Selective Spatio-Temporal Aggregation Based Pose Refinement System: Towards Understanding Human Activities In Real-World Videos
2021 IEEE WINTER CONFERENCE ON APPLICATIONS OF COMPUTER VISION WACV 2021(2021)
摘要
Taking advantage of human pose data for understanding human activities has attracted much attention these days. However, state-of-the-art pose estimators struggle in obtaining high-quality 2D or 3D pose data due to occlusion, truncation and low-resolution in real-world un-annotated videos. Hence, in this work, we propose 1) a Selective Spatio-Temporal Aggregation mechanism, named SST-A, that refines and smooths the keypoint locations extracted by multiple expert pose estimators, 2) an effective weakly-supervised self-training framework which leverages the aggregated poses as pseudo ground-truth instead of handcrafted annotations for real-world pose estimation. Extensive experiments are conducted for evaluating not only the upstream pose refinement but also the downstream action recognition performance on four datasets, Toyota Smarthome, NTU-RGB+D, Charades, and Kinetics-50. We demonstrate that the skeleton data refined by our Pose-Refinement system (SSTA-PRS) is effective at boosting various existing action recognition models, which achieves competitive or state-of-the-art performance. Refined pose data is available at: https://github.com/walker-a11y/SSTA-PRS
更多查看译文
关键词
3D pose data,real-world un-annotated videos,Selective Spatio-Temporal Aggregation mechanism,refines,smooths,weakly-supervised self-training framework,aggregated poses,real-world pose estimation,upstream pose refinement,skeleton data,Pose-Refinement system,Pose Refinement system,towards understanding human activities,real-world videos,estimators struggle,high-quality 2D
AI 理解论文
溯源树
样例
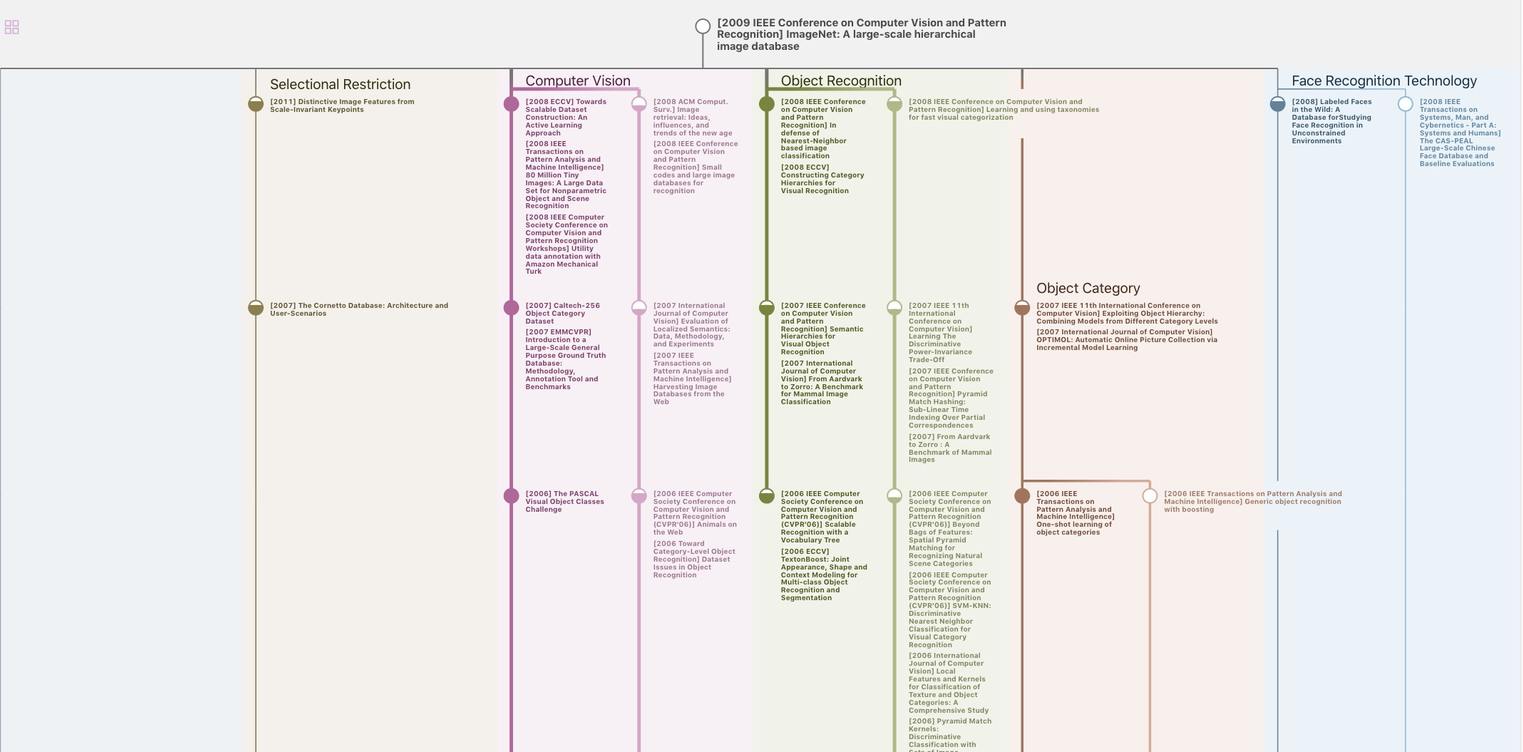
生成溯源树,研究论文发展脉络
Chat Paper
正在生成论文摘要