Uncertainty-Aware Label Refinement for Sequence Labeling
Conference on Empirical Methods in Natural Language Processing(2020)
摘要
Conditional random fields (CRF) for label decoding has become ubiquitous in sequence labeling tasks. However, the local label dependencies and inefficient Viterbi decoding have always been a problem to be solved. In this work, we introduce a novel two-stage label decoding framework to model long-term label dependencies, while being much more computationally efficient. A base model first predicts draft labels, and then a novel two-stream self-attention model makes refinements on these draft predictions based on long-range label dependencies, which can achieve parallel decoding for a faster prediction. In addition, in order to mitigate the side effects of incorrect draft labels, Bayesian neural networks are used to indicate the labels with a high probability of being wrong, which can greatly assist in preventing error propagation. The experimental results on three sequence labeling benchmarks demonstrated that the proposed method not only outperformed the CRF-based methods but also greatly accelerated the inference process.
更多查看译文
关键词
sequence,uncertainty-aware
AI 理解论文
溯源树
样例
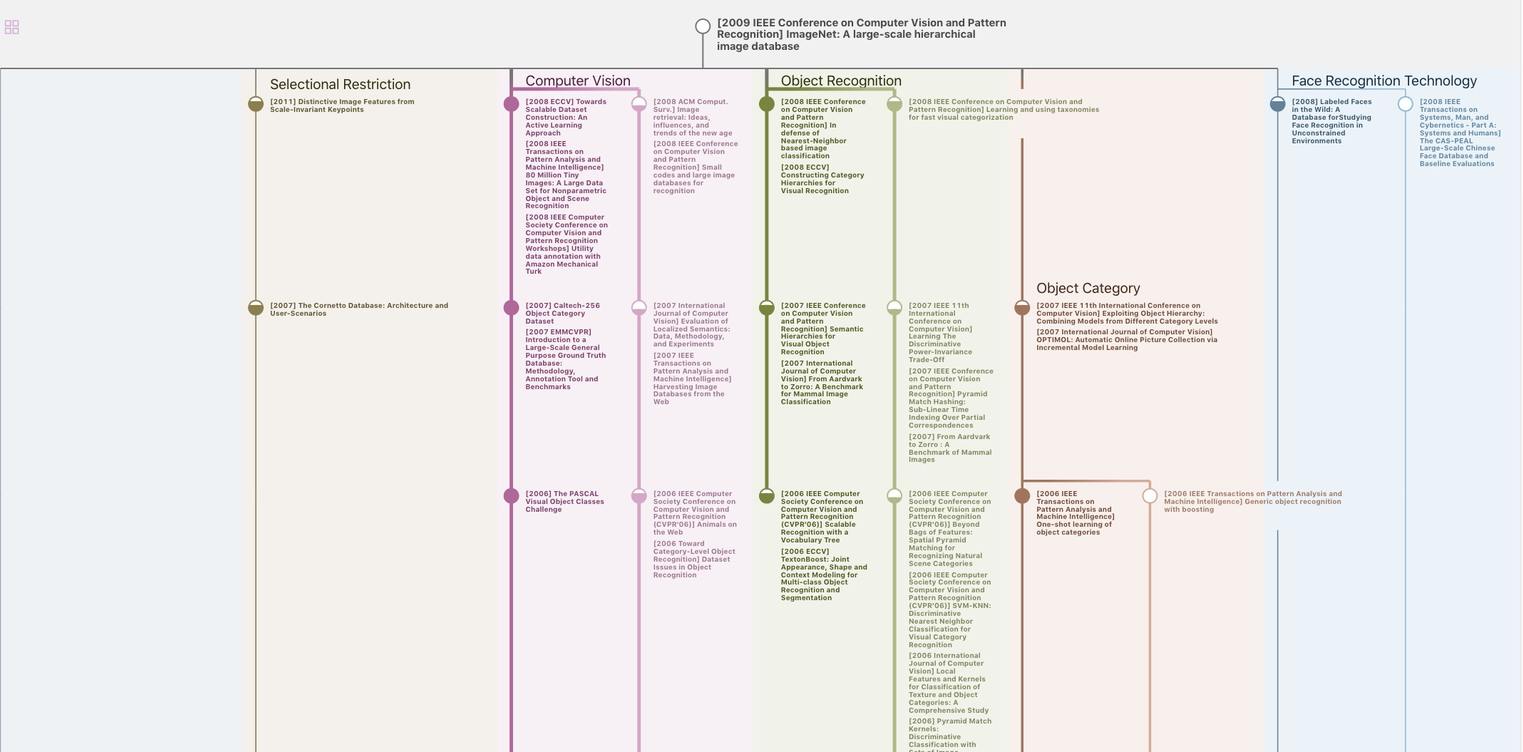
生成溯源树,研究论文发展脉络
Chat Paper
正在生成论文摘要