PREDICTION OF OBJECT GEOMETRY FROM ACOUSTIC SCATTERING USING CONVOLUTIONAL NEURAL NETWORKS
2021 IEEE INTERNATIONAL CONFERENCE ON ACOUSTICS, SPEECH AND SIGNAL PROCESSING (ICASSP 2021)(2021)
摘要
Acoustic scattering is strongly influenced by boundary geometry of objects over which sound scatters. The present work proposes a method to infer object geometry from scattering features by training convolutional neural networks. The training data is generated from a fast numerical solver developed on CUDA. The complete set of simulations is sampled to generate multiple datasets containing different amounts of channels and diverse image resolutions. The robustness of our approach in response to data degradation is evaluated by comparing the performance of networks trained using the datasets with varying levels of data degradation. The present work has found that the predictions made from our models match ground truth with high accuracy. In addition, accuracy does not degrade when fewer data channels or lower resolutions are used.
更多查看译文
关键词
Object geometry prediction, acoustic scattering, convolutional neural network, fast acoustic simulation
AI 理解论文
溯源树
样例
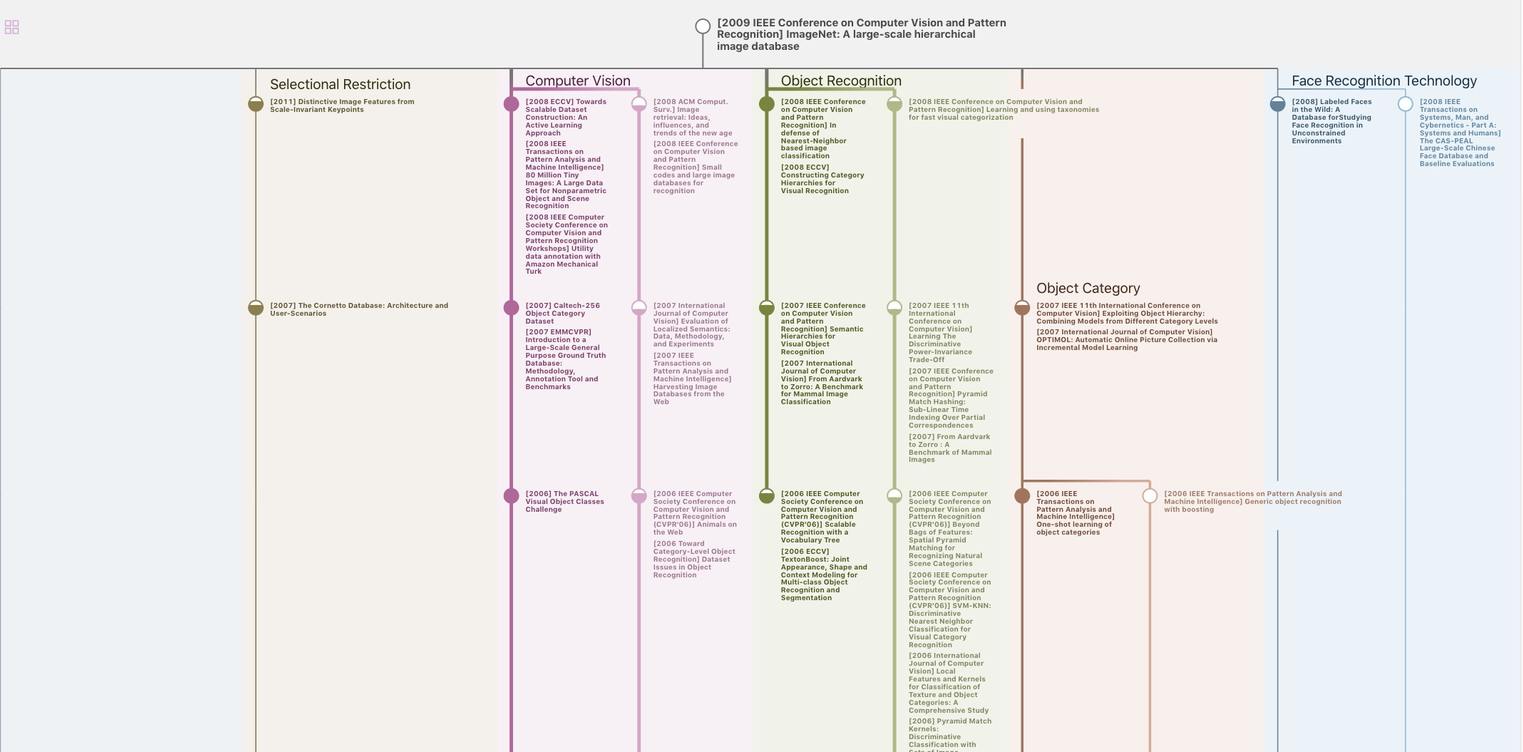
生成溯源树,研究论文发展脉络
Chat Paper
正在生成论文摘要