Interring latent states from foraging behavior
Cognitive Computational Neuroscience (CCN), New York, NY(2017)
摘要
Animal behavior is not driven simply by its current observations, but is strongly influenced by the internal states, which may reflect memories, beliefs, motivation, or arousal. Estimating the structure of these internal states is crucial for understanding the neural basis of behavior. Here we describe a partially observable semi-Markov process to infer latent states directly from observations of behavior. These behaviors are often actions at specific times. In continuous-time Markov processes, the times of transitions between observations are exponentially distributed. The semi-Markov model uses hidden states to describe non-exponential distribution of times between observations, which is a better descriptor of behavior. We test our method on an optimal actor model performing a foraging task, where there are indeed latent states representing internal beliefs that we can identify. Finally, we apply our model to identify latent states in the behaviors of monkeys performing this same foraging task, and find clusters of latent states that identify periods of time consistent with expectant waiting. This behavioral model will be invaluable for defining latent states we can use to measure correspondences between neural activity and the cognitive states that control actions.
更多查看译文
AI 理解论文
溯源树
样例
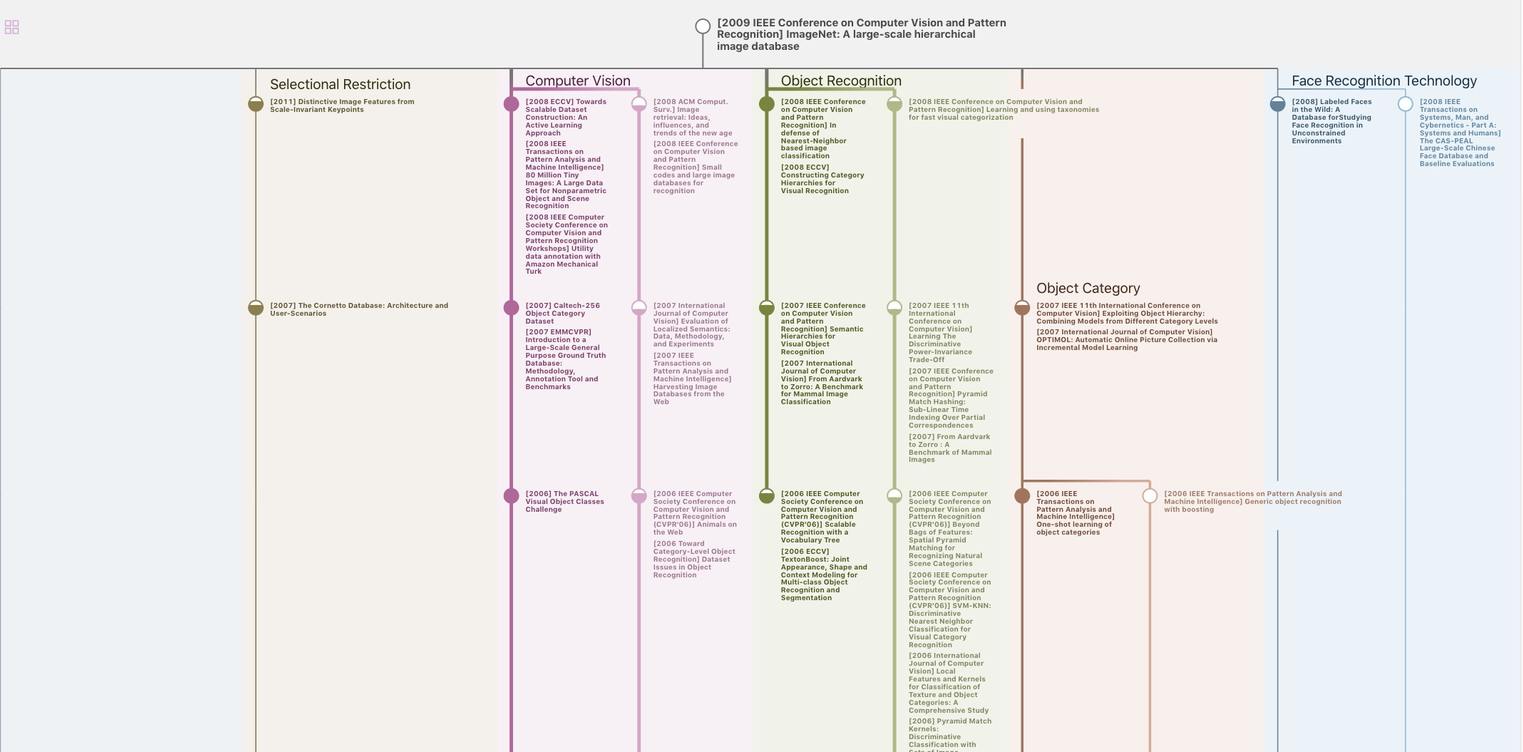
生成溯源树,研究论文发展脉络
Chat Paper
正在生成论文摘要