Text-Guided Image Inpainting
MM '20: The 28th ACM International Conference on Multimedia Seattle WA USA October, 2020(2020)
摘要
Given a partially masked image, image inpainting aims to complete the missing region and output a plausible image. Most existing image inpainting methods complete the missing region by expanding or borrowing information from the surrounding source region, which work well when the original content in the missing region is similar to the surrounding source region. Unsatisfactory results will be generated if there is no sufficient contextual information can be referenced from source region. Besides, the inpainting results should be diverse and this kind of diversity should be controllable. Based on these observations, we propose a new inpainting problem that introduces text as a kind of guidance to direct and control the inpainting process. The main difference between this problem and previous works is that we need ensure the result to be consistent with not only the source region but also the textual guidance during inpainting. By this way, we want to avoid the unreasonable completion and meanwhile make it controllable. We propose a progressively coarse-to-fine cross-modal generative network and adopt the text-image-text training schema to generate visually consistent and semantically coherent images. Extensive quantitative and qualitative experiments on two public datasets with captions demonstrate the effectiveness of our method.
更多查看译文
关键词
computer vison, image inpainting, generative models
AI 理解论文
溯源树
样例
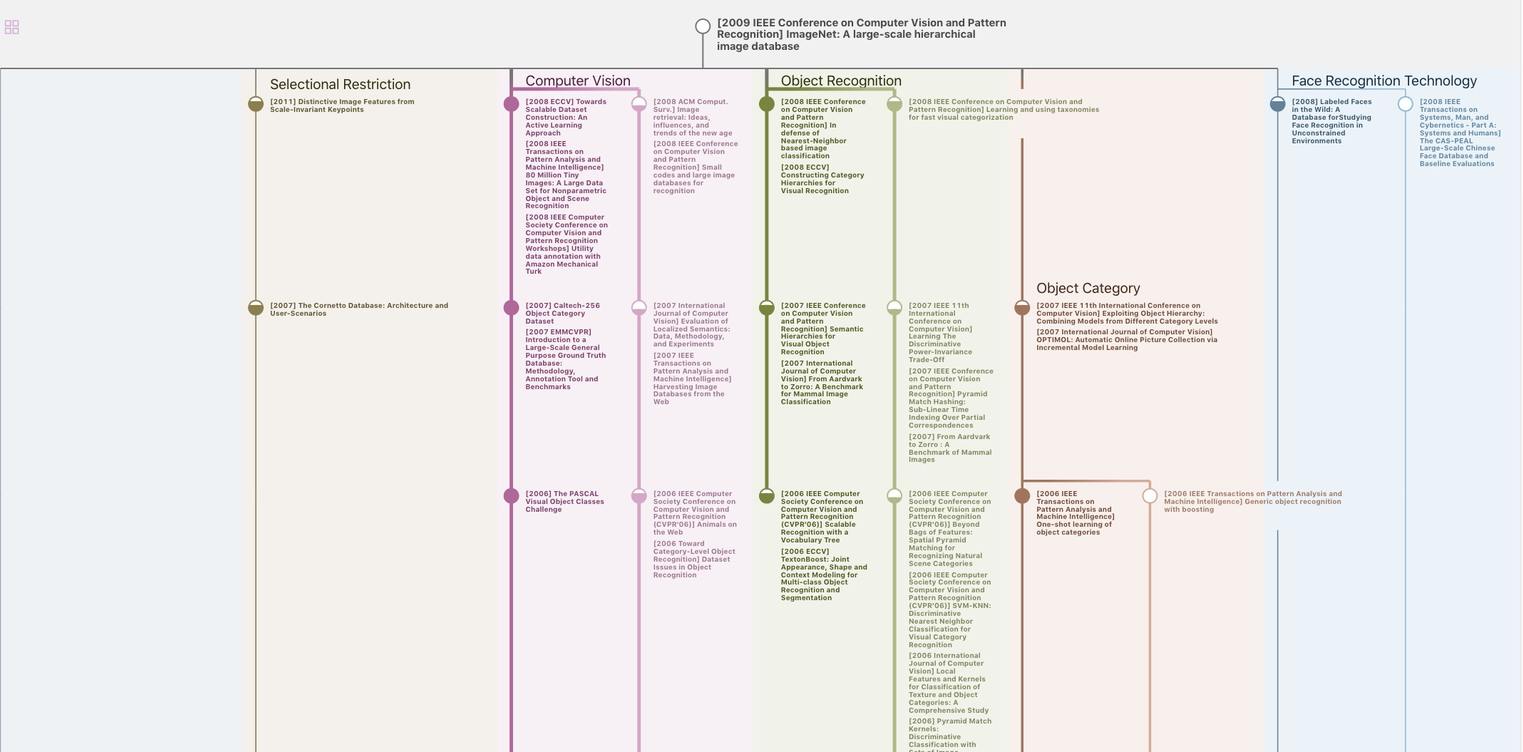
生成溯源树,研究论文发展脉络
Chat Paper
正在生成论文摘要