Learning and Evaluating Representations for Deep One-class Classification
ICLR(2021)
摘要
We present a two-stage framework for deep one-class classification. We first learn self-supervised representations from one-class data, and then build classifiers using generative or discriminative models on learned representations. In particular, we present a novel distribution-augmented contrastive learning method that extends training distributions via data augmentation to obstruct the uniformity of vanilla contrastive representations, yielding more suitable representations for one-class classification. Moreover, we argue that classifiers inspired by the statistical perspective in generative or discriminative models are more effective than existing approaches, such as an average of normality scores from a surrogate classifier. In experiments, we demonstrate state-of-the-art performance on visual domain one-class classification benchmarks. The framework does not only learn a better representation, but it also permits building one-class classifiers that are more faithful to the target task. Finally, we present visual explanations, confirming that the decision-making process of our deep one-class classifier is intuitive to humans.
更多查看译文
关键词
evaluating representations,classification,learning,one-class
AI 理解论文
溯源树
样例
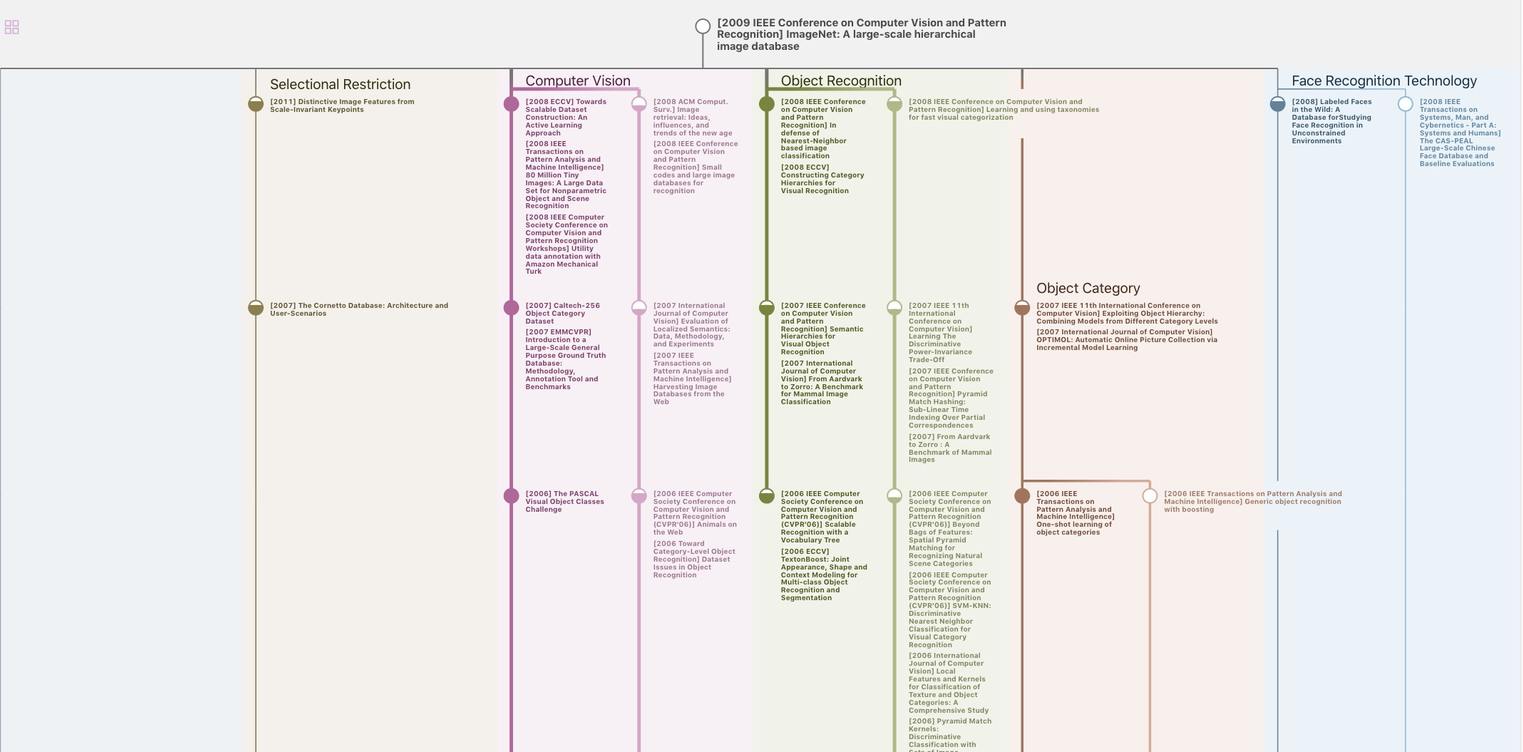
生成溯源树,研究论文发展脉络
Chat Paper
正在生成论文摘要