Deep Learning-Based Illumination Estimation Using Light Source Classification
IEEE ACCESS(2020)
摘要
Color constancy is one of the key steps in the process of image formation in digital cameras. Its goal is to process the image so that there is no influence of illumination color on the colors of objects and surfaces. To capture the target scene colors as accurately as possible, it is crucial to estimate the illumination vector with high accuracy. Unfortunately, the illumination estimation is an ill-posed problem, and solving it most often relies on assumptions. To date, various assumptions have been proposed, which resulted in a wide variety of illumination estimation methods. Statistics-based methods have shown to be appropriate for hardware implementation, but learning-based methods achieve state-of-the-art results, especially those that use deep neural networks. The large learning capacities and generalization abilities of deep neural networks can be used to develop the illumination estimation methods, which are more general and precise. This approach avoids introducing many new assumptions, which often only work in some specific situations. In this paper, a new method for illumination estimation based on light source classification is proposed. In the first step, the set of possible illuminations is reduced by classifying the input image in one of three classes. The classes include images captured in outdoor scenes under natural illuminations, images captured in outdoor scenes under artificial illuminations, and images captured in indoor scenes under artificial illuminations. In the second step, a deep illumination estimation network, which is trained exclusively on images in the class that was predicted in the first step, is applied to the input image. Dividing the illumination space into smaller regions makes the training of illumination estimation networks simpler because the distribution of image scenes and illuminations is less diverse. The experiments on the Cube & x002B; image dataset have shown the median illumination estimation error of 1.27 & x00B0;, which is an improvement of more than 25 & x0025; compared to the use of the single network for all illuminations.
更多查看译文
关键词
Color constancy,illumination estimation,classification,deep learning,white balancing,image enhancement
AI 理解论文
溯源树
样例
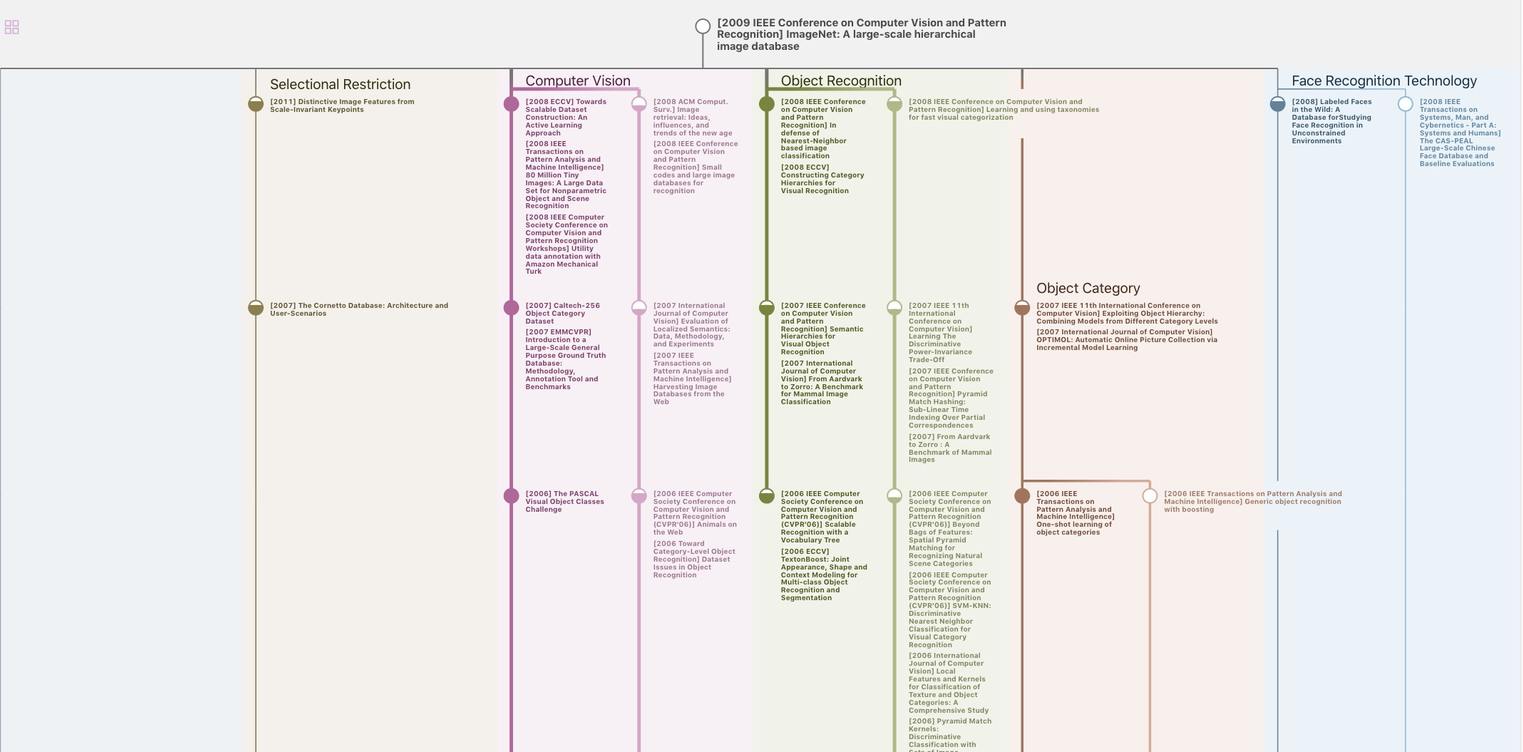
生成溯源树,研究论文发展脉络
Chat Paper
正在生成论文摘要