c-lasso -- a Python package for constrained sparse and robust regression and classification
Journal of Open Source Software(2021)
摘要
We introduce c-lasso, a Python package that enables sparse and robust linear regression and classification with linear equality constraints. The underlying statistical forward model is assumed to be of the following form: \[ y = X \beta + \sigma \epsilon \qquad \textrm{subject to} \qquad C\beta=0 \] Here, $X \in \mathbb{R}^{n\times d}$is a given design matrix and the vector $y \in \mathbb{R}^{n}$ is a continuous or binary response vector. The matrix $C$ is a general constraint matrix. The vector $\beta \in \mathbb{R}^{d}$ contains the unknown coefficients and $\sigma$ an unknown scale. Prominent use cases are (sparse) log-contrast regression with compositional data $X$, requiring the constraint $1_d^T \beta = 0$ (Aitchion and Bacon-Shone 1984) and the Generalized Lasso which is a special case of the described problem (see, e.g, (James, Paulson, and Rusmevichientong 2020), Example 3). The c-lasso package provides estimators for inferring unknown coefficients and scale (i.e., perspective M-estimators (Combettes and M\"uller 2020a)) of the form \[ \min_{\beta \in \mathbb{R}^d, \sigma \in \mathbb{R}_{0}} f\left(X\beta - y,{\sigma} \right) + \lambda \left\lVert \beta\right\rVert_1 \qquad \textrm{subject to} \qquad C\beta = 0 \] for several convex loss functions $f(\cdot,\cdot)$. This includes the constrained Lasso, the constrained scaled Lasso, and sparse Huber M-estimators with linear equality constraints.
更多查看译文
关键词
constrained sparse,robust regression,python package,classification,c-lasso
AI 理解论文
溯源树
样例
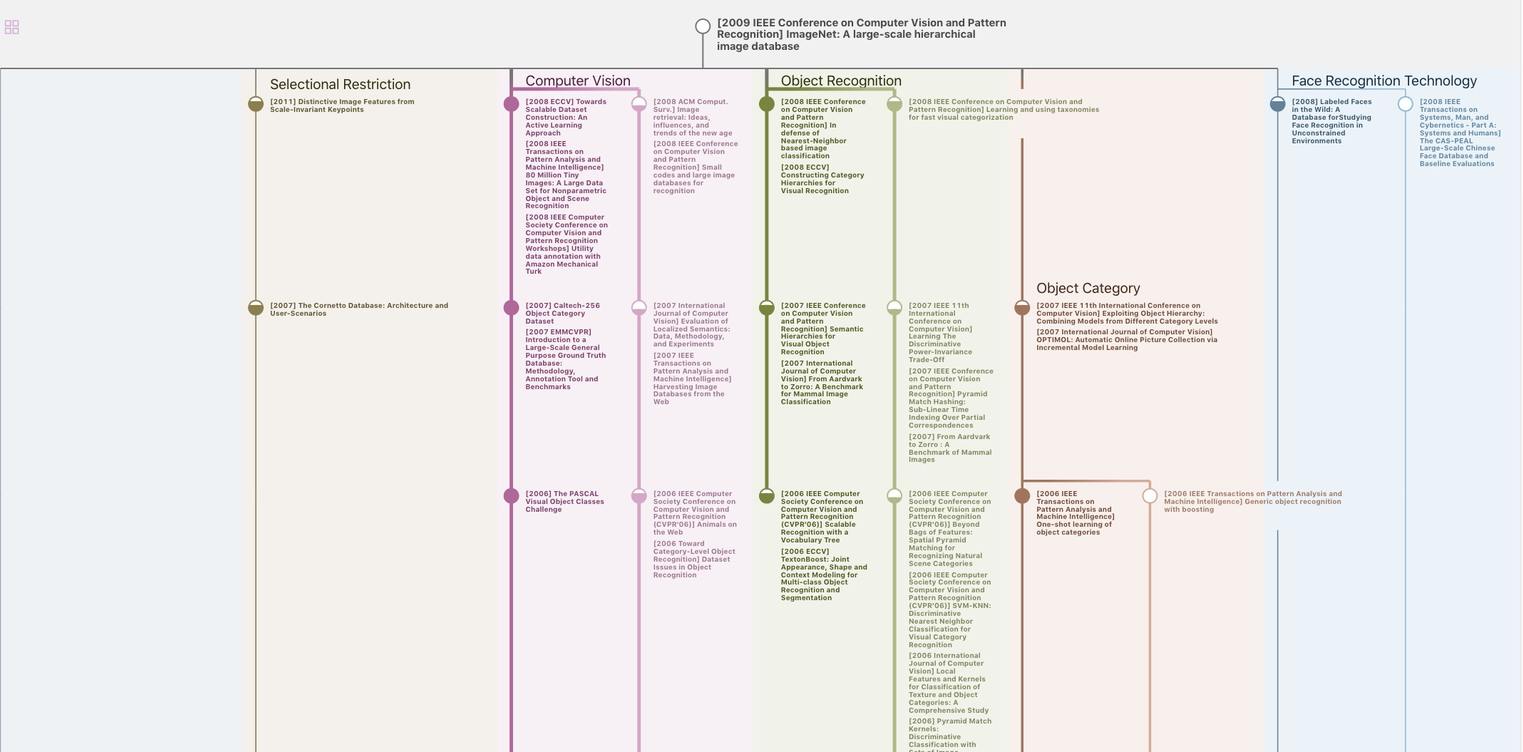
生成溯源树,研究论文发展脉络
Chat Paper
正在生成论文摘要