Diversifying Top-k Point-of-Interest Queries via Collective Social Reach
CIKM '20: The 29th ACM International Conference on Information and Knowledge Management Virtual Event Ireland October, 2020(2020)
摘要
By "checking into'' various points-of-interest (POIs), users create a rich source of location-based social network data that can be used in expressive spatio-social queries. This paper studies the use of popularity as a means to diversify results of top-k nearby POI queries. In contrast to previous work, we evaluate social diversity as a group-based, rather than individual POI, metric. Algorithmically, evaluating this set-based notion of diversity is challenging, yet we present several effective algorithms based on (integer) linear programming, a greedy framework, and r-tree distance browsing. Experiments show scalability and interactive response times for up to 100 million unique check-ins across 25000 POIs.
更多查看译文
关键词
top-k queries, socio-spatial queries, result diversification, nearest neighbours, best first search, linear programming
AI 理解论文
溯源树
样例
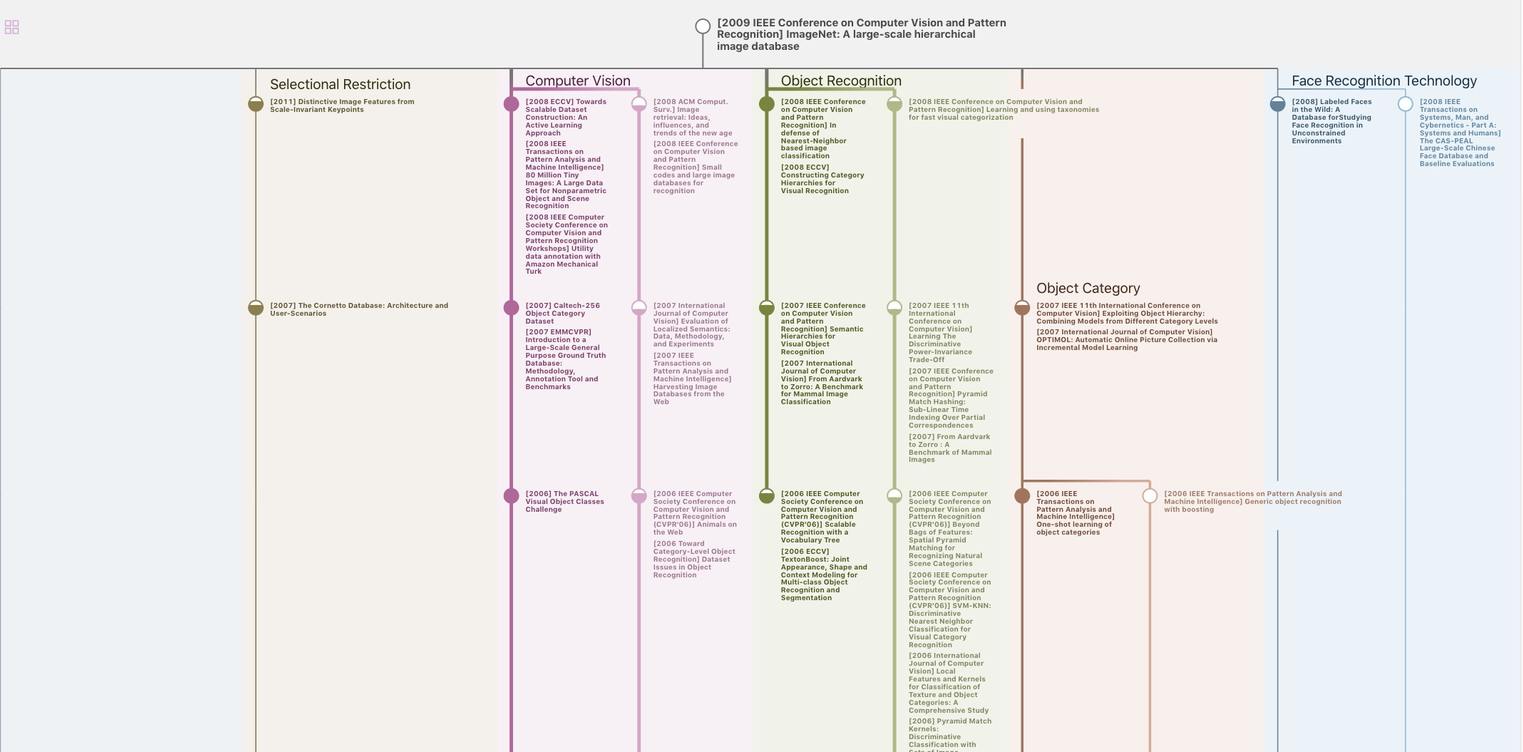
生成溯源树,研究论文发展脉络
Chat Paper
正在生成论文摘要