An Efficient Newton Method for Extreme Similarity Learning with Nonlinear Embeddings
arxiv(2020)
摘要
We study the problem of learning similarity by using nonlinear embedding models (e.g., neural networks) from all possible pairs. This problem is well-known for its difficulty of training with the extreme number of pairs. Existing optimization methods extended from stochastic gradient methods suffer from slow convergence and high complexity per pass of all possible pairs. Inspired by some recent works reporting that Newton methods are competitive for training certain types of neural networks, in this work, we novelly apply the Newton method for this problem. A prohibitive cost depending on the extreme number of pairs occurs if the Newton method is directly applied. We propose an efficient algorithm which successfully eliminates the cost. Our proposed algorithm can take advantage of second-order information and lower time complexity per pass of all possible pairs. Experiments conducted on large-scale data sets demonstrate that the proposed algorithm is more efficient than existing algorithms.
更多查看译文
关键词
extreme similarity learning,nonlinear embeddings,efficient newton method
AI 理解论文
溯源树
样例
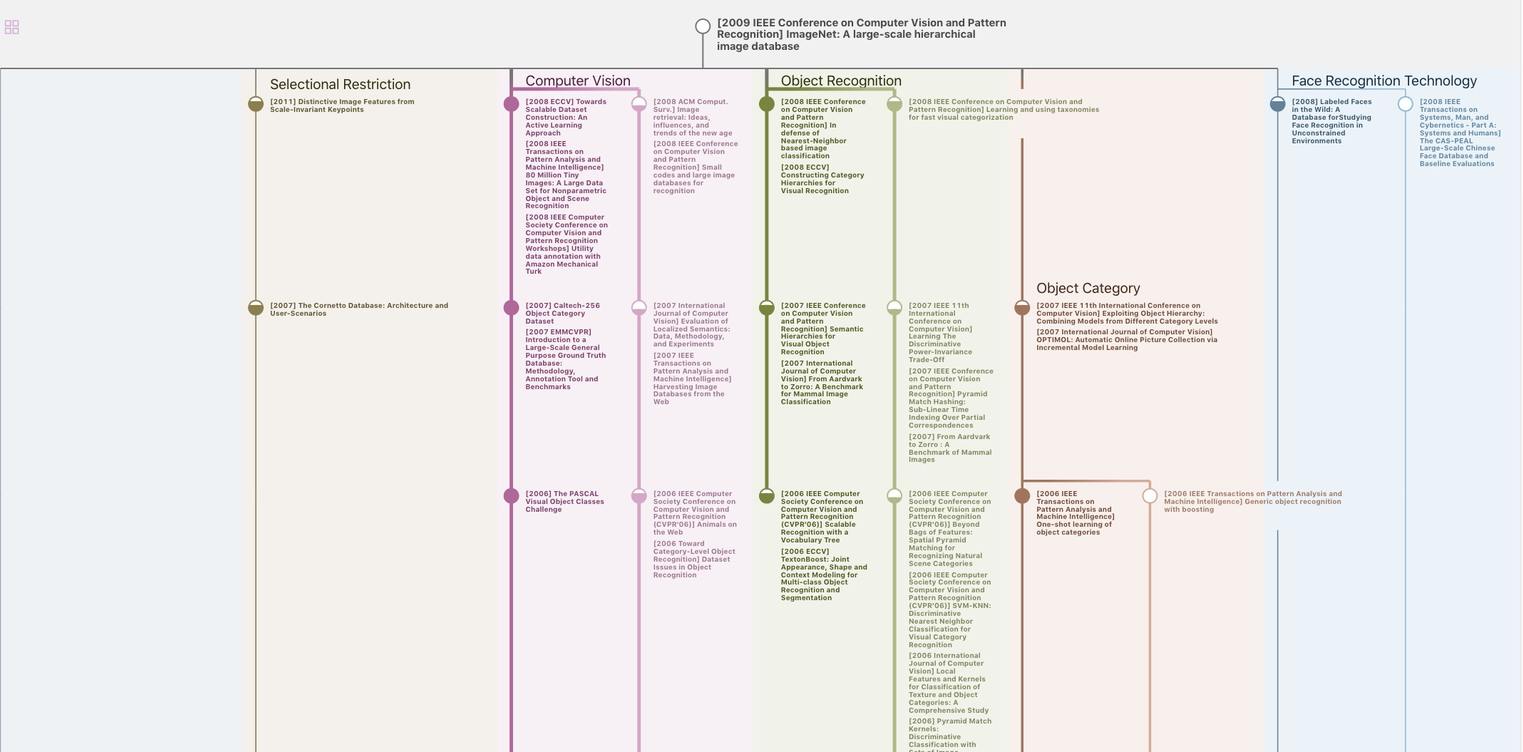
生成溯源树,研究论文发展脉络
Chat Paper
正在生成论文摘要