EPNet: Learning to Exit with Flexible Multi-Branch Network
CIKM '20: The 29th ACM International Conference on Information and Knowledge Management Virtual Event Ireland October, 2020(2020)
摘要
Dynamic inference is an emerging technique that reduces the computational cost of deep neural network under resource-constrained scenarios, such as inference on mobile devices. One way to achieve dynamic inference is to leverage multi-branch neural networks that apply different computation on input data by following different branches. Conventional research on multi-branch neural networks mainly targeted at improving the accuracy of each branch, and use manually designed rules to decide which input follows which branch of the network. Furthermore, these networks often provide a small number of exits, limiting their ability to adapt to external changes. In this paper, we investigate the problem of designing a flexible multi-branch network and early-exiting policies that can adapt to the resource consumption to individual inference request without impacting the inference accuracy. We propose a lightweight branch structure that also provides fine-grained flexibility for early-exiting and leverage Markov decision process (MDP) to automatically learn the early-exiting policies. Our proposed model, EPNet, was effective in reducing inference cost without impacting accuracy by choosing the most suitable branch exit. We also observe that EPNet achieved 3% higher accuracy with an inference budget, compared to state-of-the-art approaches.
更多查看译文
关键词
Dynamic neural network, efficient neural network, early exiting
AI 理解论文
溯源树
样例
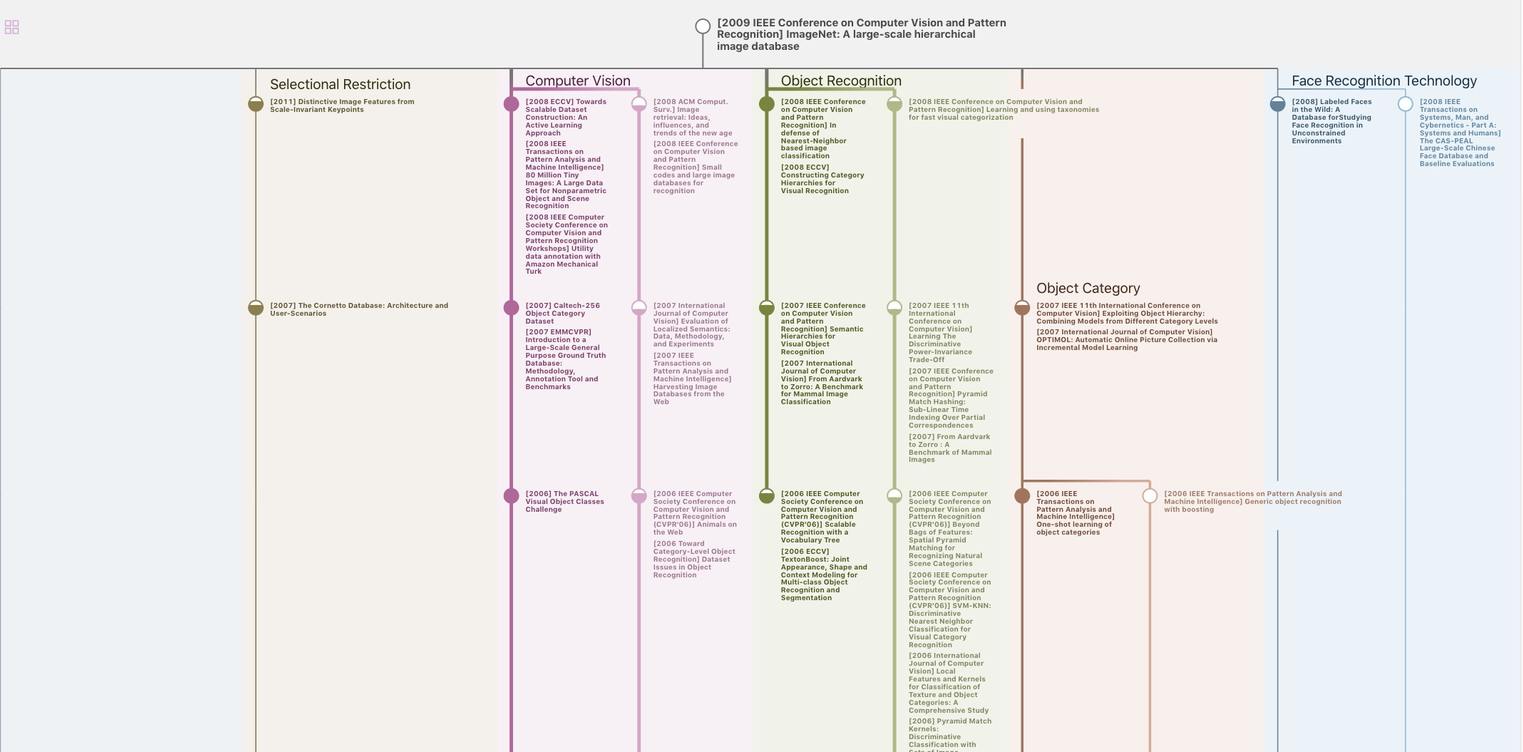
生成溯源树,研究论文发展脉络
Chat Paper
正在生成论文摘要