PCA and Kriging for the efficient exploration of consistency regions in Uncertainty Quantification
Proceedings of the Combustion Institute(2019)
摘要
For stationary power sources such as utility boilers, it is useful to dispose of parametric models able to describe their behavior in a wide range of operating conditions, to predict some Quantities of Interest (QOIs) that need to be consistent with experimental observations. The development of predictive simulation tools for large scale systems cannot rely on full-order models, as the latter would lead to prohibitive costs when coupled to sampling techniques in the model parameter space. An alternative approach consists of using a Surrogate Model (SM). As the number of QOIs is often high and many SMs need to be trained, Principal Component Analysis (PCA) can be used to encode the set of QOIs in a much smaller set of scalars, called PCA scores. A SM is then built for each PCA score rather than for each QOI. The advantage of reducing the number of variables is twofold: computational costs are reduced (less SMs need to be trained) and information is preserved (correlation among the original variables).
更多查看译文
关键词
PCA,Bound-to-Bound Data Collaboration,Uncertainty Quantification,Surrogate models
AI 理解论文
溯源树
样例
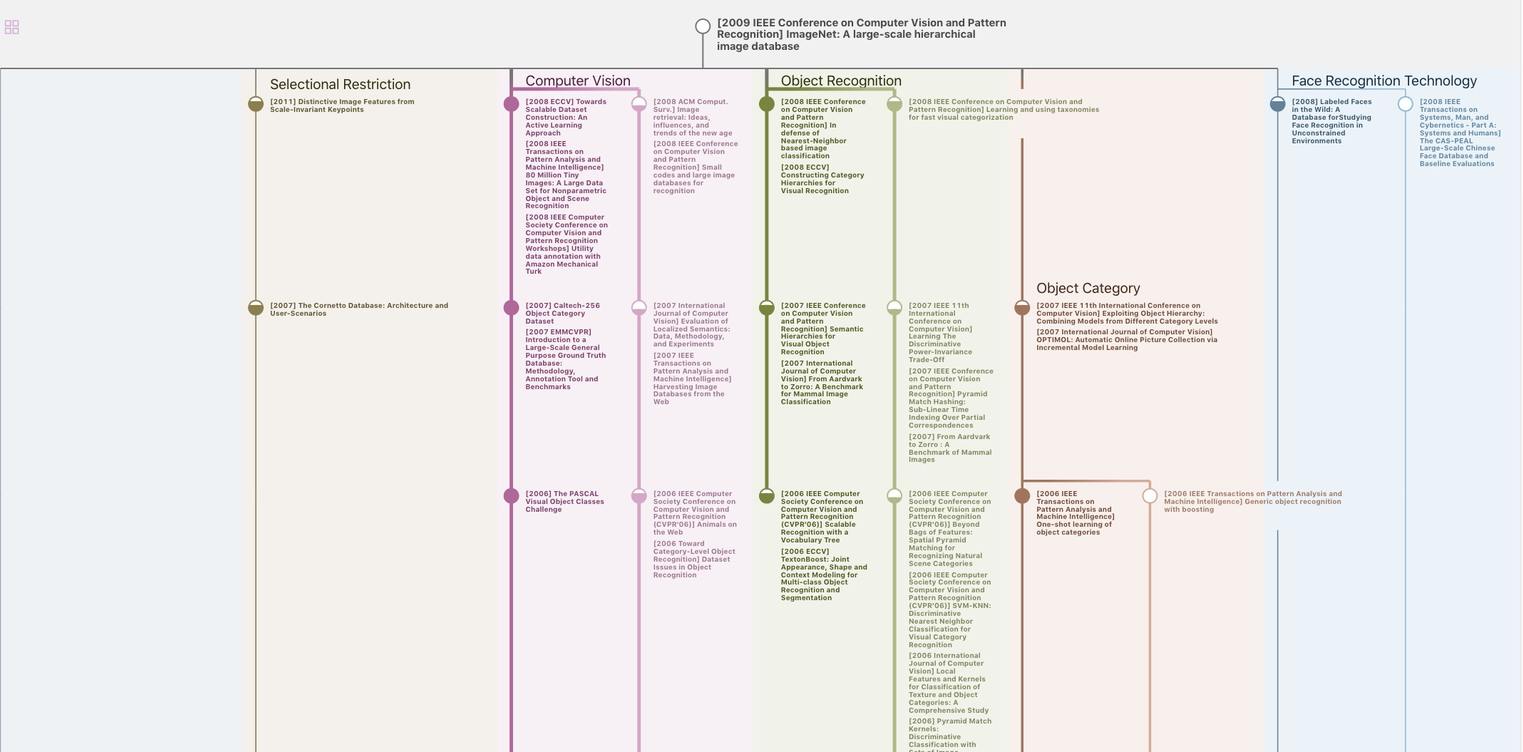
生成溯源树,研究论文发展脉络
Chat Paper
正在生成论文摘要