Image Denoising With Deep Convolutional Neural and Multi-Directional Long Short-Term Memory Networks Under Poisson Noise Environments
IEEE ACCESS(2020)
摘要
Removal Poisson noise poses a very challenging technical issue because it is difficult to capture noise characteristics. This induces from the fact that Poisson noises from different sources affect each image pixel proportional to the pixel level. This paper addresses a new image denoising method for removing Poisson noise based on the Deep Convolutional Neural and Multi-directional Long-Short Term Memory Networks. The architecture of the proposed network contains some Convolutional Neural Network (CNN) layers and multi-directional Long-Short Term Memory (LSTM) layers. CNN layers are responsible to extract image features and to estimate some noise bases existed in images. The multi-directional LSTM layers are used to effectively capture and learn the statistics of residual noise components, which possess long-range correlations and appear sparse in the spatial domain. Moreover, designing deep learning models for image denoising involves several hyperparameters such as a number of layers. To select proper hyperparameters, it is beneficial to investigate what is the best image denoising performance we can achieve under different model complexities. Moreover knowing and realizing how far the employing image denoising algorithm can do to the optimal result makes us possible to design the efficient image denoising algorithm. We utilize the Blahut-Arimoto algorithm to derive numerically distortion-mutual information function of image denoising algorithm. The derived function serves as the distortion lower bound given the mutual information between the original image and the denoised image. Based on the knowledge of distortion-mutual information function, we can decide how deep the CNN layers should be deployed in our image denoising algorithm before applying the multi-directional LSTM layers. From our experiments, the proposed image denoising algorithm can outperform other algorithms in both subjective and objective qualities.
更多查看译文
关键词
Poisson noise,deep learning,convolutional neural network,multi-directional LSTM network,distortion-mutual information function
AI 理解论文
溯源树
样例
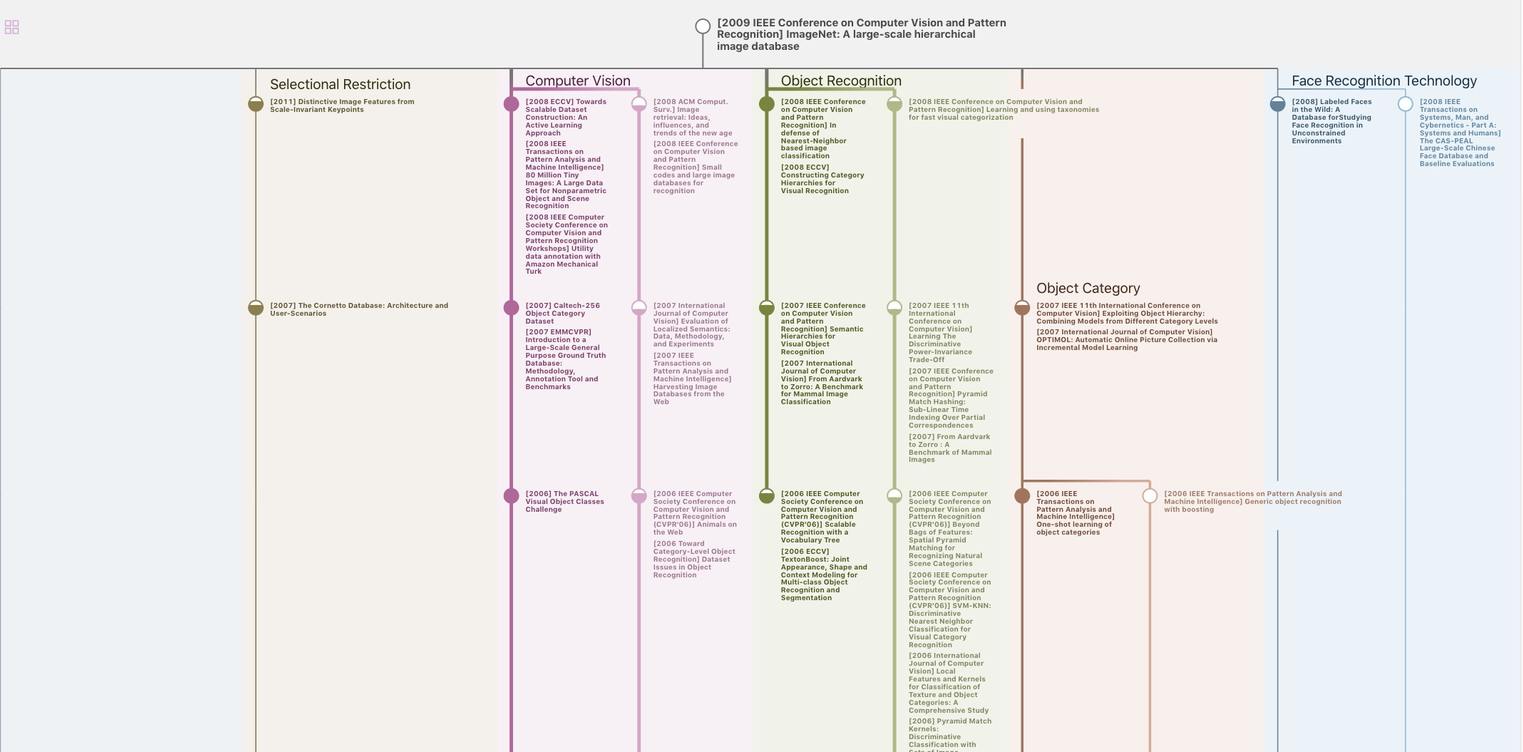
生成溯源树,研究论文发展脉络
Chat Paper
正在生成论文摘要