DATA-DRIVEN MODEL-FREE ITERATIVE LEARNING CONTROL USING REINFORCEMENT LEARNING
ASTRODYNAMICS 2018, PTS I-IV(2019)
摘要
Iterative learning control (ILC) learns to track a pre-defined maneuver with high accuracy by repetitions in practice. It can be applied to repeated scanning maneuvers with fine pointing equipment in spacecraft. An ILC learning law usually necessitates an a priori model. To remove this constraint, an off-policy reinforcement learning (RL) method is applied in the trial domain to design an ILC learning law based on data. To improve sampling efficiency and avoid dangerous learning behavior, multiplicative randomness in the learning law is used for exploration. This multiplicative randomness helps one maintain the learning process within a stable region. The algorithm developed starts with the simple model free learning that adjusts the command to the control system by the error that needs to be corrected in the previous run. The RL process uses batches of randomized ILC updates to create an improved state-action value function corresponding to a cost-to-go function. Then the cost-to-go function is used to update the ILC learning matrix. RL updates of the ILC learning matrix can be stopped at some point, and if the error starts to increase we can restart updating. This can be seen as the first step to bridge ILC and RL for data-driven learning algorithms.
更多查看译文
AI 理解论文
溯源树
样例
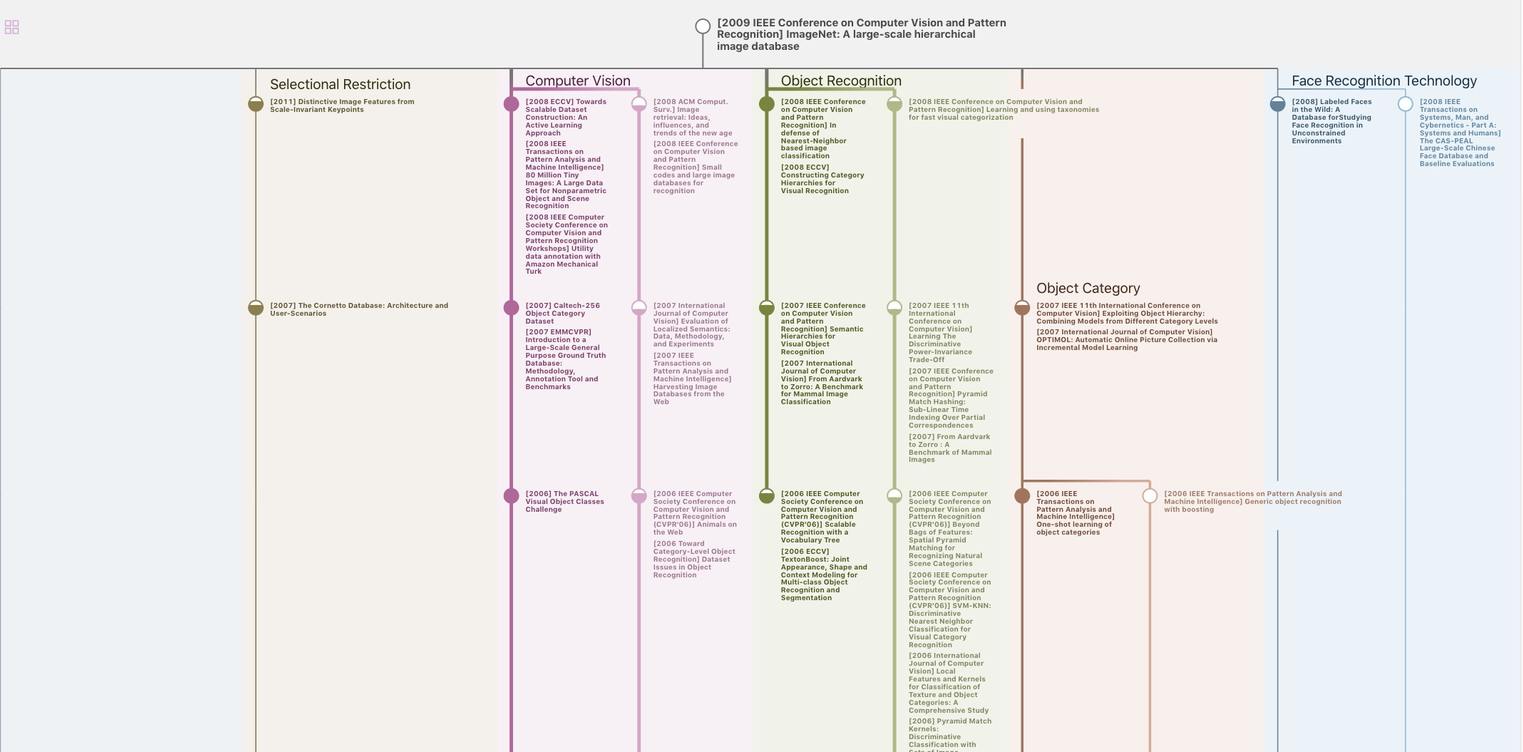
生成溯源树,研究论文发展脉络
Chat Paper
正在生成论文摘要