Comparison Of Deep Learning Synthesis Of Synthetic Cts Using Clinical Mri Inputs
PHYSICS IN MEDICINE AND BIOLOGY(2020)
摘要
There has been substantial interest in developing techniques for synthesizing CT-like images from MRI inputs, with important applications in simultaneous PET/MR and radiotherapy planning. Deep learning has recently shown great potential for solving this problem. The goal of this research was to investigate the capability of four common clinical MRI sequences (T1-weighted gradient-echo [T1], T2-weighted fat-suppressed fast spin-echo [T2-FatSat], post-contrast T1-weighted gradient-echo [T1-Post], and fast spin-echo T2-weighted fluid-attenuated inversion recovery [CUBE-FLAIR]) as inputs into a deep CT synthesis pipeline. Data were obtained retrospectively in 92 subjects who had undergone an MRI and CT scan on the same day. The patient's MR and CT scans were registered to one another using affine registration. The deep learning model was a convolutional neural network encoder-decoder with skip connections similar to the U-net architecture and Inception V3 inspired blocks instead of sequential convolution blocks. After training with 150 epochs and a batch size of 6, the model was evaluated using structural similarity index (SSIM), peak SNR (PSNR), mean absolute error (MAE), and dice coefficient. We found that feasible results were attainable for each image type, and no single image type was superior for all analyses. The MAE (in HU) of the resulting synthesized CT in the whole brain was 51.236 +/- 4.504 for CUBE-FLAIR, 45.432 +/- 8.517 for T1, 44.558 +/- 7.478 for T1-Post, and 45.721 +/- 8.7767 for T2, showing not only feasible, but also very compelling results on clinical images. Deep learning-based synthesis of CT images from MRI is possible with a wide range of inputs, suggesting that viable images can be created from a wide range of clinical input types.
更多查看译文
关键词
deep learning, synthetic CT, PET, MR, radiotherapy MR, attenuation correction
AI 理解论文
溯源树
样例
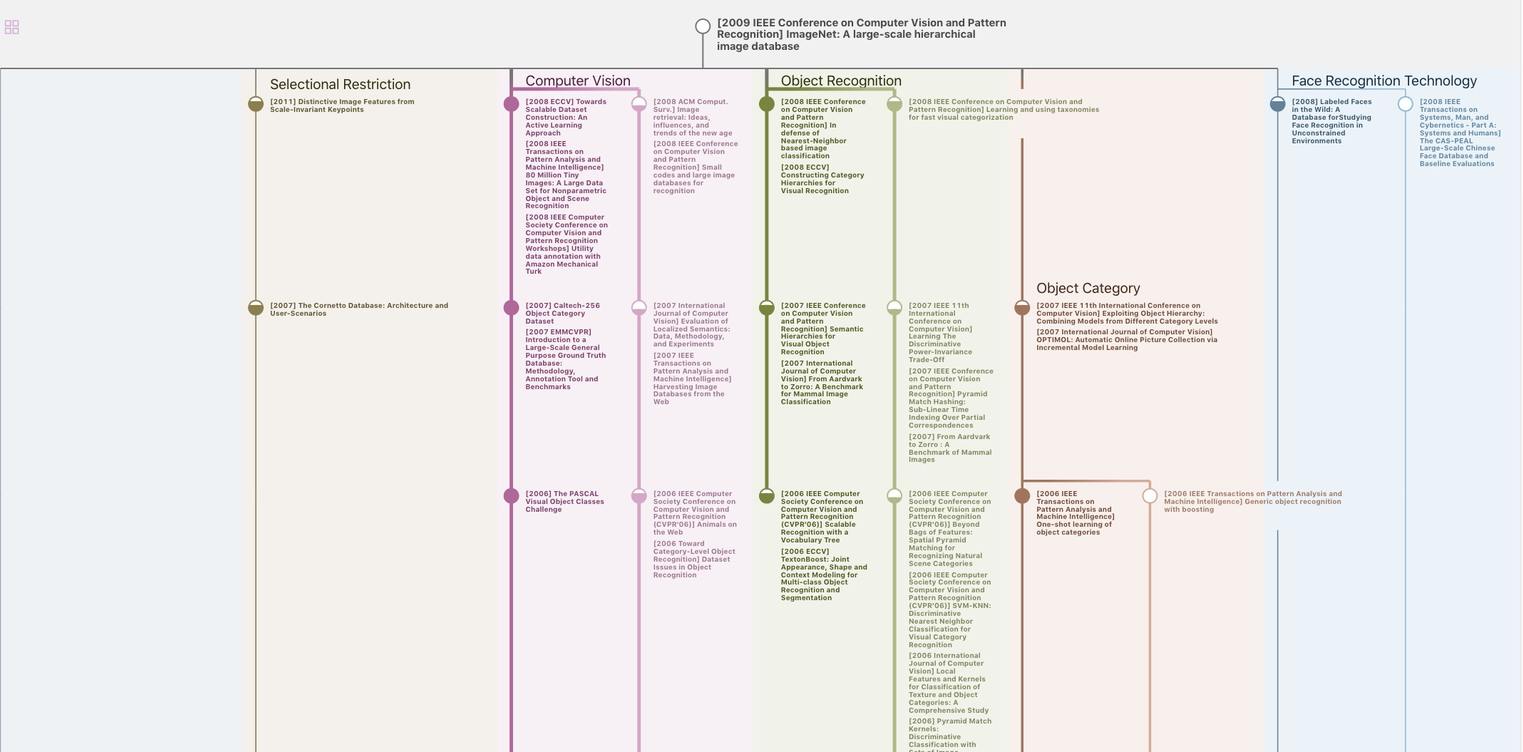
生成溯源树,研究论文发展脉络
Chat Paper
正在生成论文摘要