameters (i.e. permeate flux, module design, temperatures, etc.) or both process parameters and membrane parameters (i.e., membrane porosity, membrane thermal conductivity, and pore size) from simulated MD datasets. We then compare NN predictions of membrane permeability, heat transfer rate, and membrane thermal conductivity to experimental data and a test set of simulated data with and without random error. NNs that use both process and membrane parameters have median errors, on average, 3 times lower than NNs using only process parameters and 20 times lower than Schofield and Modified-Schofield empirical methods. This work presents the first assessment of the accuracy of empirical methods for physical parameter estimation in MD and demonstrates the value of NNs in guiding the derivation of new empirical methods by identifying critical input parameters and the required measurement accuracy of those parameters.
更多查看译文
关键词
Membrane characterization,Neural networks,Machine learning,Heat transfer,Membrane distillation
AI 理解论文
溯源树
样例
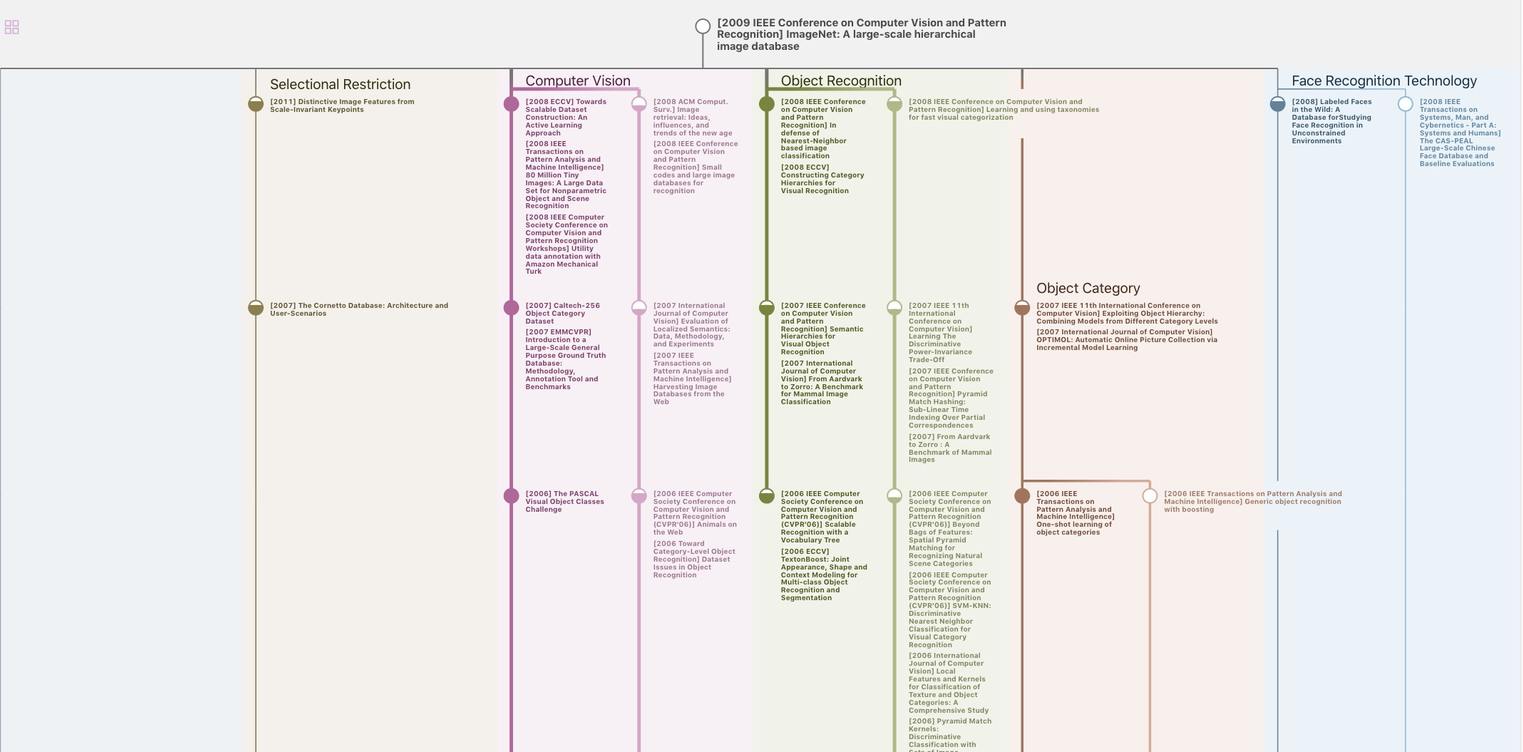
生成溯源树,研究论文发展脉络
Chat Paper
正在生成论文摘要