ACOUSTIC ECHO CANCELLATION WITH THE DUAL-SIGNAL TRANSFORMATION LSTM NETWORK
2021 IEEE INTERNATIONAL CONFERENCE ON ACOUSTICS, SPEECH AND SIGNAL PROCESSING (ICASSP 2021)(2021)
摘要
This paper applies the dual-signal transformation LSTM network (DTLN) to the task of real-time acoustic echo cancellation (AEC). The DTLN combines a short-time Fourier transform and a learned feature representation in a stacked network approach, which enables robust information processing in the time-frequency and in the time domain, which also includes phase information. The model is only trained on 60 h of real and synthetic echo scenarios. The training setup includes multi-lingual speech, data augmentation, additional noise and reverberation to create a model that should generalize well to a large variety of real-world conditions. The DTLN approach produces state-of-the-art performance on clean and noisy echo conditions reducing acoustic echo and additional noise robustly. The method outperforms the AEC-Challenge baseline by 0.30 in terms of Mean Opinion Score (MOS).
更多查看译文
关键词
AEC, real-time, deep learning, audio, voice-communication
AI 理解论文
溯源树
样例
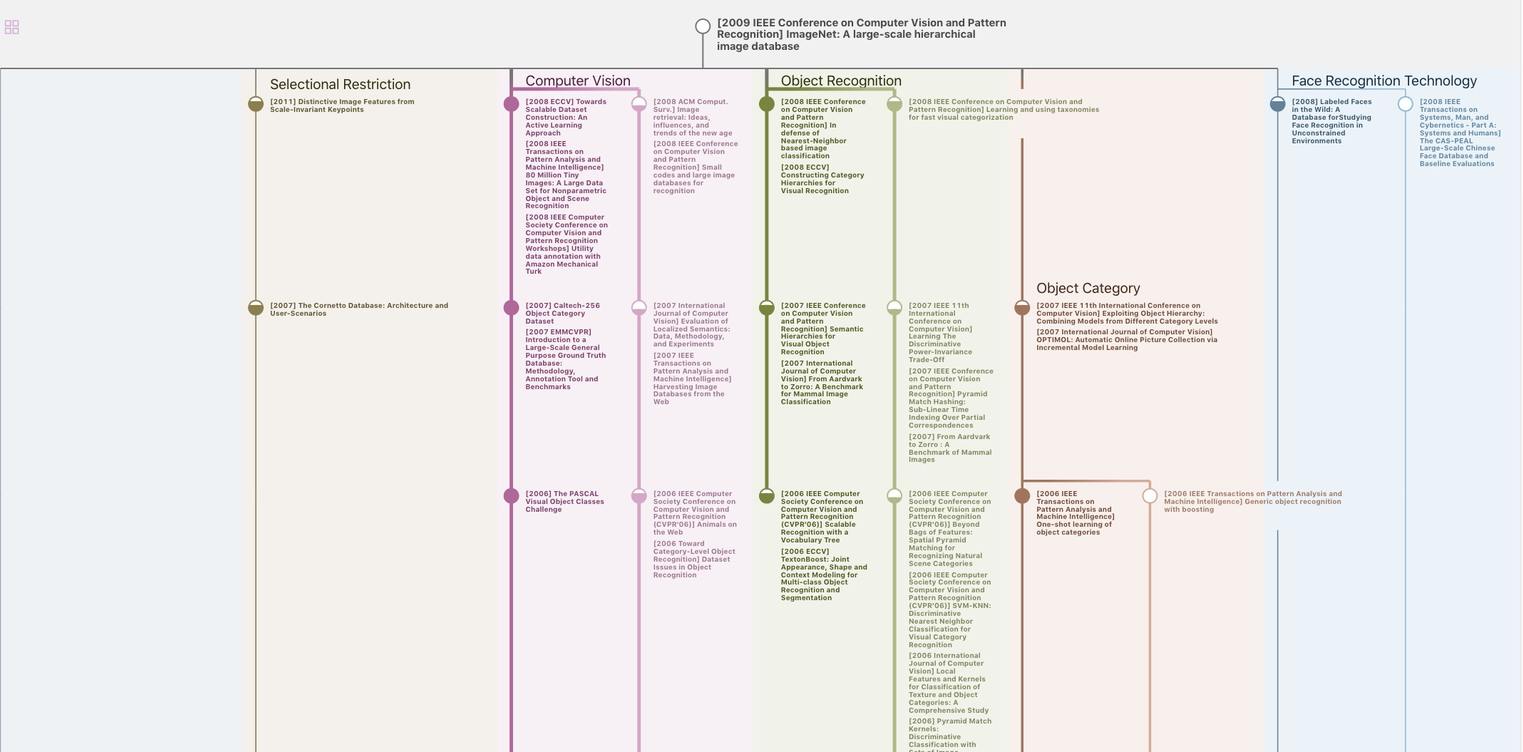
生成溯源树,研究论文发展脉络
Chat Paper
正在生成论文摘要