How to Classify, Detect, and Manage Univariate and Multivariate Outliers, With Emphasis on Pre-Registration
INTERNATIONAL REVIEW OF SOCIAL PSYCHOLOGY(2019)
摘要
Researchers often lack knowledge about how to deal with outliers when analyzing their data. Even more frequently, researchers do not pre-specify how they plan to manage outliers. In this paper we aim to improve research practices by outlining what you need to know about outliers. We start by providing a functional definition of outliers. We then lay down an appropriate nomenclature/classification of outliers. This nomenclature is used to understand what kinds of outliers can be encountered and serves as a guideline to make appropriate decisions regarding the conservation, deletion, or recoding of outliers. These decisions might impact the validity of statistical inferences as well as the reproducibility of our experiments. To be able to make informed decisions about outliers you first need proper detection tools. We remind readers why the most common outlier detection methods are problematic and recommend the use of the median absolute deviation to detect univariate outliers, and of the Mahalanobis-MCD distance to detect multivariate outliers. An R package was created that can be used to easily perform these detection tests. Finally, we promote the use of pre-registration to avoid flexibility in data analysis when handling outliers.
更多查看译文
关键词
outliers,preregistration,robust detection,Malahanobis distance,median absolute deviation,minimum covariance determinant
AI 理解论文
溯源树
样例
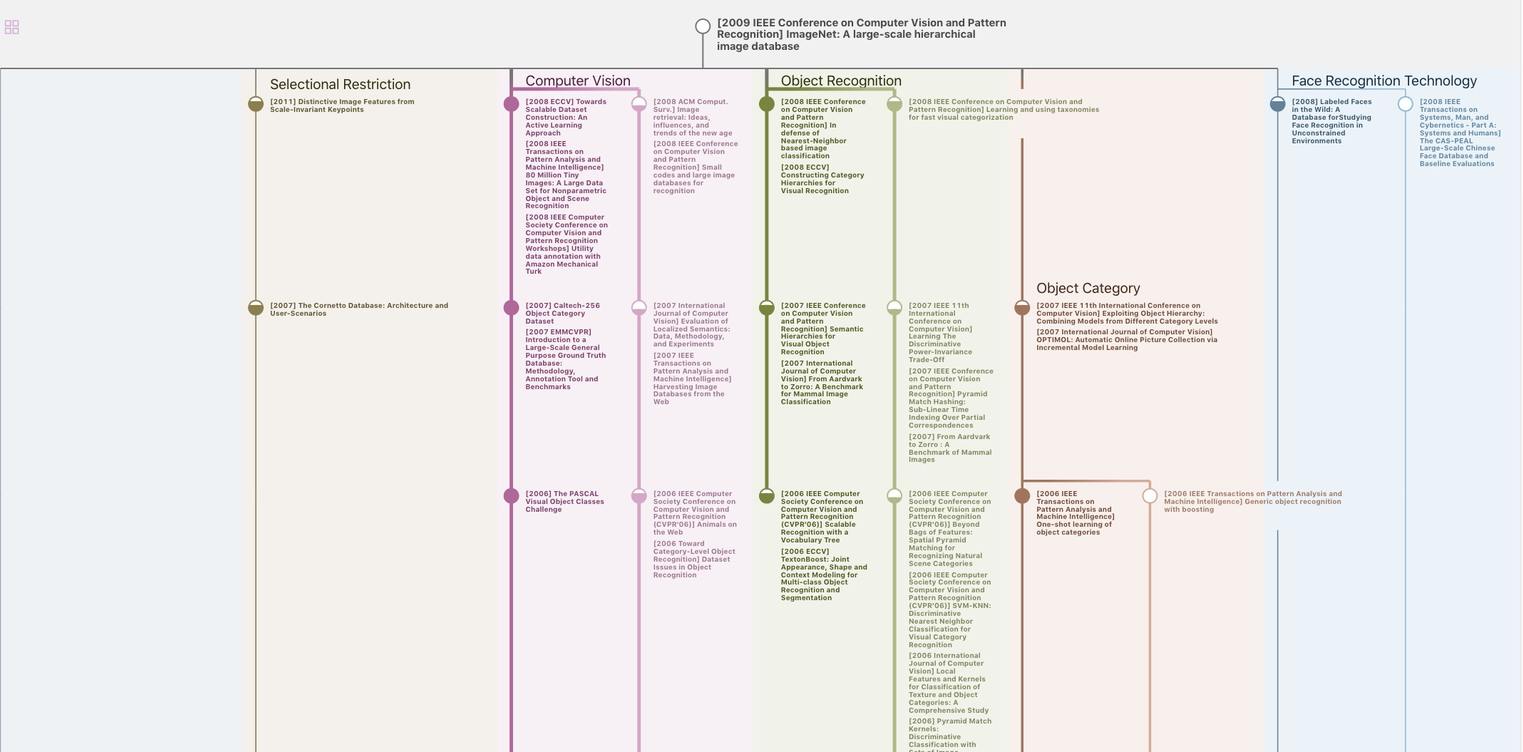
生成溯源树,研究论文发展脉络
Chat Paper
正在生成论文摘要