Uncertainty Quantification For Multiphase-Cfd Simulations Of Bubbly Flows: A Machine Learning-Based Bayesian Approach Supported By High-Resolution Experiments
RELIABILITY ENGINEERING & SYSTEM SAFETY(2021)
摘要
In this paper, we developed a machine learning-based Bayesian approach to inversely quantify and reduce the uncertainties of multiphase computational fluid dynamics (MCFD) simulations for bubbly flows. The proposed approach is supported by high-resolution two-phase flow measurements, including those by doublesensor conductivity probes, high-speed imaging, and particle image velocimetry. Local distributions of key physical quantities of interest (QoIs), including the void fraction and phasic velocities, are obtained to support the Bayesian inference. In the process, the epistemic uncertainties of the closure relations are inversely quantified while the aleatory uncertainties from stochastic fluctuations of the system are evaluated based on experimental uncertainty analysis. The combined uncertainties are then propagated through the MCFD solver to obtain uncertainties of the QoIs, based on which probability-boxes are constructed for validation. The proposed approach relies on three machine learning methods: feedforward neural networks and principal component analysis for surrogate modeling, and Gaussian processes for model form uncertainty modeling. The whole process is implemented within the framework of an open-source deep learning library PyTorch with graphics processing unit (GPU) acceleration, thus ensuring the efficiency of the computation. The results demonstrate that with the support of high-resolution data, the uncertainties of MCFD simulations can be significantly reduced. The proposed approach has the potential for other applications that involve numerical models with empirical parameters.
更多查看译文
关键词
uncertainty quantification,flows,bubbly
AI 理解论文
溯源树
样例
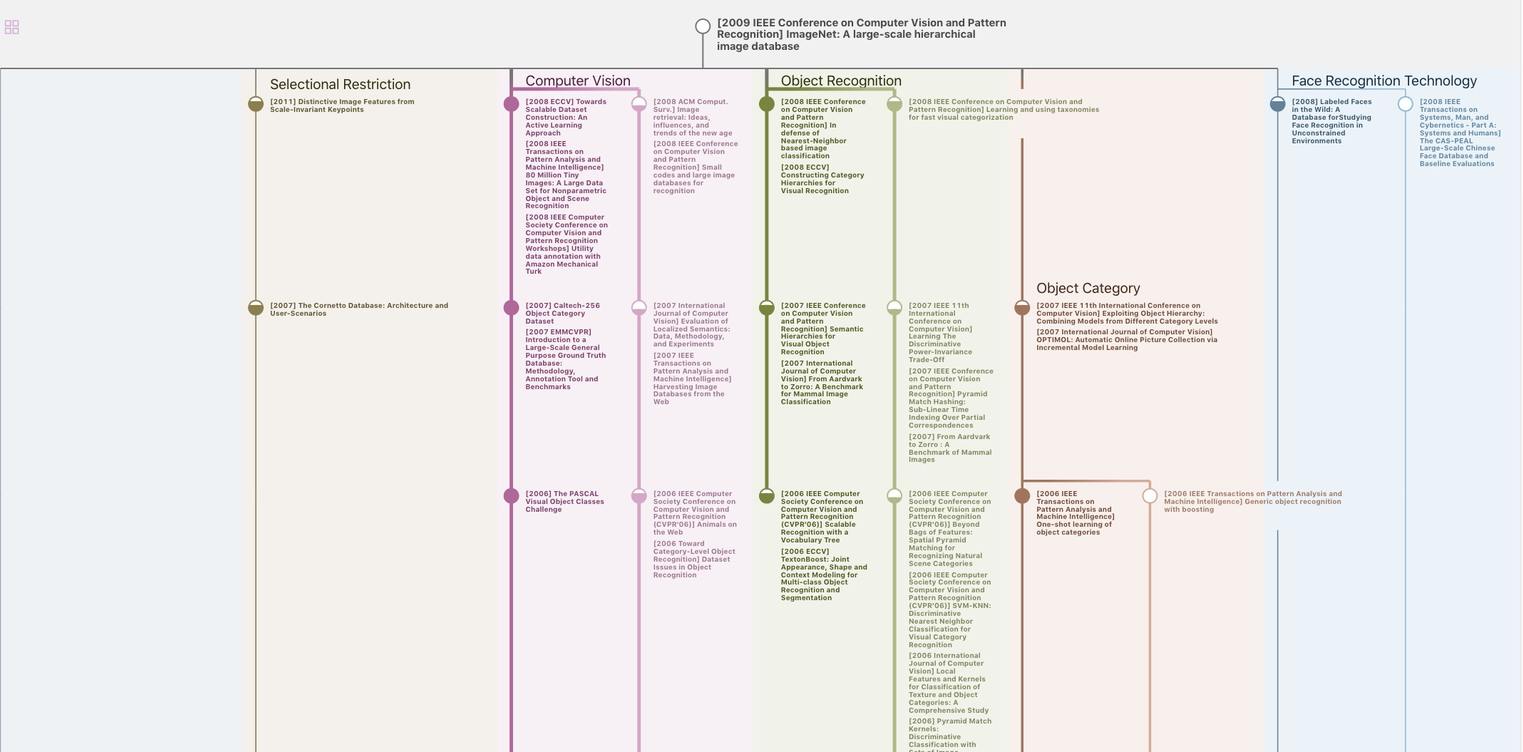
生成溯源树,研究论文发展脉络
Chat Paper
正在生成论文摘要