Robust learning of transport maps with applications to nonlinear filtering
AGUFM(2018)
摘要
We consider the problem of approximating a transport map that pushes forward a limited ensemble from an unknown distribution--possibly non-Gaussian and high-dimensional--to a standard normal distribution. This task is in general non-trivial as the limited sample size imposes severe limitations on the complexity of a transport map that can be learned robustly from data without overfitting. We formalize the bias-variance tradeoff inherent to the computation of the map, and propose novel algorithmic solutions to adaptively discover an``optimal''complexity for the map, and to learn its coefficients. In high dimensions, we regularize the estimation by injecting additional information about the problem, such as the expected smoothness properties of the density or its Markov structure. We will showcase the proposed methodology within the nonlinear filtering algorithm recently introduced by [Spantini et al., 2018]. The latter …
更多查看译文
AI 理解论文
溯源树
样例
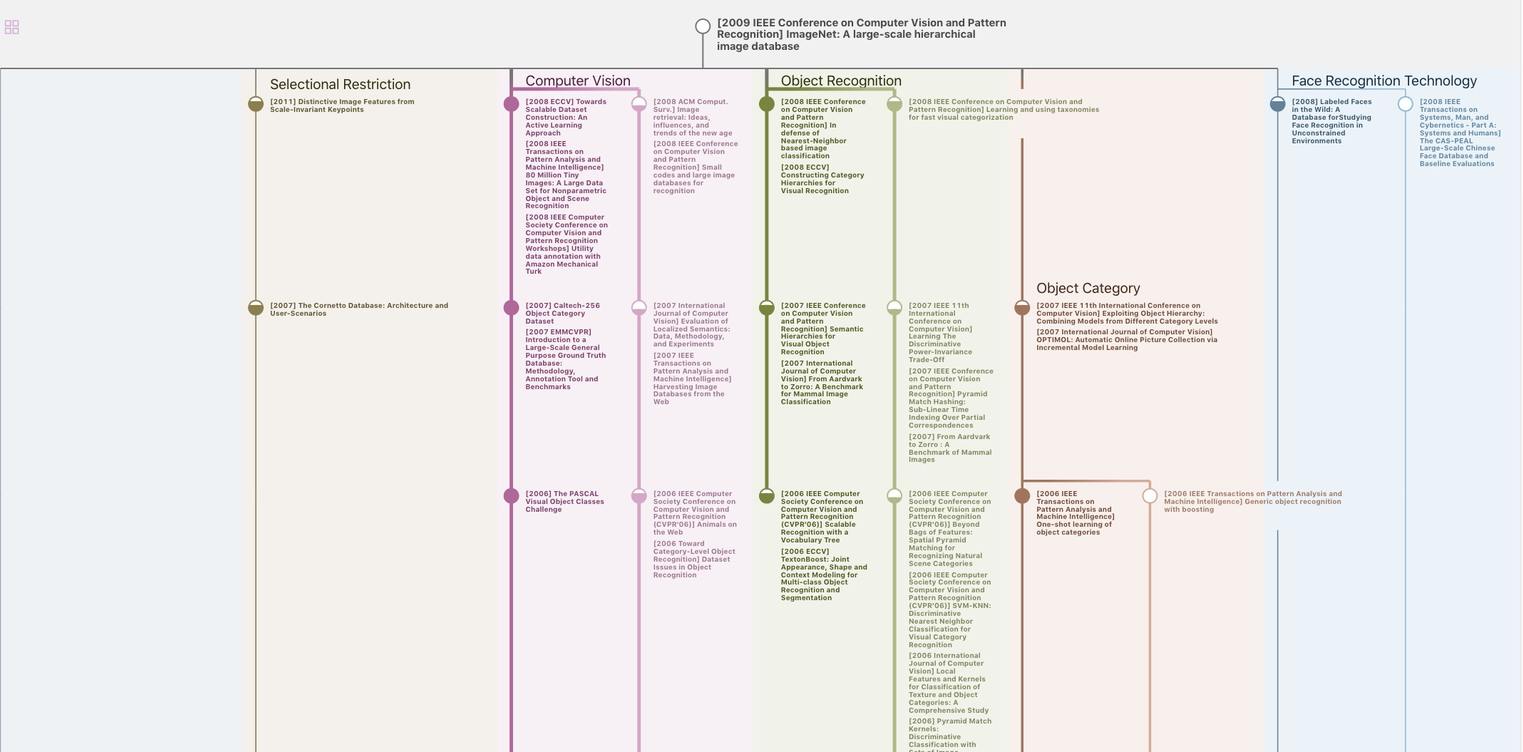
生成溯源树,研究论文发展脉络
Chat Paper
正在生成论文摘要